Federated Reinforcement Learning with Environment Heterogeneity
arXiv (Cornell University)(2022)
摘要
We study a Federated Reinforcement Learning (FedRL) problem in which n agents collaboratively learn a single policy without sharing the trajectories they collected during agent-environment interaction. We stress the constraint of environment heterogeneity, which means n environments corresponding to these n agents have different state transitions. To obtain a value function or a policy function which optimizes the overall performance in all environments, we propose two federated RL algorithms, QAvg and PAvg. We theoretically prove that these algorithms converge to suboptimal solutions, while such sub-optimality depends on how heterogeneous these n environments are. Moreover, we propose a heuristic that achieves personalization by embedding the n environments into n vectors. The personalization heuristic not only improves the training but also allows for better generalization to new environments.
更多查看译文
关键词
Reinforcement Learning
AI 理解论文
溯源树
样例
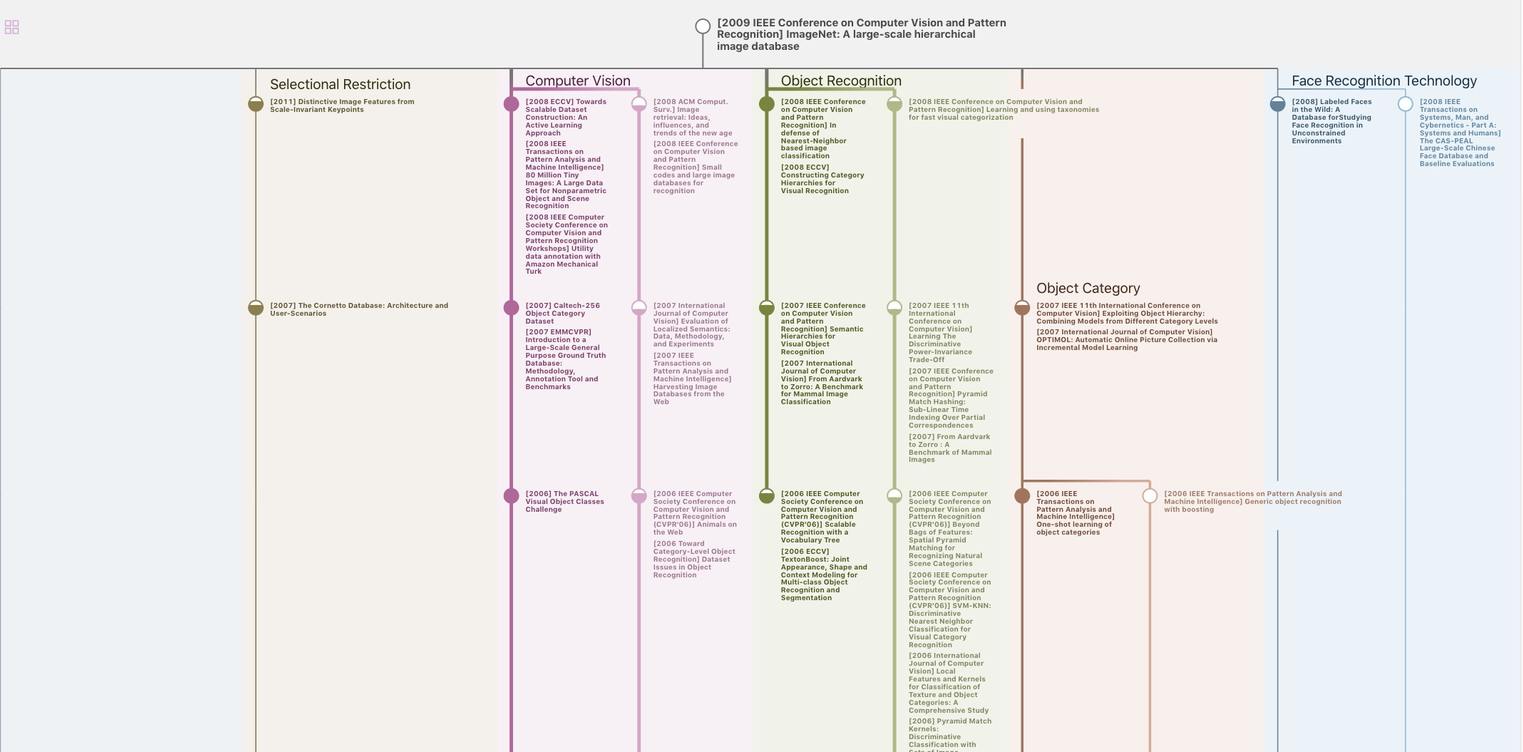
生成溯源树,研究论文发展脉络
数据免责声明
页面数据均来自互联网公开来源、合作出版商和通过AI技术自动分析结果,我们不对页面数据的有效性、准确性、正确性、可靠性、完整性和及时性做出任何承诺和保证。若有疑问,可以通过电子邮件方式联系我们:report@aminer.cn