Learning to Perceive and Manipulate Diverse Food Materials Through Interaction
semanticscholar(2021)
摘要
The home kitchen environment presents many challenges for an autonomous cooking robot, such as the deformability of food items, the wide range of material properties of food, and the complex interaction dynamics involved in food manipulation tasks. Material properties are important when interacting with nonrigid body objects, because they have a large influence on how the skills should be performed. To successfully execute skills such as food cutting, the robot should be able to infer material properties of and adapt its behaviors to different food materials. In this thesis we discuss experimental approaches to robot learning in the context of food manipulation skills. First, we present an approach to encode food item material property information through unsupervised robotic interactions with a variety of food classes, and show how these learned embeddings can be used for material property-related classification and regression tasks. Second, we focus on enabling the robot to learn a range of food cutting behaviors from human demonstrations and optimize these behaviors using reinforcement learning, specifically looking at different ways of defining rewards for robotic cutting tasks. Our results indicate that the robot can autonomously learn about food item material properties through interaction, and also that it can successfully learn and optimize its cutting behaviors for different food types using a reinforcement learning framework.
更多查看译文
AI 理解论文
溯源树
样例
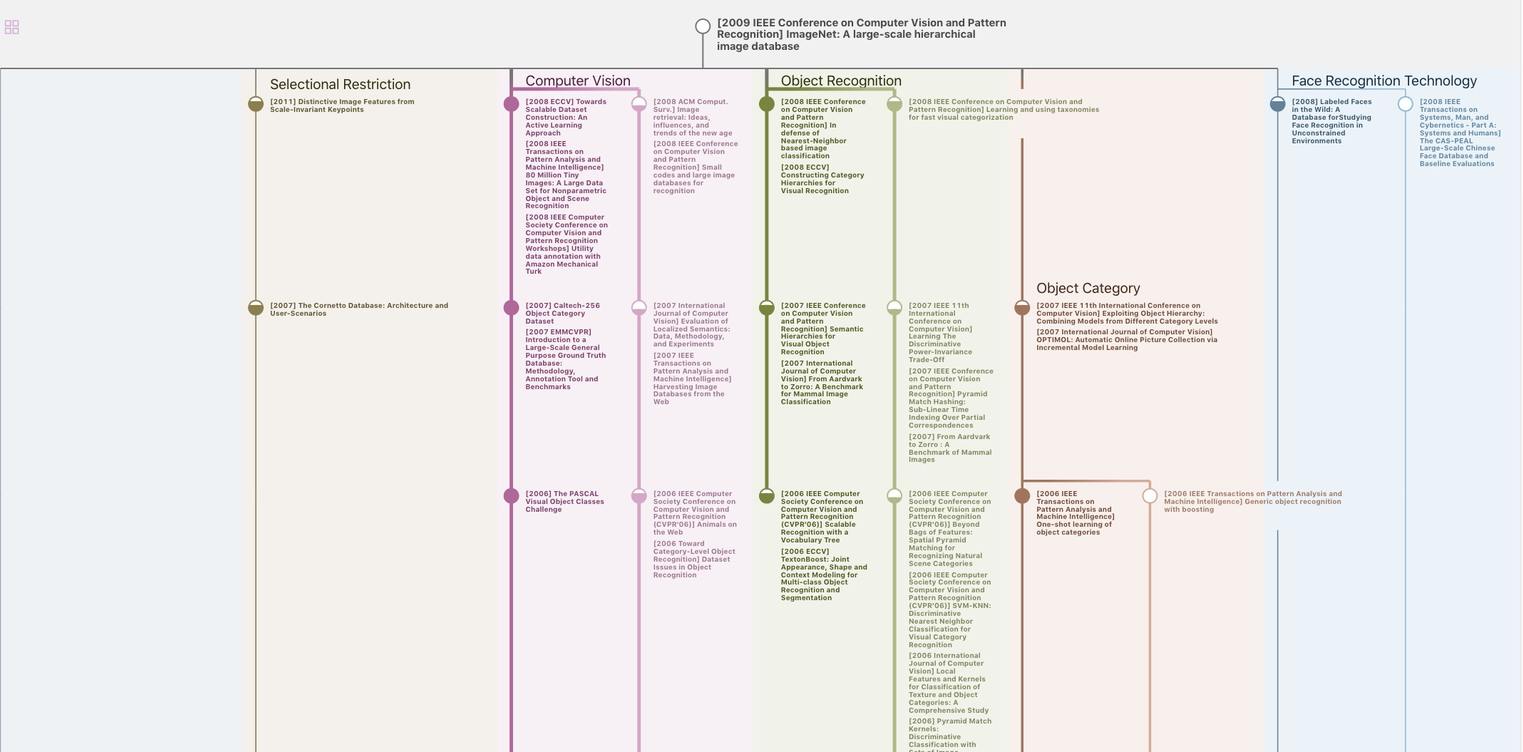
生成溯源树,研究论文发展脉络
数据免责声明
页面数据均来自互联网公开来源、合作出版商和通过AI技术自动分析结果,我们不对页面数据的有效性、准确性、正确性、可靠性、完整性和及时性做出任何承诺和保证。若有疑问,可以通过电子邮件方式联系我们:report@aminer.cn