Efficient Conditional Pre-training for Transfer Learning
2022 IEEE/CVF Conference on Computer Vision and Pattern Recognition Workshops (CVPRW)(2022)
摘要
Almost all the state-of-the-art neural networks for computer vision tasks are trained by (1) pre-training on a large-scale dataset and (2) finetuning on the target dataset. This strategy helps reduce dependence on the target dataset and improves convergence rate and generalization on the target task. Although pre-training on large-scale datasets is very useful for new methods or models, its foremost disadvantage is high training cost. To address this, we propose efficient filtering methods to select relevant subsets from the pre-training dataset. Additionally, we discover that lowering image resolutions in the pre-training step offers a great trade-off between cost and performance. We validate our techniques by pre-training on ImageNet in both the unsupervised and supervised settings and finetuning on a diverse collection of target datasets and tasks. Our proposed methods drastically reduce pre-training cost and provide strong performance boosts. Finally, we improve the current standard of ImageNet pre-training by 1-3% by tuning available models on our subsets and pre-training on a dataset filtered from a larger scale dataset.
更多查看译文
关键词
large-scale dataset,target dataset,target task,high training cost,pre-training dataset,pre-training step,target datasets,pre-training cost,ImageNet pre-training,larger scale dataset,efficient conditional pre-training,unsupervised settings
AI 理解论文
溯源树
样例
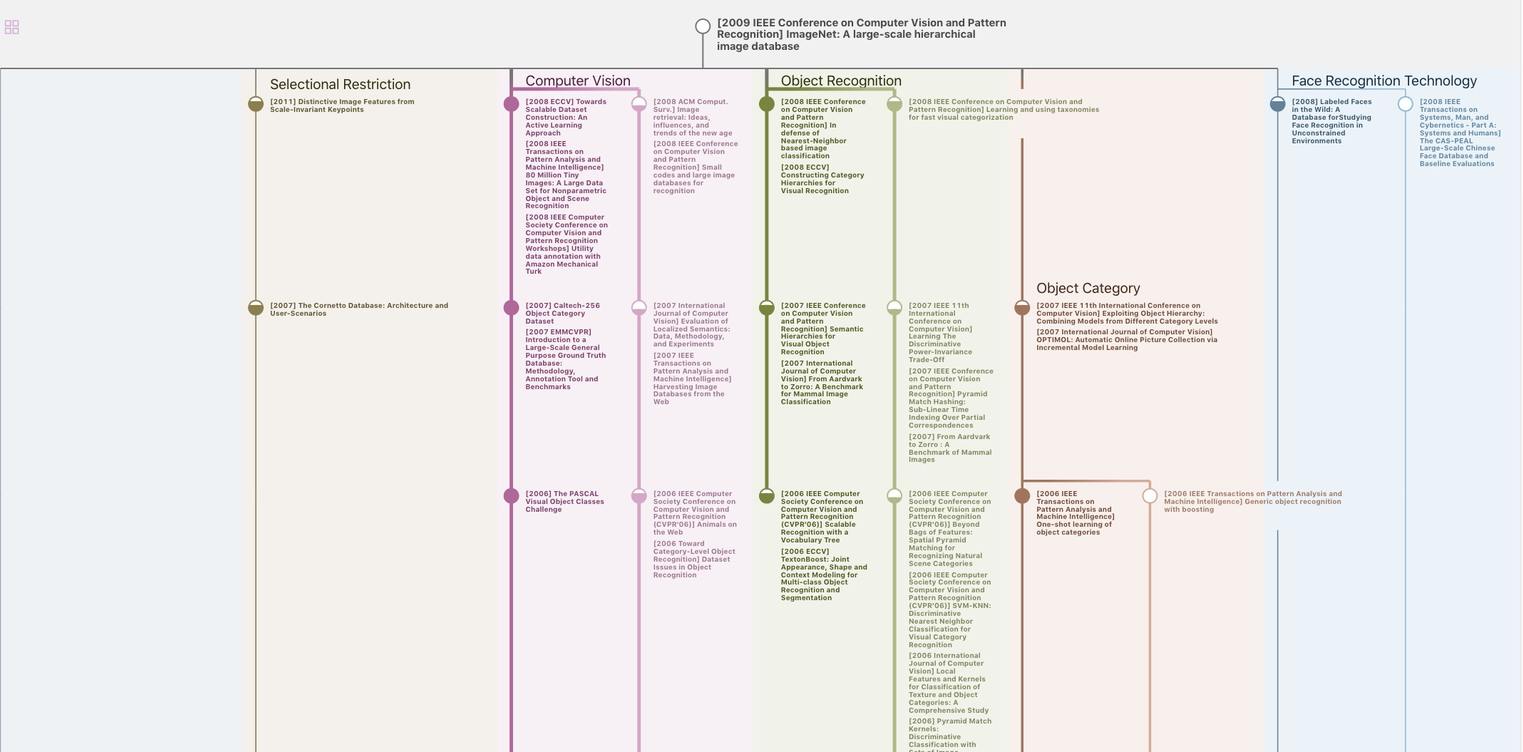
生成溯源树,研究论文发展脉络
数据免责声明
页面数据均来自互联网公开来源、合作出版商和通过AI技术自动分析结果,我们不对页面数据的有效性、准确性、正确性、可靠性、完整性和及时性做出任何承诺和保证。若有疑问,可以通过电子邮件方式联系我们:report@aminer.cn