Idarts: Improving DARTS by Node Normalization and Decorrelation Discretization
IEEE TRANSACTIONS ON NEURAL NETWORKS AND LEARNING SYSTEMS(2023)
摘要
Differentiable ARchiTecture Search (DARTS) uses a continuous relaxation of network representation and dramatically accelerates Neural Architecture Search (NAS) by almost thousands of times in GPU-day. However, the searching process of DARTS is unstable, which suffers severe degradation when training epochs become large, thus limiting its application. In this article, we claim that this degradation issue is caused by the imbalanced norms between different nodes and the highly correlated outputs from various operations. We then propose an improved version of DARTS, namely iDARTS, to deal with the two problems. In the training phase, it introduces node normalization to maintain the norm balance. In the discretization phase, the continuous architecture is approximated based on the similarity between the outputs of the node and the decorrelated operations rather than the values of the architecture parameters. Extensive evaluation is conducted on CIFAR-10 and ImageNet, and the error rates of 2.25% and 24.7% are reported within 0.2 and 1.9 GPU-day for architecture search, respectively, which shows its effectiveness. Additional analysis also reveals that iDARTS has the advantage in robustness and generalization over other DARTS-based counterparts.
更多查看译文
关键词
Computer architecture,Degradation,Training,Microprocessors,Optimization,Computational efficiency,Neural networks,AutoML,deep learning,Differentiable ARchiTecture Search (DARTS),Neural Architecture Search (NAS)
AI 理解论文
溯源树
样例
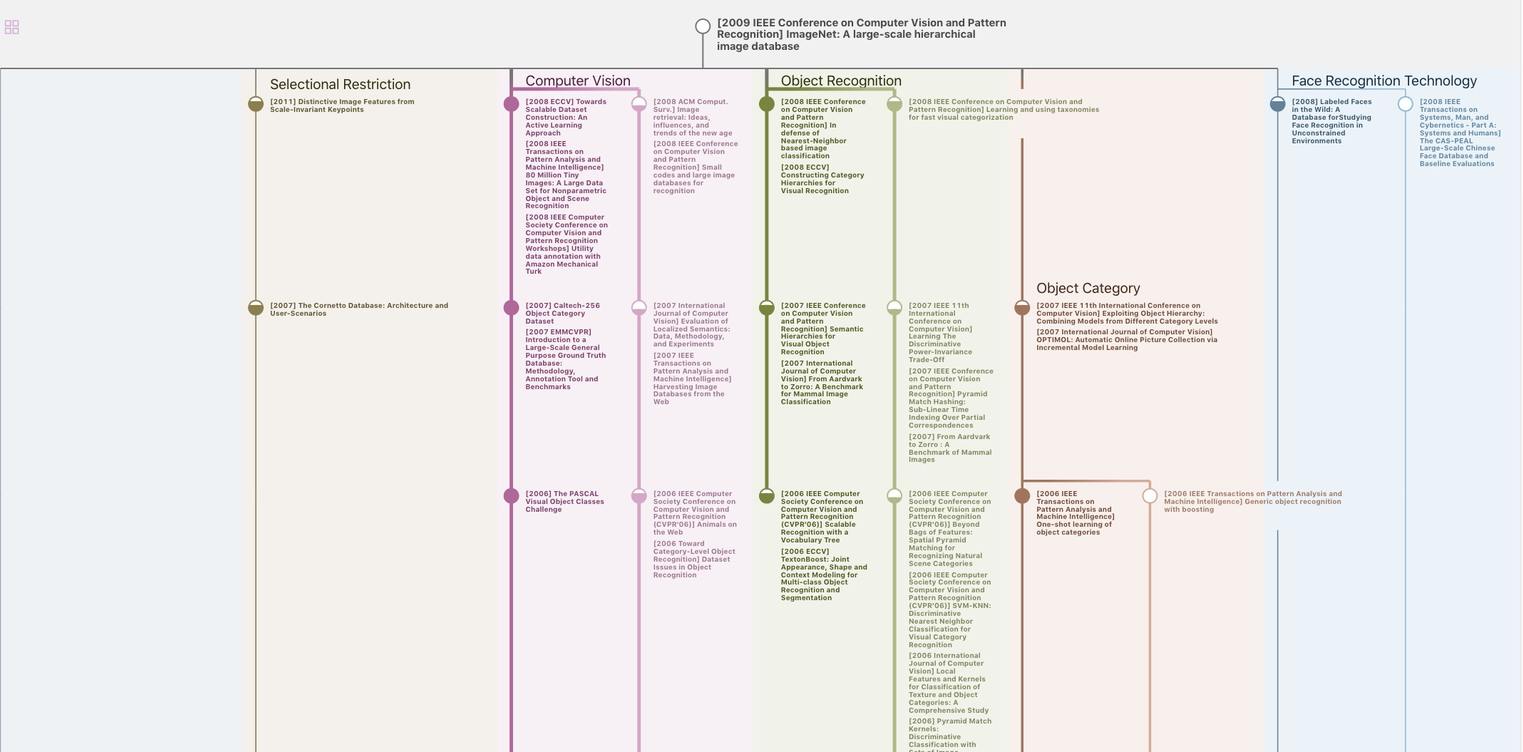
生成溯源树,研究论文发展脉络
数据免责声明
页面数据均来自互联网公开来源、合作出版商和通过AI技术自动分析结果,我们不对页面数据的有效性、准确性、正确性、可靠性、完整性和及时性做出任何承诺和保证。若有疑问,可以通过电子邮件方式联系我们:report@aminer.cn