Language is Strong, Vision is Not: A Diagnostic Study of the Limitations of the Embodied Question Answering Task
semanticscholar(2022)
摘要
We examine the limitations of the Embodied 001 Question Answering (EQA) task, the dataset 002 and the models (Das et al., 2018). We observe 003 that the role of vision in EQA is small, and the 004 models often exploit language biases found in 005 the dataset. We demonstrate that perturbing 006 vision at different levels (incongruent, black or 007 random noise images) still allows the models to 008 learn from general visual patterns, suggesting 009 that they capture some common sense reason- 010 ing about the visual world. We argue that a 011 better set of data and models are required to 012 achieve better performance in predicting (gen- 013 erating) correct answers. We make the code 014 used in the experiments available here: [the 015 GitHub link placeholder]. 016
更多查看译文
AI 理解论文
溯源树
样例
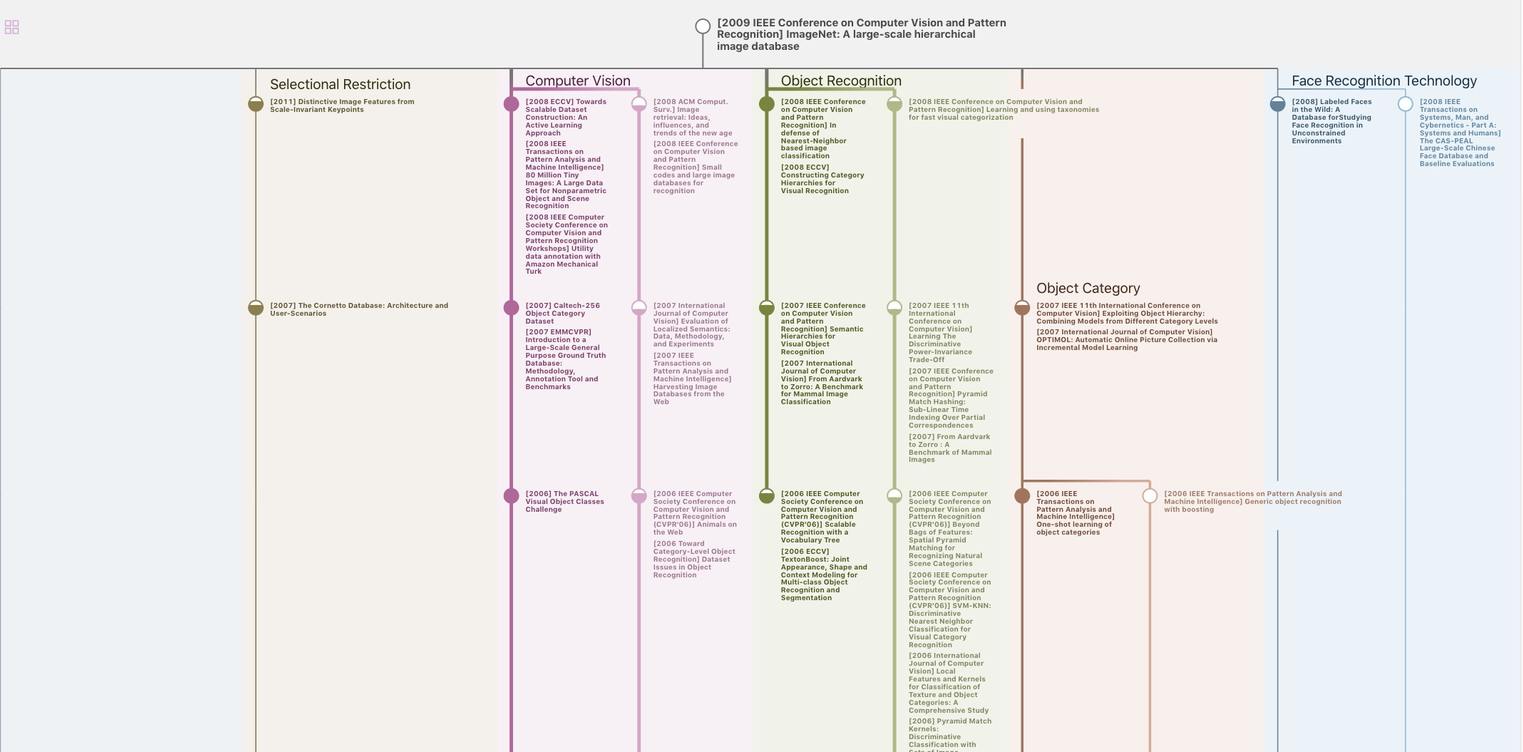
生成溯源树,研究论文发展脉络
数据免责声明
页面数据均来自互联网公开来源、合作出版商和通过AI技术自动分析结果,我们不对页面数据的有效性、准确性、正确性、可靠性、完整性和及时性做出任何承诺和保证。若有疑问,可以通过电子邮件方式联系我们:report@aminer.cn