Multispectral Compressive Imaging Strategies Using Fabry–Pérot Filtered Sensors
IEEE Transactions on Computational Imaging(2018)
摘要
In this paper, we introduce two novel acquisition schemes for multispectral compressive imaging. Unlike most existing methods, the proposed computational imaging techniques do not include any dispersive element, as they use a dedicated sensor that integrates narrowband Fabry-Perot spectral filters at the pixel level. The first scheme leverages joint inpainting and super-resolution to fill in those voxels that are missing due to the device's limited pixel count. The second scheme introduces spatial random convolutions in link with the framework of compressive sensing, but is more complex and may be limited by diffraction. In both cases, we solve the associated inverse problems by using the same signal prior and recovery algorithm. Specifically, we propose a redundant, analysis-sparse signal prior in a convex formulation. We validate our schemes through numerical simulation in different realistic setups. Moreover, we highlight some practical guidelines and discuss complexity tradeoffs to integrate these schemes into actual computational imaging systems. Our conclusion is that the second technique performs best when targeting high compression levels if embodied in a properly designed and calibrated setup. Otherwise the first, simpler technique should be favored.
更多查看译文
关键词
Multispectral imaging,compressed sensing,spectral filters,Fabry-Perot,random convolution,generalized inpainting
AI 理解论文
溯源树
样例
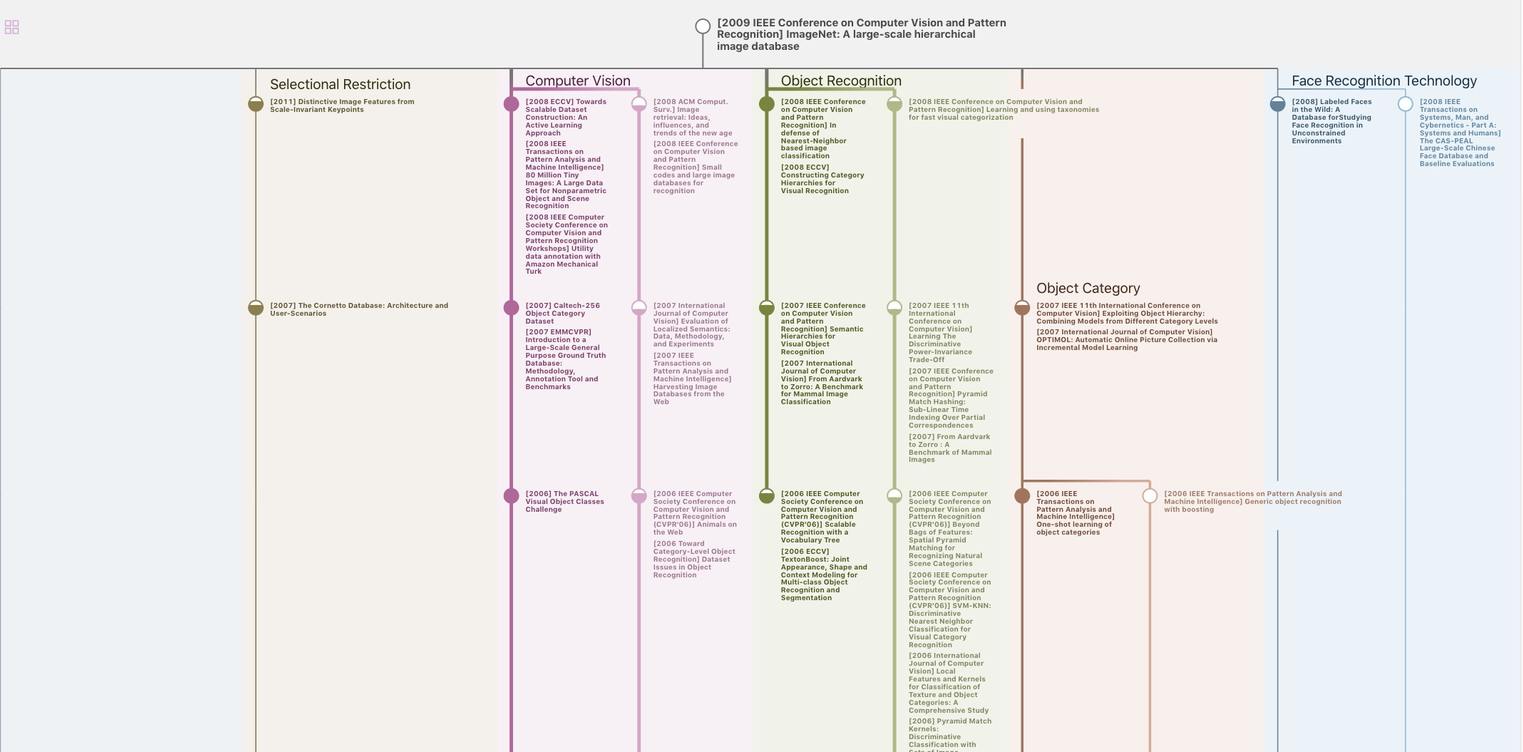
生成溯源树,研究论文发展脉络
数据免责声明
页面数据均来自互联网公开来源、合作出版商和通过AI技术自动分析结果,我们不对页面数据的有效性、准确性、正确性、可靠性、完整性和及时性做出任何承诺和保证。若有疑问,可以通过电子邮件方式联系我们:report@aminer.cn