Provable Adaptivity of Adam under Non-uniform Smoothness
KDD 2024(2024)
摘要
Adam is widely adopted in practical applications due to its fast convergence. However, its theoretical analysis is still far from satisfactory. Existing convergence analyses for Adam rely on the bounded smoothness assumption, referred to as the L-smooth condition. Unfortunately, this assumption does not hold for many deep learning tasks. Moreover, we believe that this assumption obscures the true benefit of Adam, as the algorithm can adapt its update magnitude according to local smoothness. This important feature of Adam becomes irrelevant when assuming globally bounded smoothness. This paper studies the convergence of randomly reshuffled Adam (RR Adam) with diminishing learning rate, which is the major version of Adam adopted in deep learning tasks. We present the first convergence analysis of RR Adam without the bounded smoothness assumption. We demonstrate that RR Adam can maintain its convergence properties when smoothness is linearly bounded by the gradient norm, referred to as the (L0, L1)-smooth condition. We further compare Adam to SGD when both methods use diminishing learning rate. We refine the existing lower bound of SGD and show that SGD can be slower than Adam. To our knowledge, this is the first time that Adam and SGD are rigorously compared in the same setting and the advantage of Adam is revealed.
更多查看译文
关键词
Adam Optimization,Non-uniform Smoothness,Convergence Analysis,Diminishing Learning Rate,(L0, L1)-Smooth Condition,SGD Comparison
AI 理解论文
溯源树
样例
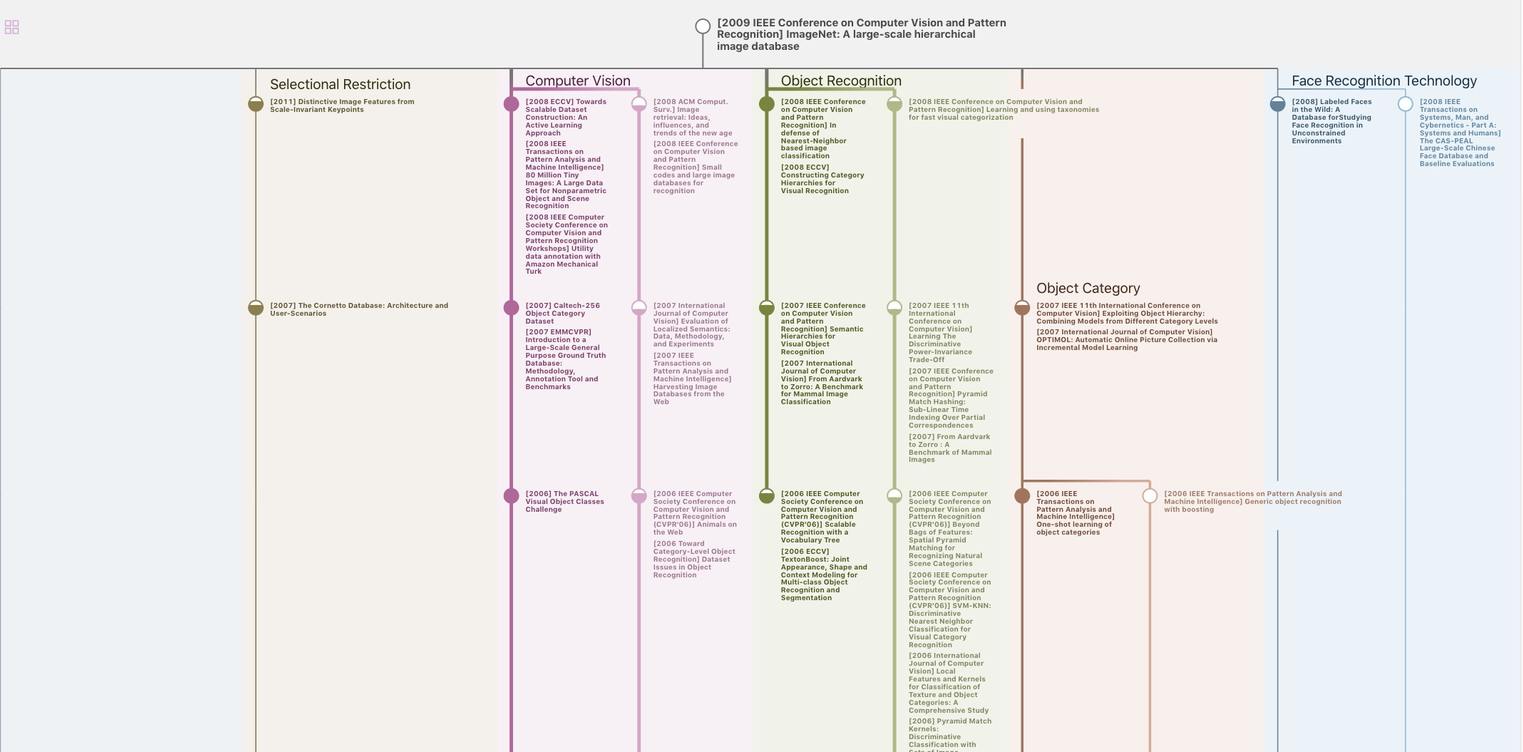
生成溯源树,研究论文发展脉络
数据免责声明
页面数据均来自互联网公开来源、合作出版商和通过AI技术自动分析结果,我们不对页面数据的有效性、准确性、正确性、可靠性、完整性和及时性做出任何承诺和保证。若有疑问,可以通过电子邮件方式联系我们:report@aminer.cn