A Crossbar Array of Magnetoresistive Memory Devices for In-Memory Computing
Nature(2022)
摘要
Implementations of artificial neural networks that borrow analogue techniques could potentially offer low-power alternatives to fully digital approaches 1 – 3 . One notable example is in-memory computing based on crossbar arrays of non-volatile memories 4 – 7 that execute, in an analogue manner, multiply–accumulate operations prevalent in artificial neural networks. Various non-volatile memories—including resistive memory 8 – 13 , phase-change memory 14 , 15 and flash memory 16 – 19 —have been used for such approaches. However, it remains challenging to develop a crossbar array of spin-transfer-torque magnetoresistive random-access memory (MRAM) 20 – 22 , despite the technology’s practical advantages such as endurance and large-scale commercialization 5 . The difficulty stems from the low resistance of MRAM, which would result in large power consumption in a conventional crossbar array that uses current summation for analogue multiply–accumulate operations. Here we report a 64 × 64 crossbar array based on MRAM cells that overcomes the low-resistance issue with an architecture that uses resistance summation for analogue multiply–accumulate operations. The array is integrated with readout electronics in 28-nanometre complementary metal–oxide–semiconductor technology. Using this array, a two-layer perceptron is implemented to classify 10,000 Modified National Institute of Standards and Technology digits with an accuracy of 93.23 per cent (software baseline: 95.24 per cent). In an emulation of a deeper, eight-layer Visual Geometry Group-8 neural network with measured errors, the classification accuracy improves to 98.86 per cent (software baseline: 99.28 per cent). We also use the array to implement a single layer in a ten-layer neural network to realize face detection with an accuracy of 93.4 per cent.
更多查看译文
关键词
Electrical and electronic engineering,Neural circuits,Science,Humanities and Social Sciences,multidisciplinary
AI 理解论文
溯源树
样例
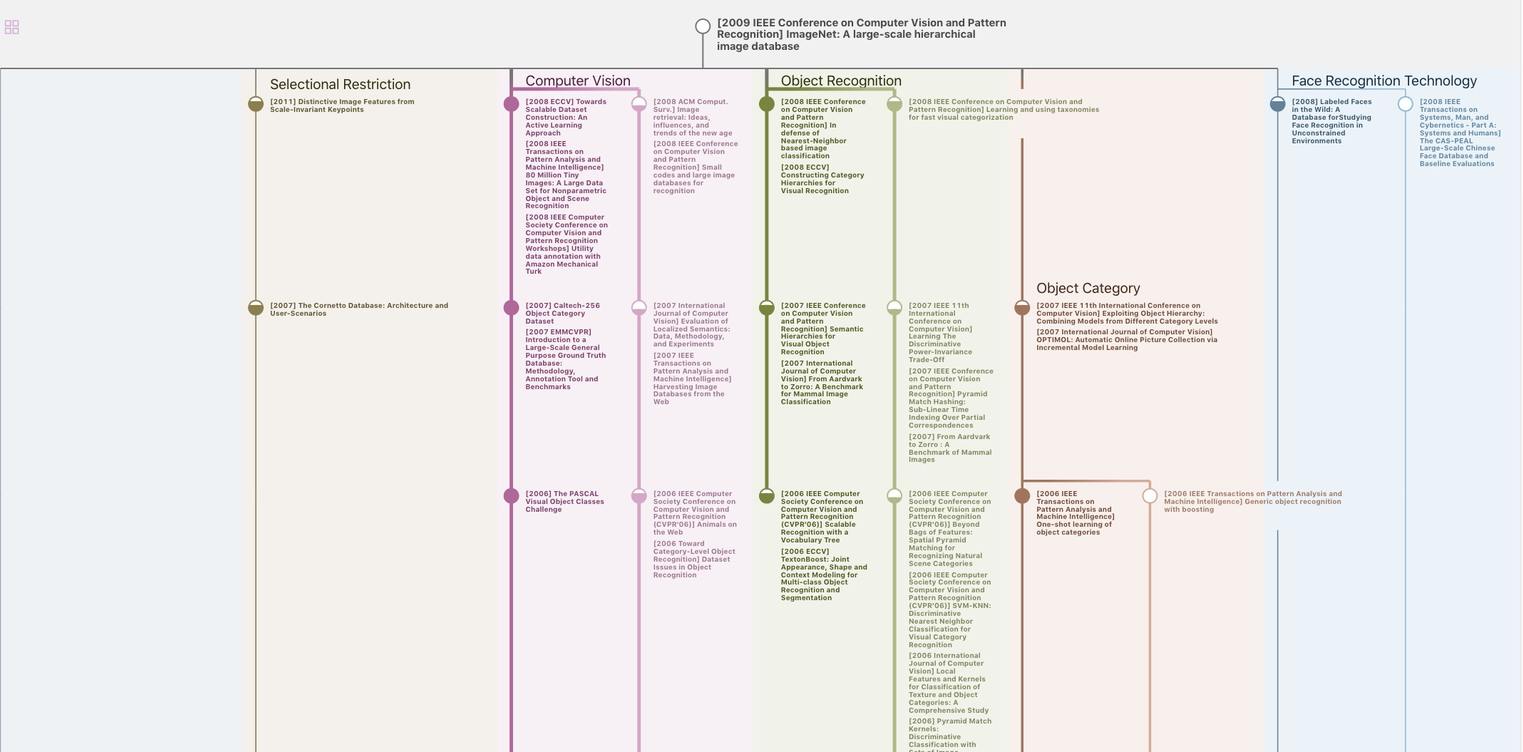
生成溯源树,研究论文发展脉络
数据免责声明
页面数据均来自互联网公开来源、合作出版商和通过AI技术自动分析结果,我们不对页面数据的有效性、准确性、正确性、可靠性、完整性和及时性做出任何承诺和保证。若有疑问,可以通过电子邮件方式联系我们:report@aminer.cn