Multiview Deep Anomaly Detection: A Systematic Exploration.
IEEE TRANSACTIONS ON NEURAL NETWORKS AND LEARNING SYSTEMS(2024)
摘要
Anomaly detection (AD), which models a given normal class and distinguishes it from the rest of abnormal classes, has been a long-standing topic with ubiquitous applications. As modern scenarios often deal with massive high-dimensional complex data spawned by multiple sources, it is natural to consider AD from the perspective of multiview deep learning. However, it has not been formally discussed by the literature and remains underexplored. Motivated by this blank, this article makes fourfold contributions: First, to the best of our knowledge, this is the first work that formally identifies and formulates the multiview deep AD problem. Second, we take recent advances in relevant areas into account and systematically devise various baseline solutions, which lays the foundation for multiview deep AD research. Third, to remedy the problem that limited benchmark datasets are available for multiview deep AD, we extensively collect the existing public data and process them into more than 30 multiview benchmark datasets via multiple means, so as to provide a better evaluation platform for multiview deep AD. Finally, by comprehensively evaluating the devised solutions on different types of multiview deep AD benchmark datasets, we conduct a thorough analysis on the effectiveness of the designed baselines and hopefully provide other researchers with beneficial guidance and insight into the new multiview deep AD topic.
更多查看译文
关键词
Deep learning,Data models,Benchmark testing,Task analysis,Anomaly detection,Training,Systematics,Deep anomaly detection (AD),multiview deep AD,multiview deep learning
AI 理解论文
溯源树
样例
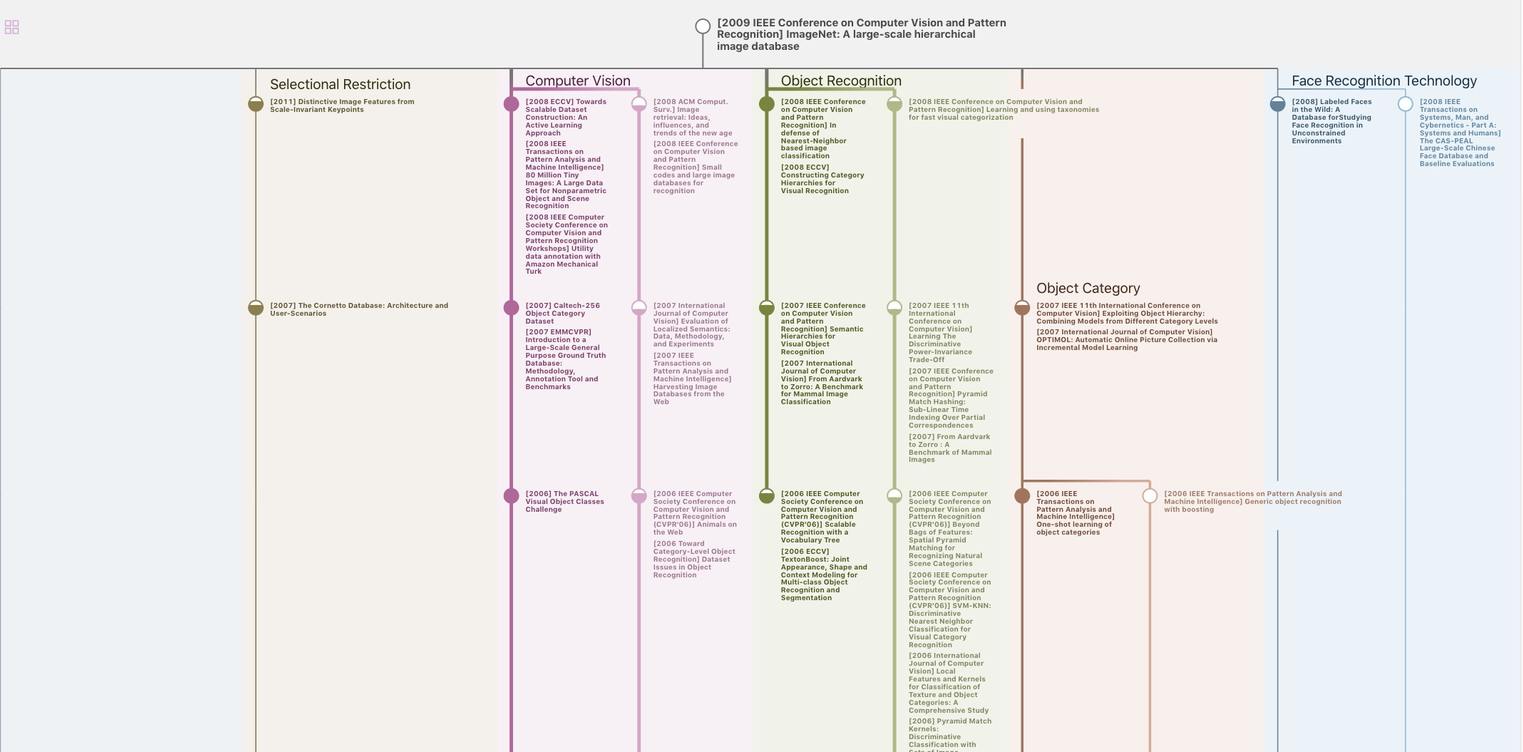
生成溯源树,研究论文发展脉络
数据免责声明
页面数据均来自互联网公开来源、合作出版商和通过AI技术自动分析结果,我们不对页面数据的有效性、准确性、正确性、可靠性、完整性和及时性做出任何承诺和保证。若有疑问,可以通过电子邮件方式联系我们:report@aminer.cn