Off-Policy Correction for Deep Deterministic Policy Gradient Algorithms Via Batch Prioritized Experience Replay.
2021 IEEE 33rd International Conference on Tools with Artificial Intelligence (ICTAI)(2021)
摘要
The experience replay mechanism allows agents to use the experiences multiple times. In prior works, the sampling probability of the transitions was adjusted according to their importance. Reassigning sampling probabilities for every transition in the replay buffer after each iteration is highly inefficient. Therefore, experience replay prioritization algorithms recalculate the significance of a transition when the corresponding transition is sampled to gain computational efficiency. However, the importance level of the transitions changes dynamically as the policy and the value function of the agent are updated. In addition, experience replay stores the transitions are generated by the previous policies of the agent that may significantly deviate from the most recent policy of the agent. Higher deviation from the most recent policy of the agent leads to more off-policy updates, which is detrimental for the agent. In this paper, we develop a novel algorithm, Batch Prioritizing Experience Replay via KL Divergence (KLPER), which prioritizes batch of transitions rather than directly prioritizing each transition. Moreover, to reduce the off-policyness of the updates, our algorithm selects one batch among a certain number of batches and forces the agent to learn through the batch that is most likely generated by the most recent policy of the agent. We combine our algorithm with Deep Deterministic Policy Gradient and Twin Delayed Deep Deterministic Policy Gradient and evaluate it on various continuous control tasks. KLPER provides promising improvements for deep deterministic continuous control algorithms in terms of sample efficiency, final performance, and stability of the policy during the training.
更多查看译文
关键词
deep reinforcement learning,experience replay,prioritized sampling,continuous control,off-policy learning
AI 理解论文
溯源树
样例
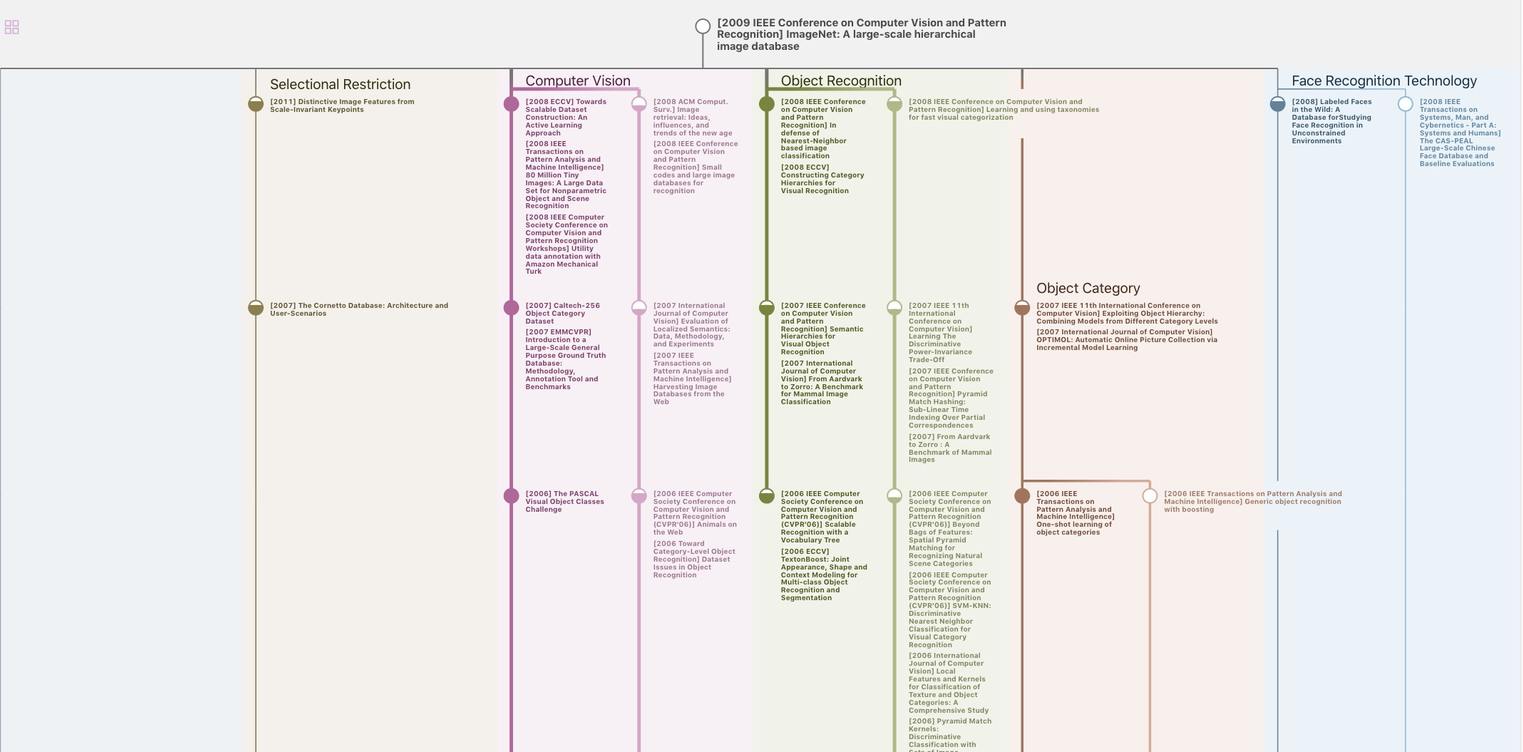
生成溯源树,研究论文发展脉络
数据免责声明
页面数据均来自互联网公开来源、合作出版商和通过AI技术自动分析结果,我们不对页面数据的有效性、准确性、正确性、可靠性、完整性和及时性做出任何承诺和保证。若有疑问,可以通过电子邮件方式联系我们:report@aminer.cn