Exploiting 3D Hand Pose Estimation in Deep Learning-Based Sign Language Recognition from RGB Videos
Computer Vision – ECCV 2020 Workshops Lecture Notes in Computer Science(2020)
摘要
In this paper, we investigate the benefit of 3D hand skeletal information to the task of sign language (SL) recognition from RGB videos, within a state-of-the-art, multiple-stream, deep-learning recognition system. As most SL datasets are available in traditional RGB-only video lacking depth information, we propose to infer 3D coordinates of the hand joints from RGB data via a powerful architecture that has been primarily introduced in the literature for the task of 3D human pose estimation. We then fuse these estimates with additional SL informative streams, namely 2D skeletal data, as well as convolutional neural network-based handand mouth-region representations, and employ an attention-based encoder-decoder for recognition. We evaluate our proposed approach on a corpus of isolated signs of Greek SL and a dataset of continuous finger-spelling in American SL, reporting significant gains by the inclusion of 3D hand pose information, while also outperforming the state-of-the-art on both databases. Further, we evaluate the 3D hand pose estimation technique as standalone.
更多查看译文
AI 理解论文
溯源树
样例
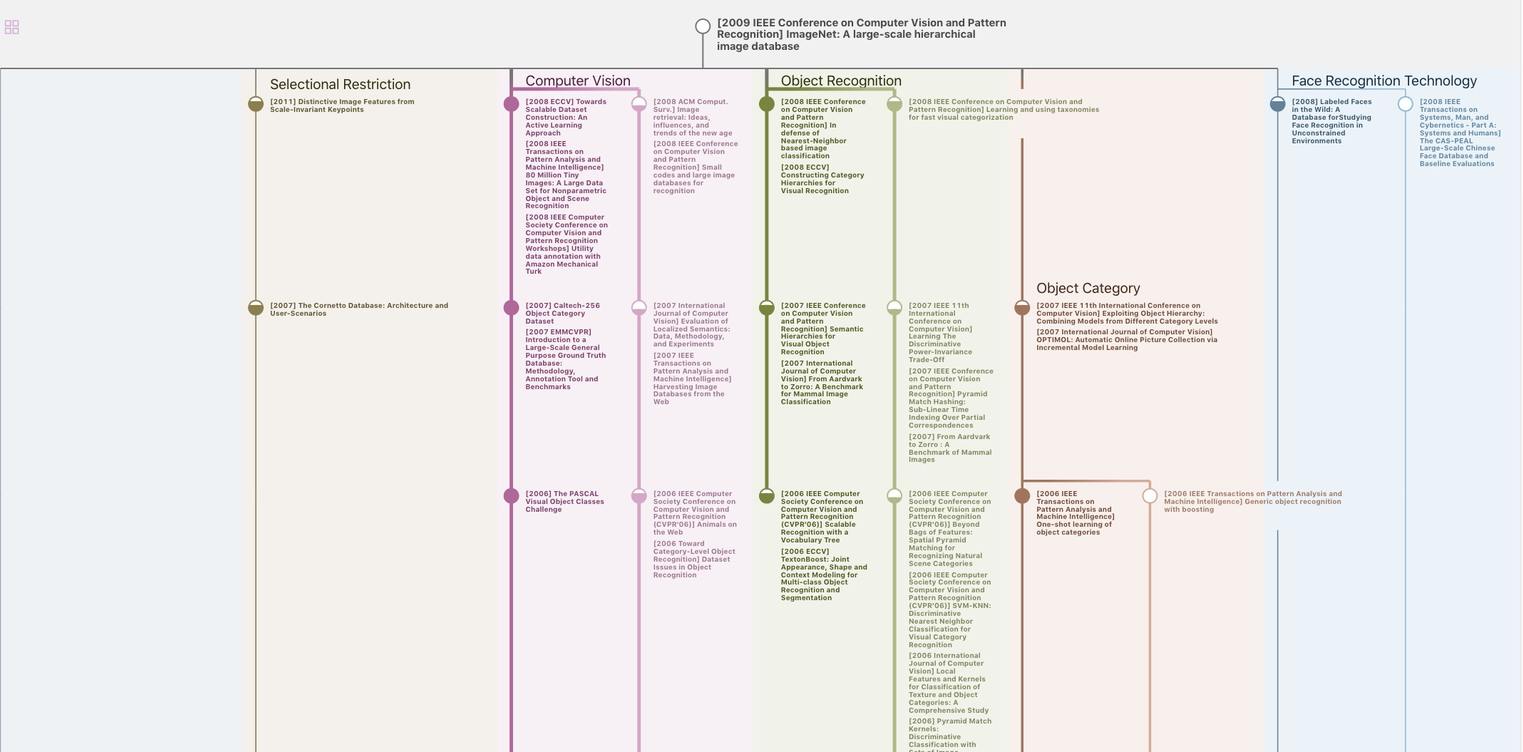
生成溯源树,研究论文发展脉络
数据免责声明
页面数据均来自互联网公开来源、合作出版商和通过AI技术自动分析结果,我们不对页面数据的有效性、准确性、正确性、可靠性、完整性和及时性做出任何承诺和保证。若有疑问,可以通过电子邮件方式联系我们:report@aminer.cn