Dynamic Range Expansion Using Cumulative Histogram Learning for High Dynamic Range Image Generation.
IEEE Access(2020)
摘要
In modern digital photographs, most images have low dynamic range (LDR) formats, which means that the range of light intensities from the darkest to the brightest is much lower than the range that can be perceived by the human eye. Therefore, to visualize images as naturally as possible on devices that display them in high dynamic range (HDR) format, the LDR images need to be converted into HDR images. The aim of this study was to develop an adaptive inverse tone mapping operator (iTMO) that can convert a single LDR image into a realistic HDR image based on artificial neural networks. In contrast to conventional iTMO algorithms, our technique was developed by learning the complicated relationship between various LDR-HDR pair images, which enabled nearly ground-truth HDR images to be generated from various types of LDR images. The novel learning technique is called cumulative histogram-based learning and color difference learning. The superior performance of our technique over conventional methods was assessed through objective evaluations of various types of LDR and HDR images.
更多查看译文
关键词
High dynamic range,inverse tone mapping operator,cumulative histogram,color difference,convolutional neural network
AI 理解论文
溯源树
样例
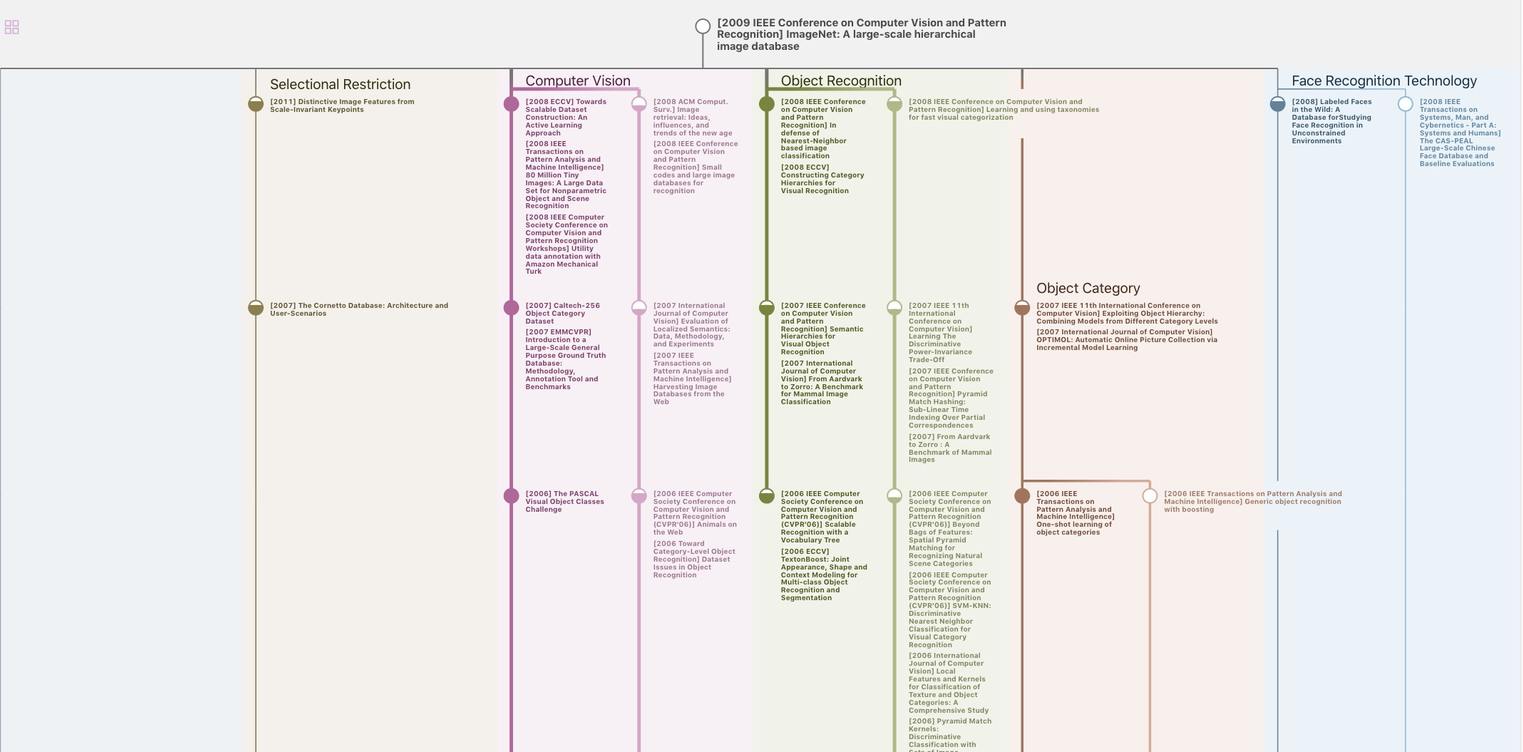
生成溯源树,研究论文发展脉络
数据免责声明
页面数据均来自互联网公开来源、合作出版商和通过AI技术自动分析结果,我们不对页面数据的有效性、准确性、正确性、可靠性、完整性和及时性做出任何承诺和保证。若有疑问,可以通过电子邮件方式联系我们:report@aminer.cn