Machine Learning of Genomic Features in Organotropic Metastases Stratifies Progression Risk of Primary Tumors
Nature Communications(2021)
摘要
Metastatic cancer is associated with poor patient prognosis but its spatiotemporal behavior remains unpredictable at early stage. Here we develop MetaNet, a computational framework that integrates clinical and sequencing data from 32,176 primary and metastatic cancer cases, to assess metastatic risks of primary tumors. MetaNet achieves high accuracy in distinguishing the metastasis from the primary in breast and prostate cancers. From the prediction, we identify Metastasis-Featuring Primary (MFP) tumors, a subset of primary tumors with genomic features enriched in metastasis and demonstrate their higher metastatic risk and shorter disease-free survival. In addition, we identify genomic alterations associated with organ-specific metastases and employ them to stratify patients into various risk groups with propensities toward different metastatic organs. This organotropic stratification method achieves better prognostic value than the standard histological grading system in prostate cancer, especially in the identification of Bone-MFP and Liver-MFP subtypes, with potential in informing organ-specific examinations in follow-ups.
更多查看译文
关键词
Data integration,Gene regulatory networks,Machine learning,Science,Humanities and Social Sciences,multidisciplinary
AI 理解论文
溯源树
样例
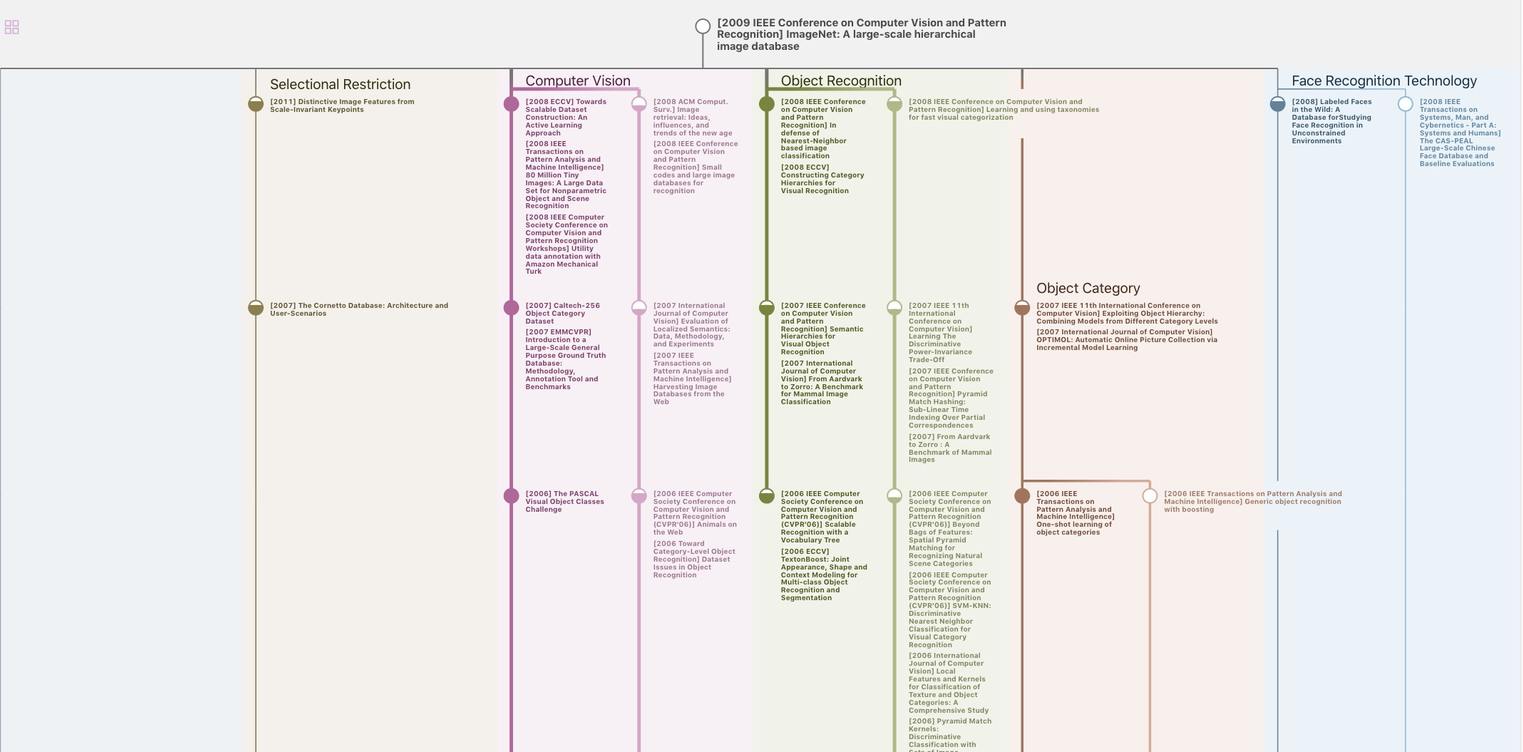
生成溯源树,研究论文发展脉络
数据免责声明
页面数据均来自互联网公开来源、合作出版商和通过AI技术自动分析结果,我们不对页面数据的有效性、准确性、正确性、可靠性、完整性和及时性做出任何承诺和保证。若有疑问,可以通过电子邮件方式联系我们:report@aminer.cn