A Systematic Review of Distributed Deep Learning Frameworks for Big Data
INTELLIGENT COMPUTING METHODOLOGIES, PT III(2022)
摘要
Traditional Machine Learning and Deep Learning techniques (data acquisition, preparation, model training and evaluation) take a lot of computational resources and time to produce even a simple prediction model, especially when implemented on a single machine. Intuitively, the demand for computational requirements is higher in case of management of Big Data and training of complex models. Thus, a paradigm shift from a single machine to a BD-oriented approach is required for making traditional Machine Learning and Deep Learning techniques fit to Big Data. In particular, it emerges the need for developing and deploying Big Data Analytics Infrastructures on cluster of machines. In this context, main features and principles of Distributed Deep Learning frameworks are here discussed. The main contribution of this paper is a systematic review of proposed solutions, aimed at investigating under a unifying lens their foundational elements, functional features and capabilities, despite the inherent literature fragmentation. To this, we conducted a literature search in Scopus and Google Scholar. This review also compares Distributed Deep Learning approaches according to more technical facets: implemented of parallelism techniques, supported hardware, model parameters sharing modalities, computation modalities for stochastic gradient descent and compatibility with other frameworks.
更多查看译文
关键词
Big Data,Distributed Deep Learning,Parallel computing,Distributed Deep Learning Frameworks
AI 理解论文
溯源树
样例
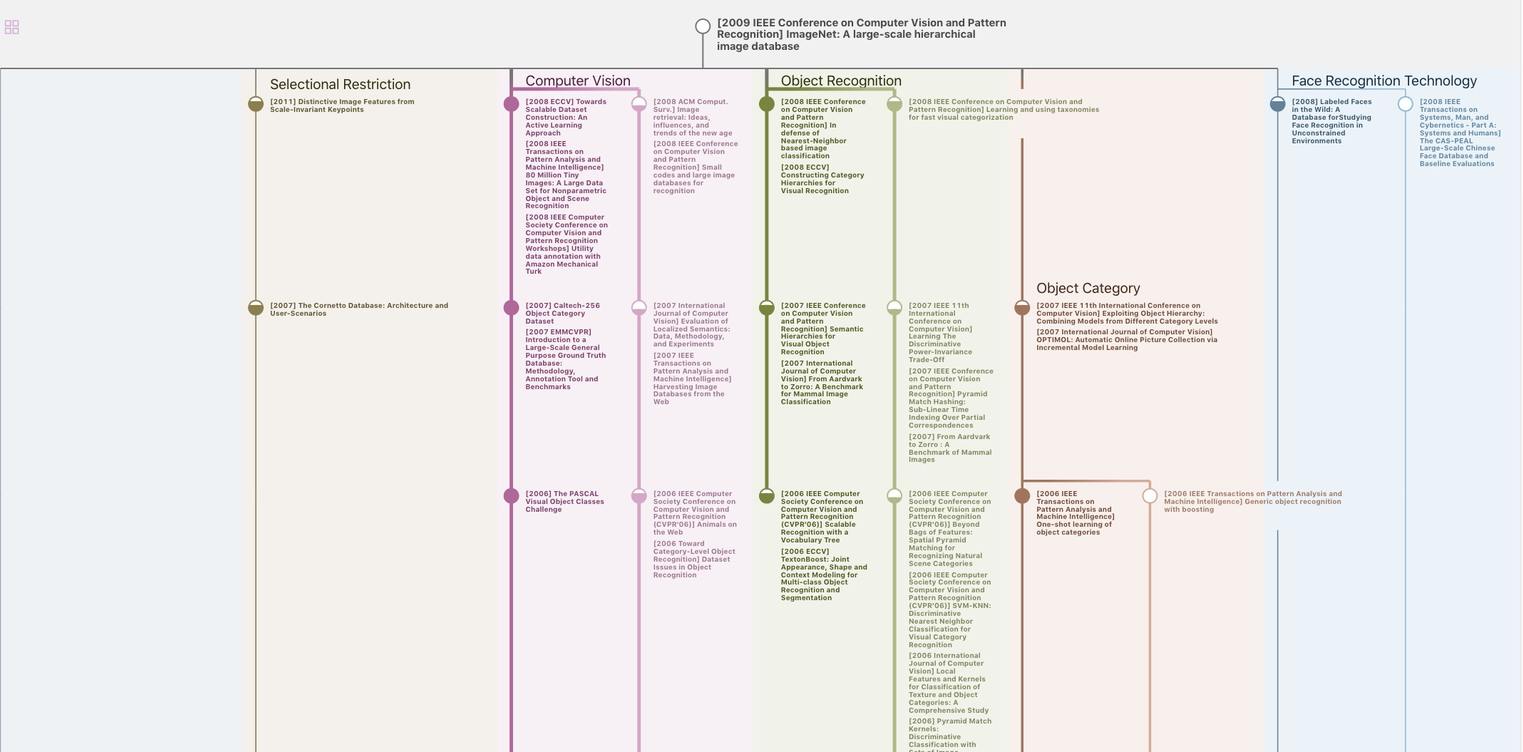
生成溯源树,研究论文发展脉络
数据免责声明
页面数据均来自互联网公开来源、合作出版商和通过AI技术自动分析结果,我们不对页面数据的有效性、准确性、正确性、可靠性、完整性和及时性做出任何承诺和保证。若有疑问,可以通过电子邮件方式联系我们:report@aminer.cn