Intelligent Camera Selection Decisions for Target Tracking in a Camera Network
2022 IEEE/CVF Winter Conference on Applications of Computer Vision (WACV)(2022)
摘要
Camera Selection Decisions (CSD) are highly useful for several applications in a multi-camera network. For example, CSD benefit multi-camera target tracking by reducing the number of candidate cameras to look for the target’s next location. The correct candidate cameras, decreases the number of false Re-ID queries as well as the computation time. Also, in multi-camera trajectory forecasting (MCTF) to predict where a person will re-appear in the camera network along with the transition time. These applications require a large amount of annotated data for training. In this paper, we use state-representation learning with a reinforcement learning based policy to effectively and efficiently make camera selection decisions. We further demonstrate that by using learned state representations, as opposed to hand-crafted state variables, we are able to achieve state-of-the-art results on camera selection, while reducing the training time for the RL policy. Along with this, we use a reward function that helps to reduce the amount of supervision in training the policy in a semi-supervised way. We report our results on four datasets: NLPR_MCT, DukeMTMC, CityFlow, and WNMF dataset. We show that an RL policy reduces unnecessary Re-ID queries and therefore the false alarms, scales well to larger camera networks, and is target-agnostic.
更多查看译文
关键词
Vision Systems and Applications Multimedia Applications,Security/Surveillance,Transfer,Few-shot,Semi- and Un- supervised Learning
AI 理解论文
溯源树
样例
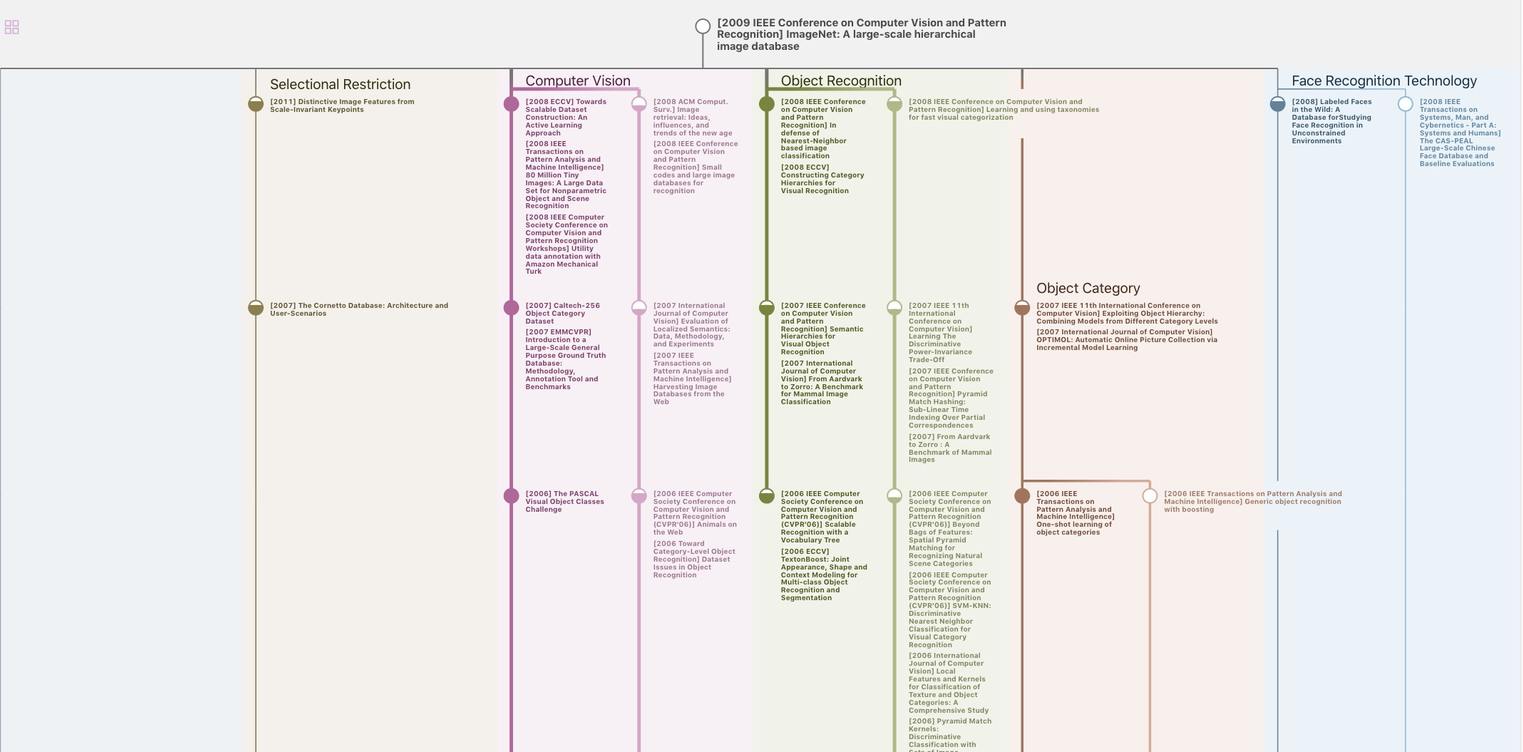
生成溯源树,研究论文发展脉络
数据免责声明
页面数据均来自互联网公开来源、合作出版商和通过AI技术自动分析结果,我们不对页面数据的有效性、准确性、正确性、可靠性、完整性和及时性做出任何承诺和保证。若有疑问,可以通过电子邮件方式联系我们:report@aminer.cn