TrustAL: Trustworthy Active Learning Using Knowledge Distillation
Proceedings of the AAAI Conference on Artificial Intelligence(2022)
摘要
Active learning can be defined as iterations of data labeling, model training, and data acquisition, until sufficient labels are acquired. A traditional view of data acquisition is that, through iterations, knowledge from human labels and models is implicitly distilled to monotonically increase the accuracy and label consistency. Under this assumption, the most recently trained model is a good surrogate for the current labeled data, from which data acquisition is requested based on uncertainty/diversity. Our contribution is debunking this myth and proposing a new objective for distillation. First, we found example forgetting, which indicates the loss of knowledge learned across iterations. Second, for this reason, the last model is no longer the best teacher-- For mitigating such forgotten knowledge, we select one of its predecessor models as a teacher, by our proposed notion of "consistency". We show that this novel distillation is distinctive in the following three aspects; First, consistency ensures to avoid forgetting labels. Second, consistency improves both uncertainty/diversity of labeled data. Lastly, consistency redeems defective labels produced by human annotators.
更多查看译文
关键词
Machine Learning (ML)
AI 理解论文
溯源树
样例
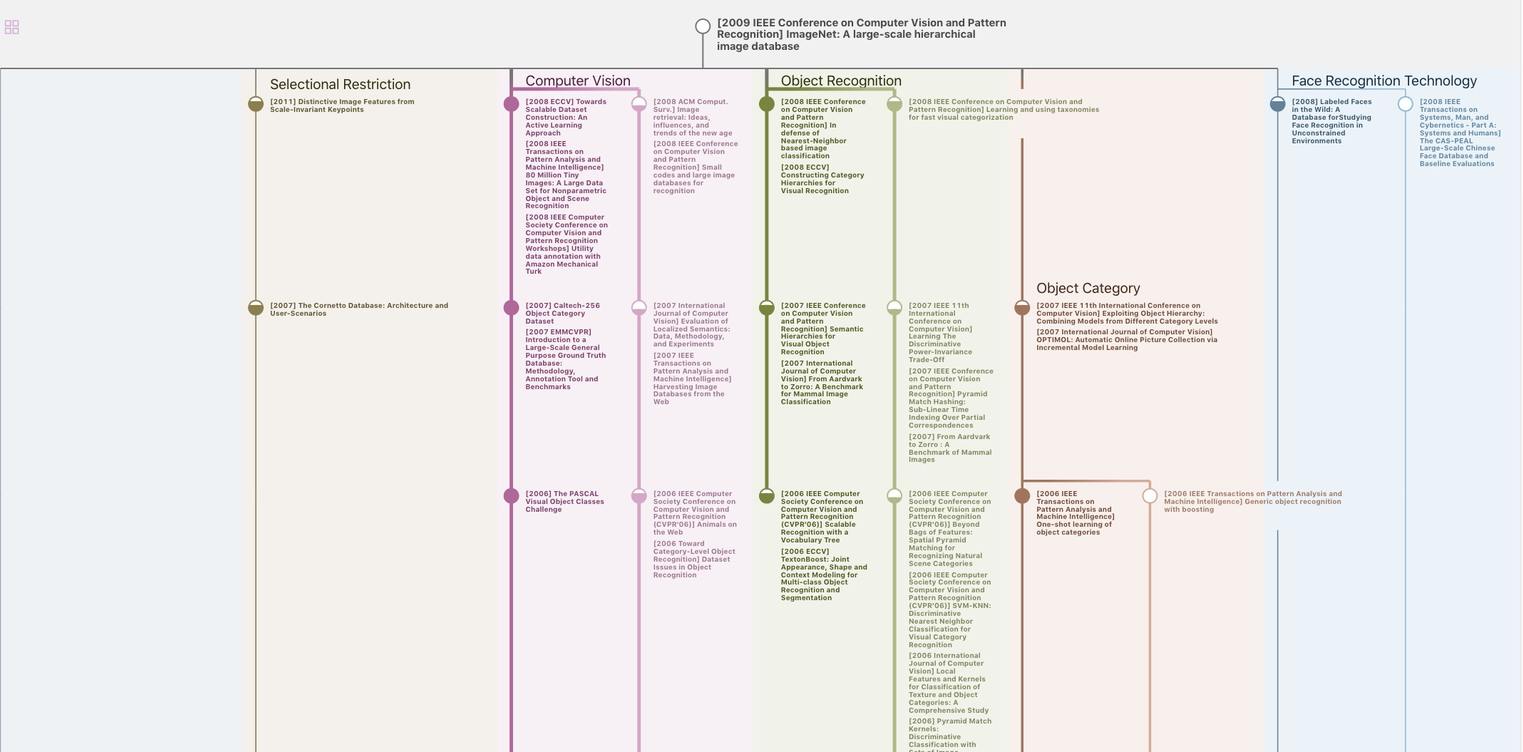
生成溯源树,研究论文发展脉络
数据免责声明
页面数据均来自互联网公开来源、合作出版商和通过AI技术自动分析结果,我们不对页面数据的有效性、准确性、正确性、可靠性、完整性和及时性做出任何承诺和保证。若有疑问,可以通过电子邮件方式联系我们:report@aminer.cn