Deffini: A Family-Specific Deep Neural Network Model for Structure-Based Virtual Screening
Computers in Biology and Medicine(2022)
摘要
Deep learning-based virtual screening methods have been shown to significantly improve the accuracy of traditional docking-based virtual screening methods. In this paper, we developed Deffini, a structure-based virtual screening neural network model. During training, Deffini learns protein-ligand docking poses to distinguish actives and decoys and then to predict whether a new ligand will bind to the protein target. Deffini outperformed Smina with an average AUC ROC of 0.92 and AUC PRC of 0.44 in 3-fold cross-validation on the benchmark dataset DUD-E. However, when tested on the maximum unbiased validation (MUV) dataset, Deffini achieved poor results with an average AUC ROC of 0.517. We used the family-specific training approach to train the model to improve the model performance and concluded that family-specific models performed better than the pan-family models. To explore the limits of the predictive power of the family-specific models, we constructed Kernie, a new protein kinase dataset consisting of 358 kinases. Deffini trained with the Kernie dataset outperformed all recent benchmarks on the MUV kinases, with an average AUC ROC of 0.745, which highlights the importance of quality datasets in improving the performance of deep neural network models and the importance of using family-specific models.
更多查看译文
关键词
Virtual screening,Protein family-specific model,Structure-based,Convolutional neural network,Drug discovery
AI 理解论文
溯源树
样例
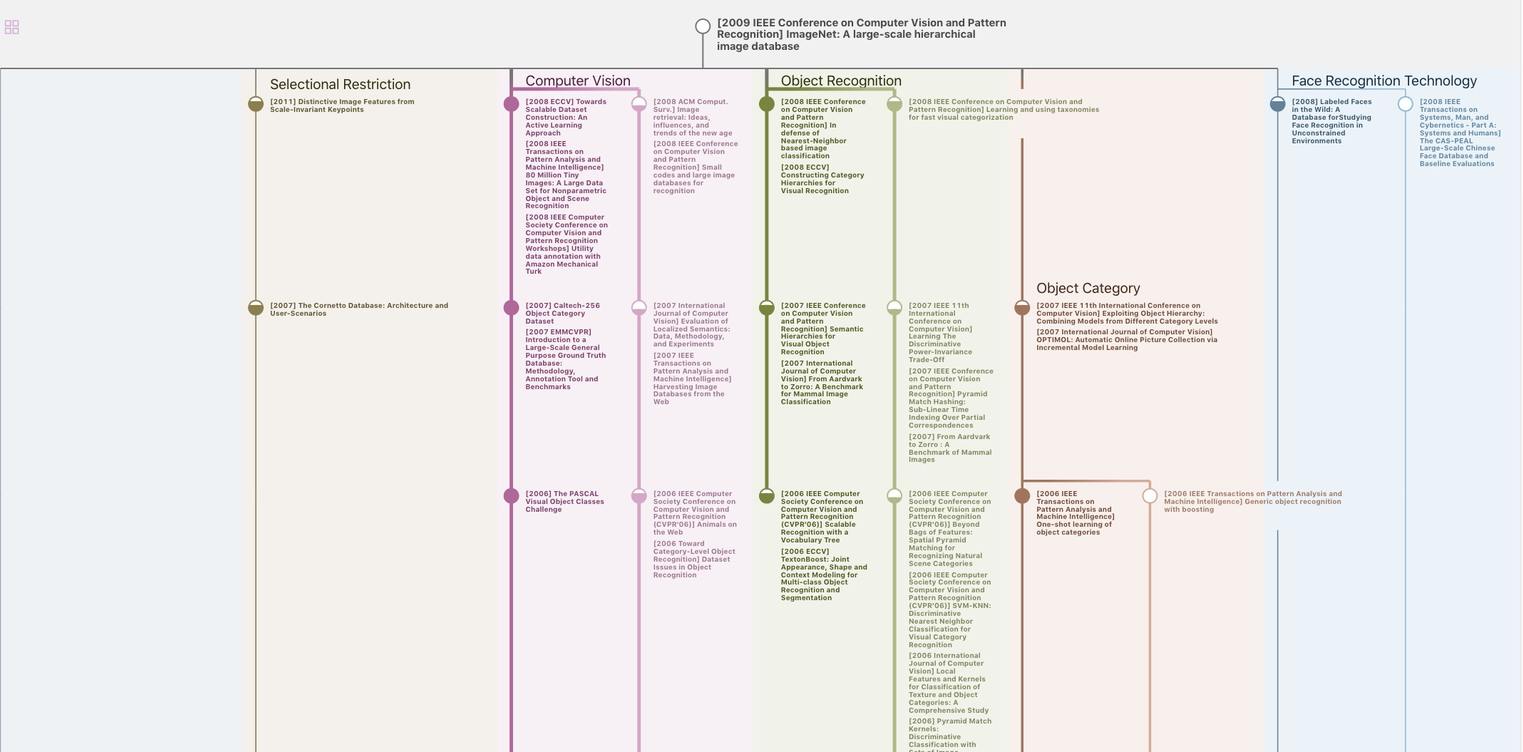
生成溯源树,研究论文发展脉络
数据免责声明
页面数据均来自互联网公开来源、合作出版商和通过AI技术自动分析结果,我们不对页面数据的有效性、准确性、正确性、可靠性、完整性和及时性做出任何承诺和保证。若有疑问,可以通过电子邮件方式联系我们:report@aminer.cn