Unsupervised learning of two-component nematicity from STM data on magic angle bilayer graphene
arXiv (Cornell University)(2022)
摘要
Moir\'e materials such as magic angle twisted bilayer graphene (MATBG) exhibit remarkable phenomenology, but present significant challenges for certain experimental methods, particularly scanning probes such as scanning tunneling microscopy (STM). Typical STM studies that can image tens of thousands of atomic unit cells can image roughly ten moir\'e cells, making data analysis statistically fraught. Here, we propose a method to mitigate this problem by aggregating STM conductance data from several bias voltages, and then using the unsupervised machine learning method of gaussian mixture model clustering to draw maximal insight from the resulting dataset. We apply this method, using as input coarse-grained bond variables respecting the point group symmetry, to investigate nematic ordering tendencies in MATBG for both charge neutral and hole-doped samples. For the charge-neutral dataset, the clustering reveals the surprising coexistence of multiple types of nematicity that are unrelated by symmetry, and therefore generically nondegenerate. By contrast, the clustering in the hole doped data is consistent with long range order of a single type. Beyond its value in analyzing nematicity in MATBG, our method has the potential to enhance understanding of symmetry breaking and its spatial variation in a variety of moir\'e materials.
更多查看译文
关键词
angle bilayer graphene,unsupervised learning,two-component
AI 理解论文
溯源树
样例
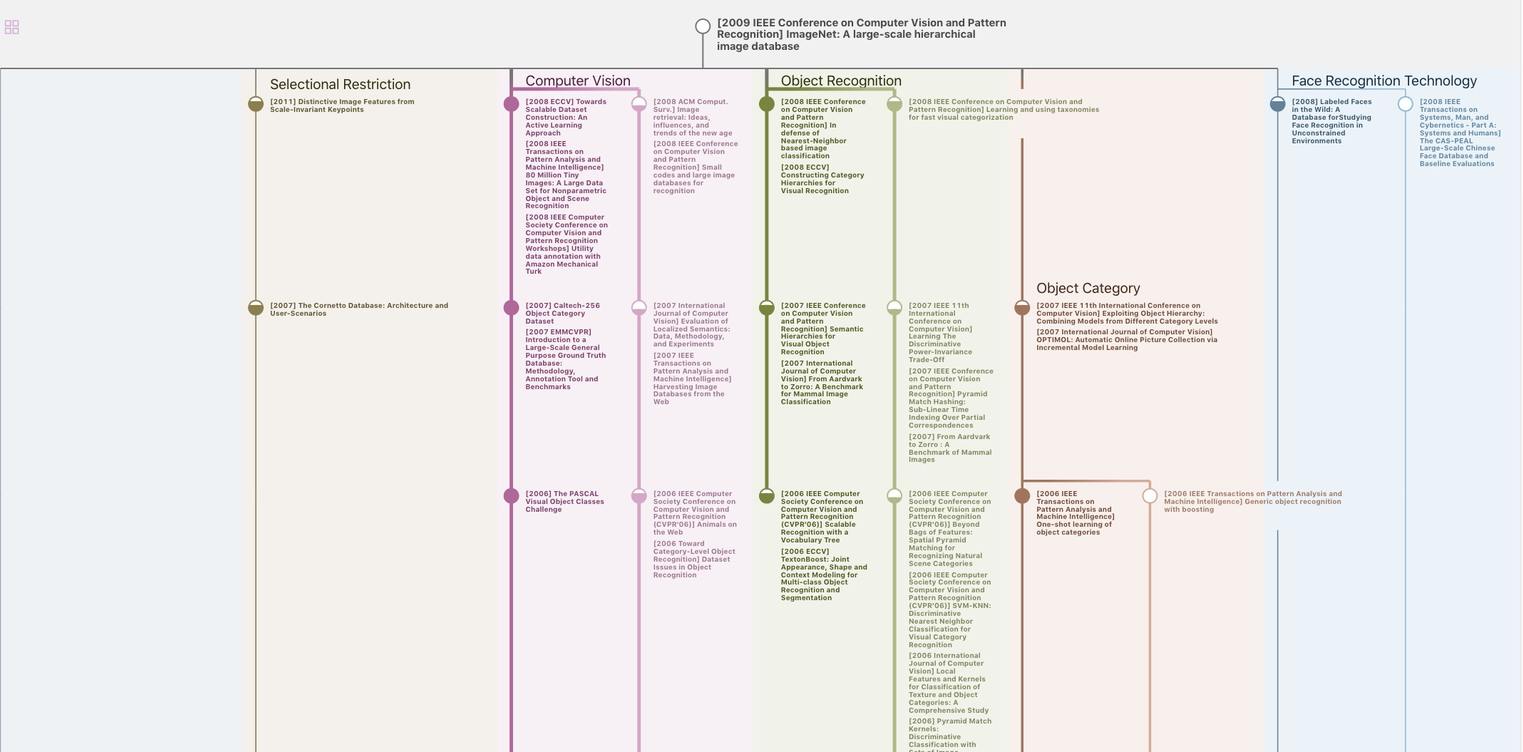
生成溯源树,研究论文发展脉络
数据免责声明
页面数据均来自互联网公开来源、合作出版商和通过AI技术自动分析结果,我们不对页面数据的有效性、准确性、正确性、可靠性、完整性和及时性做出任何承诺和保证。若有疑问,可以通过电子邮件方式联系我们:report@aminer.cn