AI-based chest CT semantic segmentation algorithm enables semi-automated lung cancer surgery planning by recognizing anatomical variants of pulmonary vessels
FRONTIERS IN ONCOLOGY(2022)
摘要
BackgroundThe recognition of anatomical variants is essential in preoperative planning for lung cancer surgery. Although three-dimensional (3-D) reconstruction provided an intuitive demonstration of the anatomical structure, the recognition process remains fully manual. To render a semiautomated approach for surgery planning, we developed an artificial intelligence (AI)-based chest CT semantic segmentation algorithm that recognizes pulmonary vessels on lobular or segmental levels. Hereby, we present a retrospective validation of the algorithm comparing surgeons' performance. MethodsThe semantic segmentation algorithm to be validated was trained on non-contrast CT scans from a single center. A retrospective pilot study was performed. An independent validation dataset was constituted by an arbitrary selection from patients who underwent lobectomy or segmentectomy in three institutions during Apr. 2020 to Jun. 2021. The golden standard of anatomical variants of each enrolled case was obtained via expert surgeons' judgments based on chest CT, 3-D reconstruction, and surgical observation. The performance of the algorithm is compared against the performance of two junior thoracic surgery attendings based on chest CT. ResultsA total of 27 cases were included in this study. The overall case-wise accuracy of the AI model was 82.8% in pulmonary vessels compared to 78.8% and 77.0% for the two surgeons, respectively. Segmental artery accuracy was 79.7%, 73.6%, and 72.7%; lobular vein accuracy was 96.3%, 96.3%, and 92.6% by the AI model and two surgeons, respectively. No statistical significance was found. In subgroup analysis, the anatomic structure-wise analysis of the AI algorithm showed a significant difference in accuracies between different lobes (p = 0.012). Higher AI accuracy in the right-upper lobe (RUL) and left-lower lobe (LLL) arteries was shown. A trend of better performance in non-contrast CT was also detected. Most recognition errors by the algorithm were the misclassification of LA(1+2) and LA(3). Radiological parameters did not exhibit a significant impact on the performance of both AI and surgeons. ConclusionThe semantic segmentation algorithm achieves the recognition of the segmental pulmonary artery and the lobular pulmonary vein. The performance of the model approximates that of junior thoracic surgery attendings. Our work provides a novel semiautomated surgery planning approach that is potentially beneficial to lung cancer patients.
更多查看译文
关键词
pulmonary vessel,artificial intelligence,semantic segmentation,surgery planning,lung cancer
AI 理解论文
溯源树
样例
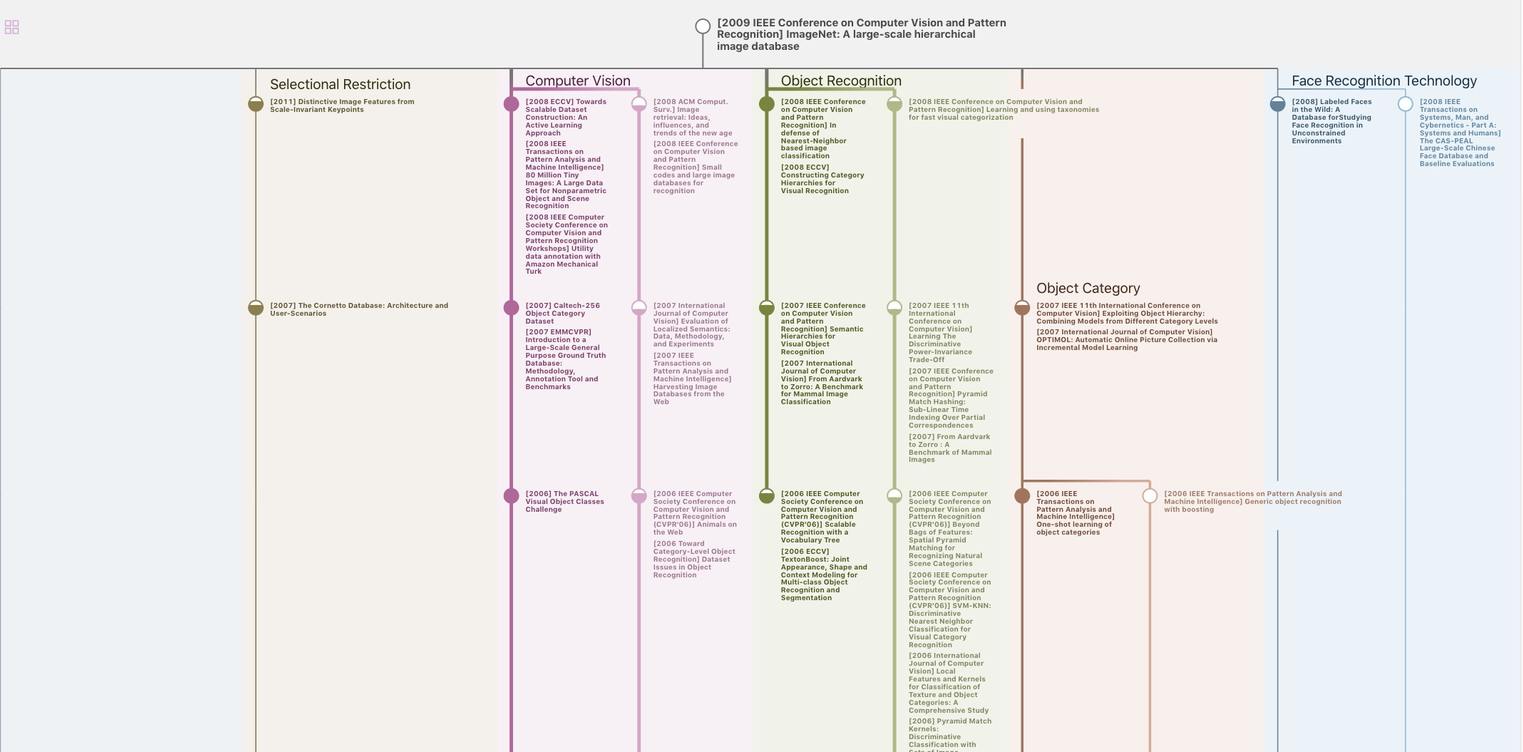
生成溯源树,研究论文发展脉络
数据免责声明
页面数据均来自互联网公开来源、合作出版商和通过AI技术自动分析结果,我们不对页面数据的有效性、准确性、正确性、可靠性、完整性和及时性做出任何承诺和保证。若有疑问,可以通过电子邮件方式联系我们:report@aminer.cn