Data-efficient reinforcement learning
semanticscholar(2021)
摘要
Data efficiency poses a major challenge for deep reinforcement learning. We approach this issue from the perspective of self-supervised representation learning, leveraging reward-free exploratory data to pretrain encoder networks. We employ a novel combination of latent dynamics modelling and goal-reaching objectives, which exploit the inherent structure of data in reinforcement learning. We demonstrate that our method scales well with network capacity and pretraining data. When evaluated on the Atari 100k data-efficiency benchmark, our approach significantly outperforms previous methods combining unsupervised pretraining with task-specific finetuning, and approaches human-level performance.
更多查看译文
AI 理解论文
溯源树
样例
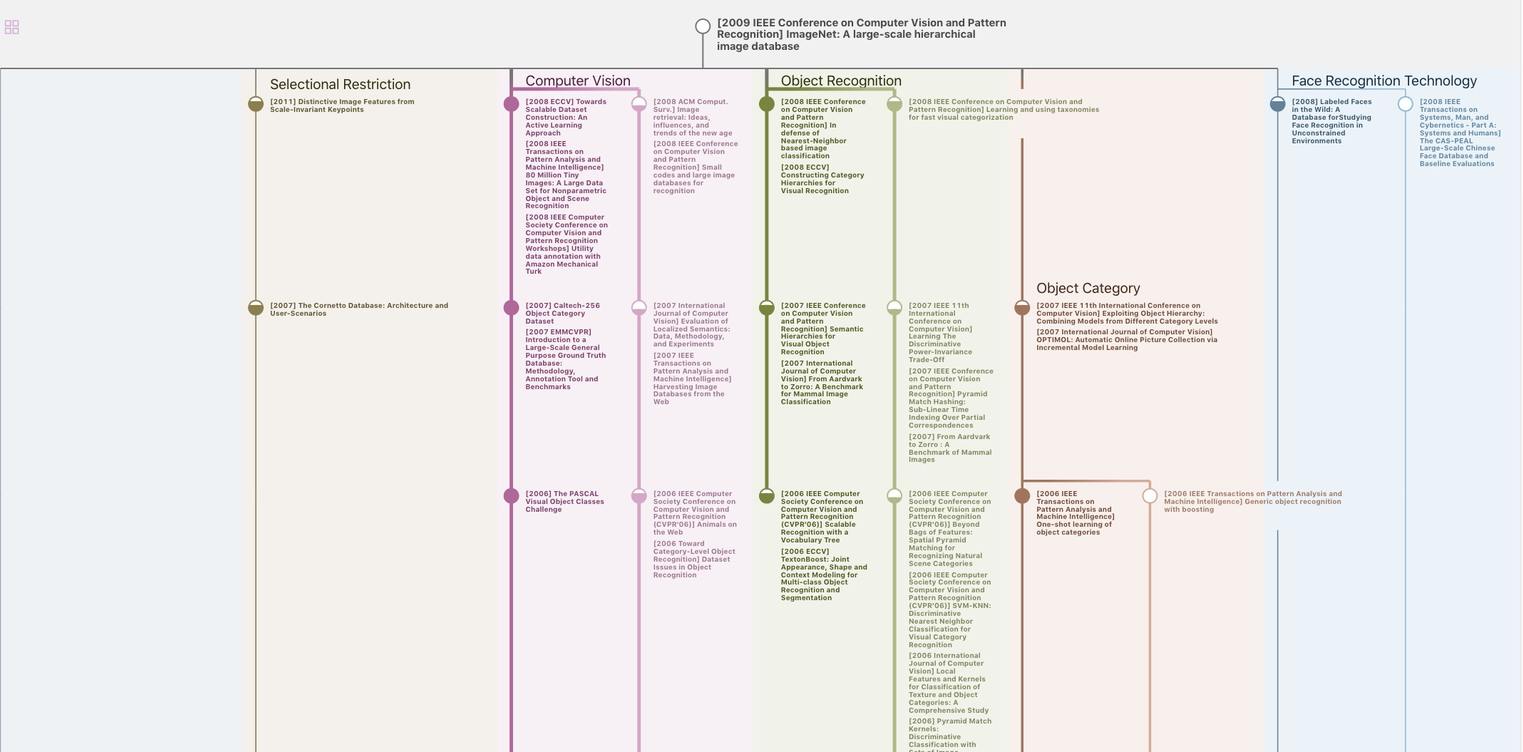
生成溯源树,研究论文发展脉络
数据免责声明
页面数据均来自互联网公开来源、合作出版商和通过AI技术自动分析结果,我们不对页面数据的有效性、准确性、正确性、可靠性、完整性和及时性做出任何承诺和保证。若有疑问,可以通过电子邮件方式联系我们:report@aminer.cn