Weak Correlations in Health Services Research: Weak Relationships or Common Error?
HEALTH SERVICES RESEARCH(2022)
摘要
Objective To examine whether the correlation between a provider's effect on one population of patients and the same provider's effect on another population is underestimated if the effects for each population are estimated separately as opposed to being jointly modeled as random effects, and to characterize how the impact of the estimation procedure varies with sample size. Data sources Medicare claims and enrollment data on emergency department (ED) visits, including patient characteristics, the patient's hospitalization status, and identification of the doctor responsible for the decision to hospitalize the patient. Study design We used a three-pronged investigation consisting of analytical derivation, simulation experiments, and analysis of administrative data to demonstrate the fallibility of stratified estimation. Under each investigation method, results are compared between the joint modeling approach to those based on stratified analyses. Data collection/extraction methods We used data on ED visits from administrative claims from traditional (fee-for-service) Medicare from January 2012 through September 2015. Principal findings The simulation analysis demonstrates that the joint modeling approach is generally close to unbiased, whereas the stratified approach can be severely biased in small samples, a consequence of joint modeling benefitting from bivariate shrinkage and the stratified approach being compromised by measurement error. In the administrative data analyses, the estimated correlation of doctor admission tendencies between female and male patients was estimated to be 0.98 under the joint model but only 0.38 using stratified estimation. The analogous correlations for White and non-White patients are 0.99 and 0.28 and for Medicaid dual-eligible and non-dual-eligible patients are 0.99 and 0.31, respectively. These results are consistent with the analytical derivations. Conclusions Joint modeling targets the parameter of primary interest. In the case of population correlations, it yields estimates that are substantially less biased and higher in magnitude than naive estimators that post-process the estimates obtained from stratified models.
更多查看译文
关键词
attenuation,bivariate random effects,bivariate shrinkage,hierarchical model,measurement error
AI 理解论文
溯源树
样例
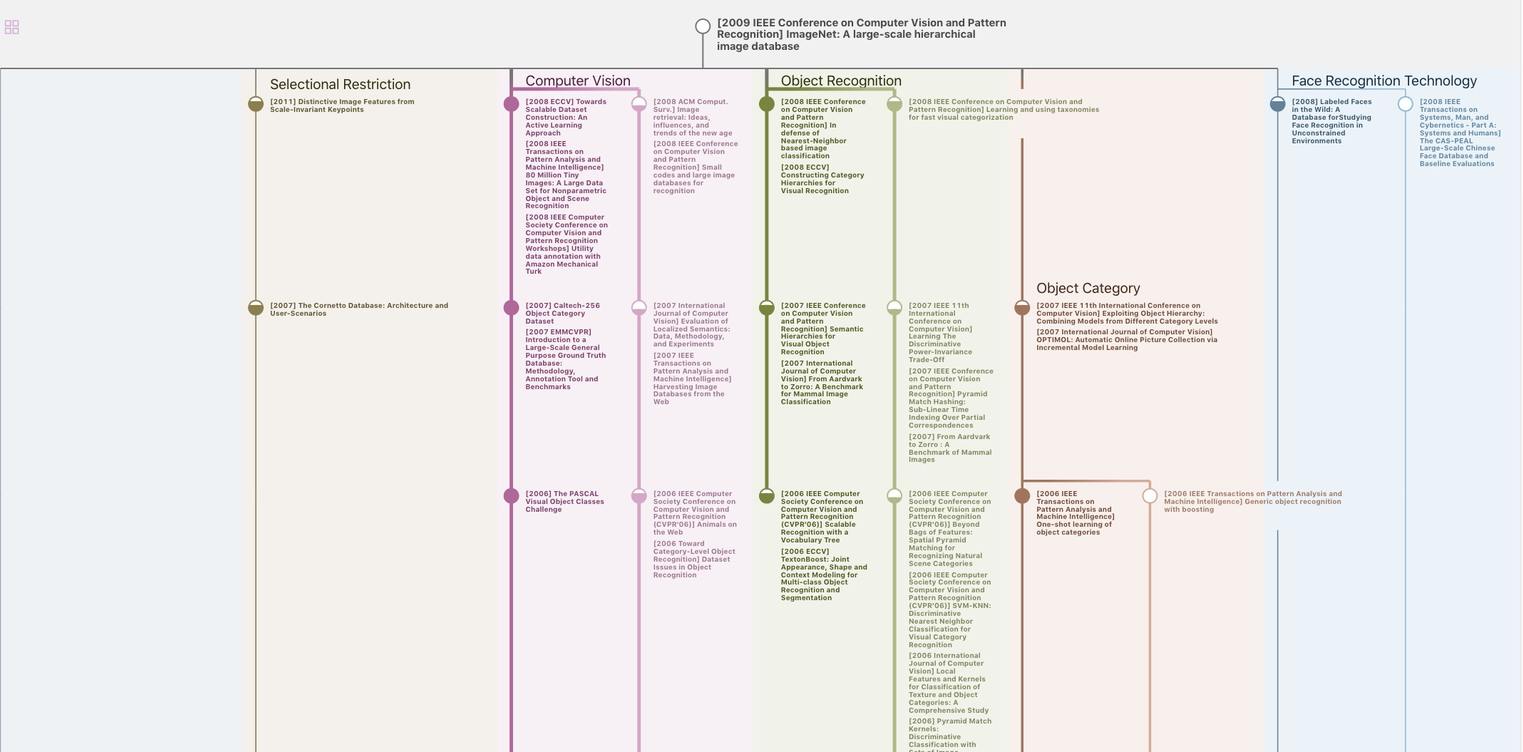
生成溯源树,研究论文发展脉络
数据免责声明
页面数据均来自互联网公开来源、合作出版商和通过AI技术自动分析结果,我们不对页面数据的有效性、准确性、正确性、可靠性、完整性和及时性做出任何承诺和保证。若有疑问,可以通过电子邮件方式联系我们:report@aminer.cn