Surround-Net: A Multi-Branch Arbitrary-Oriented Detector for Remote Sensing
Remote Sensing(2022)
摘要
With the development of oriented object detection technology, especially in the area of remote sensing, significant progress has been made, and multiple excellent detection architectures have emerged. Oriented detection architectures can be broadly divided into five-parameter systems and eight-parameter systems that encounter the periodicity problem of angle regression and the discontinuous problem of vertex regression during training, respectively. Therefore, we propose a new multi-branch anchor-free one-stage model that can effectively alleviate the corner case when representing rotating objects, called Surround-Net. The creative contribution submitted in this paper mainly includes three aspects. Firstly, a multi-branch strategy is adopted to make the detector choose the best regression path adaptively for the discontinuity problem. Secondly, to address the inconsistency between classification and quality estimation (location), a modified high-dimensional Focal Loss and a new Surround IoU Loss are proposed to enhance the unity ability of the features. Thirdly, in the refined process after backbone feature extraction, a center vertex attention mechanism is adopted to deal with the environmental noise introduced in the remote sensing images. This type of auxiliary module is able to focus the model’s attention on the boundary of the bounding box. Finally, extensive experiments were carried out on the DOTA dataset, and the results demonstrate that Surround-Net can solve regression boundary problems and can achieve a more competitive performance (e.g., 75.875 mAP) than other anchor-free one-stage detectors with higher speeds.
更多查看译文
关键词
object detection,anchor-free,multi-branch,sliding ratios,eight-parameter regression
AI 理解论文
溯源树
样例
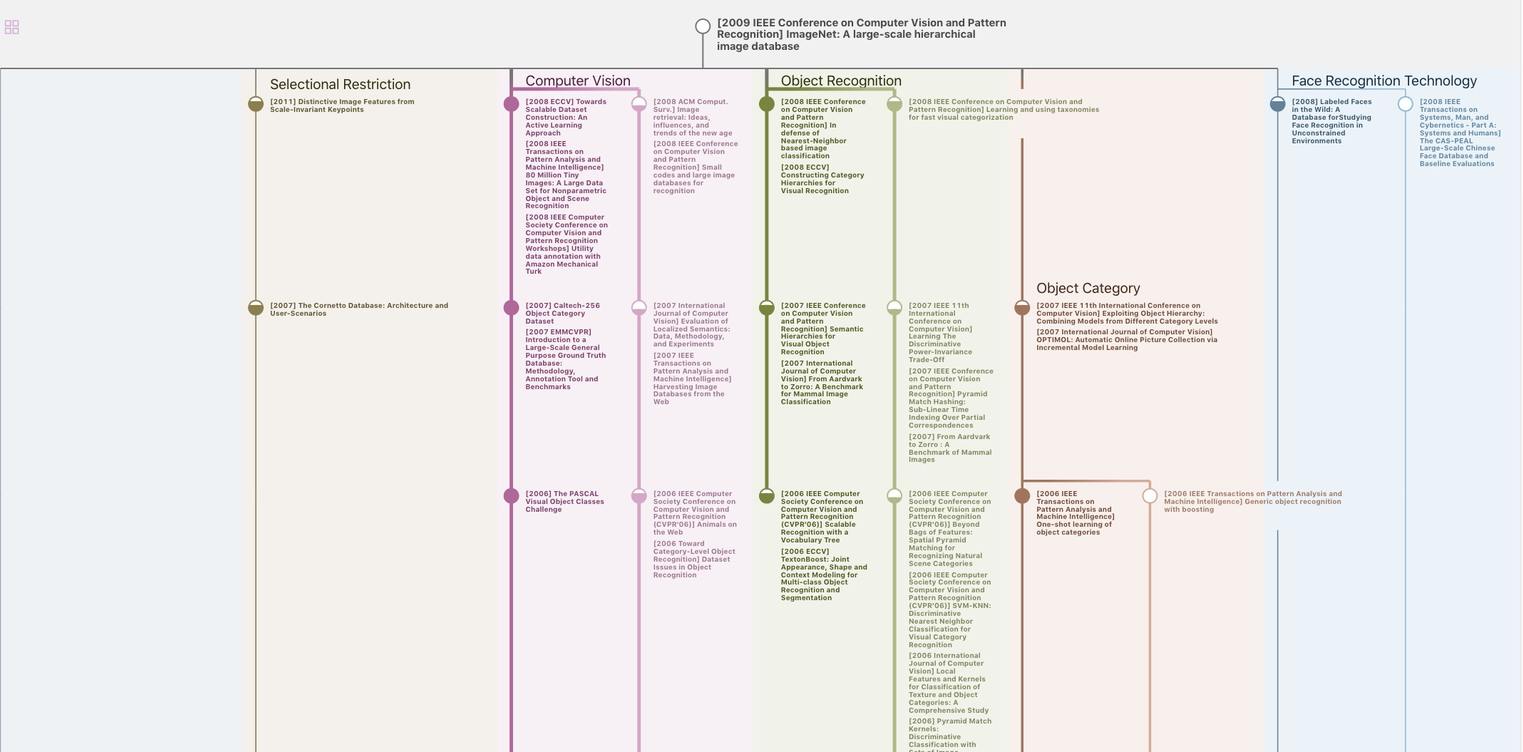
生成溯源树,研究论文发展脉络
数据免责声明
页面数据均来自互联网公开来源、合作出版商和通过AI技术自动分析结果,我们不对页面数据的有效性、准确性、正确性、可靠性、完整性和及时性做出任何承诺和保证。若有疑问,可以通过电子邮件方式联系我们:report@aminer.cn