M3T: Three-Dimensional Medical Image Classifier Using Multi-plane and Multi-slice Transformer
2022 IEEE/CVF CONFERENCE ON COMPUTER VISION AND PATTERN RECOGNITION (CVPR 2022)(2022)
摘要
In this study, we propose a three-dimensional Medical image classifier using Multi-plane and Multi-slice Trans-former (M3T) network to classify Alzheimer's disease (AD) in 3D MRI images. The proposed network synergically com-bines 3D CNN, 2D CNN, and Transformer for accurate AD classification. The 3D CNN is used to perform natively 3D representation learning, while 2D CNN is used to utilize the pre-trained weights on large 2D databases and 2D repre-sentation learning. It is possible to efficiently extract the lo-cality information for AD-related abnormalities in the local brain using CNN networks with inductive bias. The trans-former network is also used to obtain attention relationships among multi-plane (axial, coronal, and sagittal) and multi-slice images after CNN. It is also possible to learn the ab-normalities distributed over the wider region in the brain using the transformer without inductive bias. In this ex-periment, we used a training dataset from the Alzheimer's Disease Neuroimaging Initiative (ADNI) which contains a total of 4,786 3D T1-weighted MRI images. For the validation data, we used dataset from three different institutions: The Australian Imaging, Biomarker and Lifestyle Flagship Study of Ageing (AIBL), The Open Access Series of Imaging Studies (OASIS), and some set of ADNI data indepen-dent from the training dataset. Our proposed M3T is compared to conventional 3D classification networks based on an area under the curve (AUC) and classification accuracy for AD classification. This study represents that the pro-posed network M3T achieved the highest performance in multi-institutional validation database, and demonstrates the feasibility of the method to efficiently combine CNN and Transformer for 3D medical images.
更多查看译文
关键词
Medical,biological and cell microscopy,Deep learning architectures and techniques
AI 理解论文
溯源树
样例
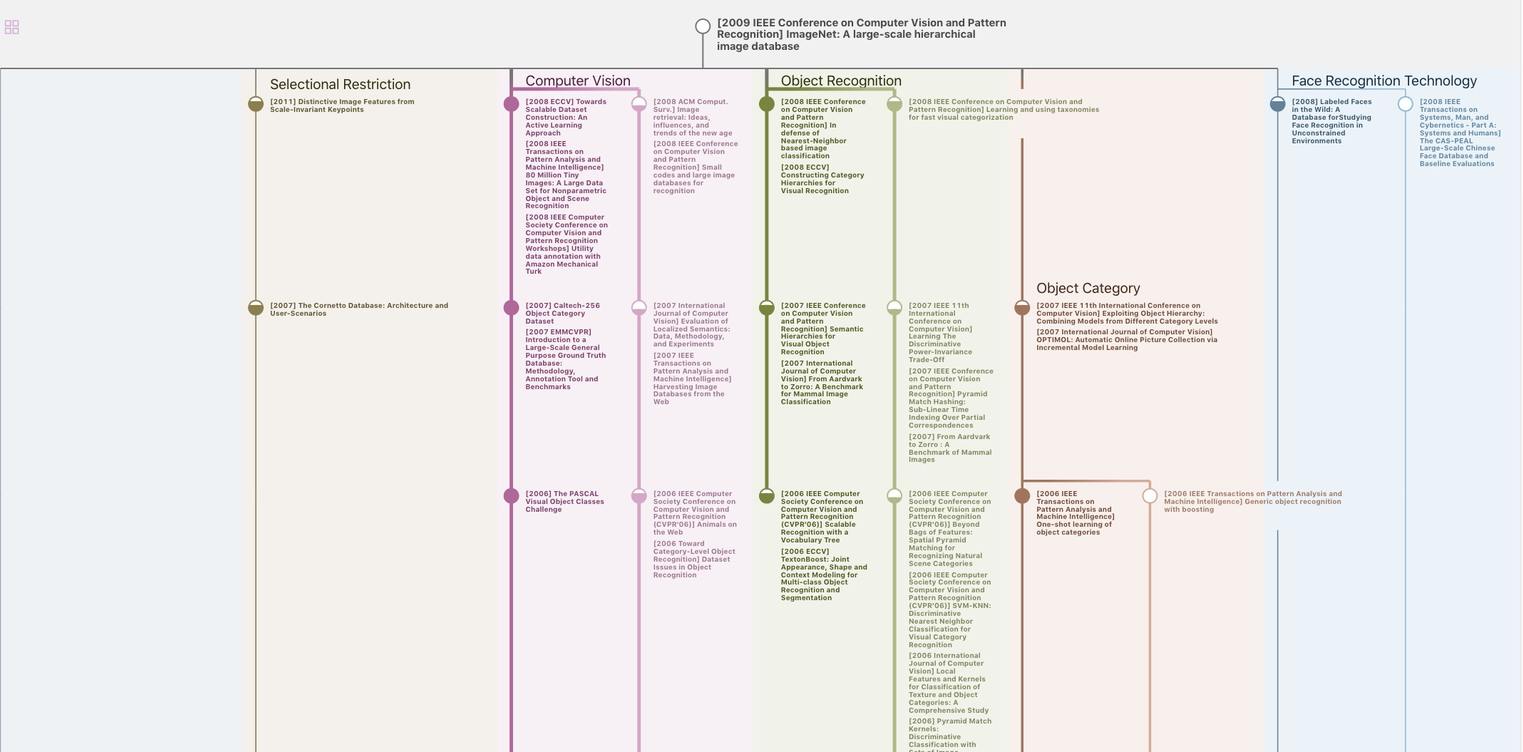
生成溯源树,研究论文发展脉络
数据免责声明
页面数据均来自互联网公开来源、合作出版商和通过AI技术自动分析结果,我们不对页面数据的有效性、准确性、正确性、可靠性、完整性和及时性做出任何承诺和保证。若有疑问,可以通过电子邮件方式联系我们:report@aminer.cn