Hierarchical Graph Attention Network for Few-shot Visual-Semantic Learning.
ICCV(2021)
摘要
Deep learning has made tremendous success in computer vision, natural language processing and even visual-semantic learning, which requires a huge amount of labeled training data. Nevertheless, the goal of human-level intelligence is to enable a model to quickly obtain an in-depth understanding given a small number of samples, especially with heterogeneity in the multi-modal scenarios such as visual question answering and image captioning. In this paper, we study the few-shot visual-semantic learning and present the Hierarchical Graph ATtention network (HGAT). This two-stage network models the intra- and inter-modal relationships with limited image-text samples. The main contributions of HGAT can be summarized as follows: 1) it sheds light on tackling few-shot multi-modal learning problems, which focuses primarily, but not exclusively on visual and semantic modalities, through better exploitation of the intra-relationship of each modality and an attention-based co-learning framework between modalities using a hierarchical graph-based architecture; 2) it achieves superior performance on both visual question answering and image captioning in the few-shot setting; 3) it can be easily extended to the semi-supervised setting where image-text samples are partially unlabeled. We show via extensive experiments that HGAT delivers state-of-the-art performance on three widely-used benchmarks of two visual-semantic learning tasks.
更多查看译文
关键词
Vision + language,Transfer/Low-shot/Semi/Unsupervised Learning,Visual reasoning and logical representation
AI 理解论文
溯源树
样例
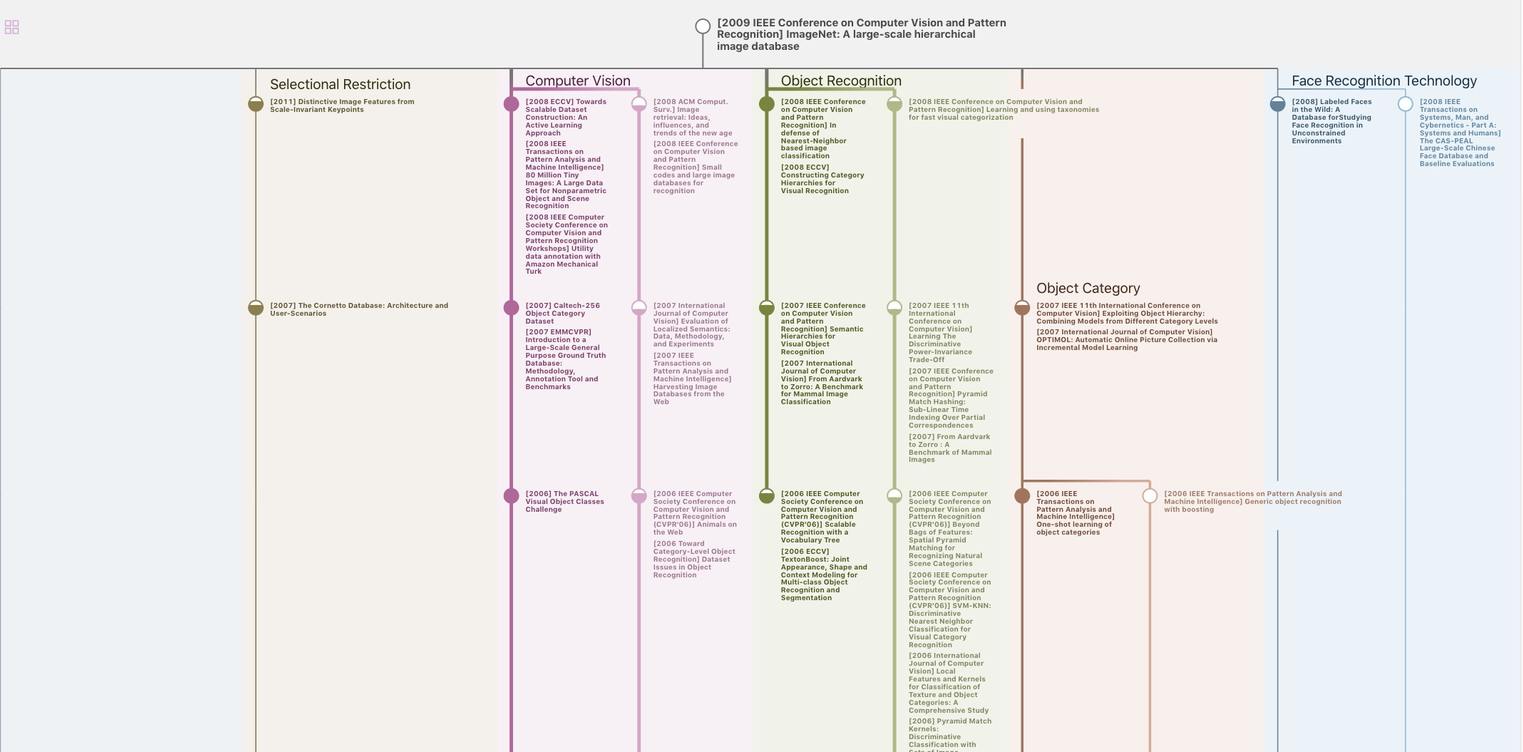
生成溯源树,研究论文发展脉络
数据免责声明
页面数据均来自互联网公开来源、合作出版商和通过AI技术自动分析结果,我们不对页面数据的有效性、准确性、正确性、可靠性、完整性和及时性做出任何承诺和保证。若有疑问,可以通过电子邮件方式联系我们:report@aminer.cn