Feature-domain Adaptive Contrastive Distillation for Efficient Single Image Super-Resolution
IEEE ACCESS(2023)
摘要
Convolutional neural network-based single image super-resolution (SISR) involves numerous parameters and high computational expenses to ensure improved performance, limiting its applicability in resource-constrained devices such as mobile phones. Knowledge distillation (KD), which transfers useful knowledge from a teacher network to a student network, has been investigated as a method to make networks more efficient in terms of performance. To this end, feature distillation (FD) has been utilized in KD to minimize the Euclidean distance-based loss of feature maps between teacher and student networks. However, this technique does not adequately consider the effective and meaningful delivery of knowledge from the teacher to the student network to improve the latter’s performance under given network capacity constraints. In this study, we propose a feature-domain adaptive contrastive distillation (FACD) method to train lightweight student SISR networks efficiently. We highlight the limitations of existing FD methods in terms of Euclidean distance-based loss, and propose a feature-domain contrastive loss, which causes student networks to learn richer information from the teacher’s representation in the feature domain. We also implement adaptive distillation that performs distillation selectively depending on the conditions of the training patches. Experimental results demonstrated that the proposed FACD scheme improves student enhanced deep residual networks and residual channel attention networks not only in terms of the peak signal-to-noise ratio (PSNR) on all benchmark datasets and scales but also in terms of subjective image quality, compared to the conventional FD approaches. In particular, FACD achieved an average PSNR improvement of 0.07 dB over conventional FD in both networks. Code will be release at https://github.com/hcmoon0613/FACD.
更多查看译文
关键词
Contrastive learning,efficient super-resolution,feature distillation,knowledge distillation,single image super-resolution
AI 理解论文
溯源树
样例
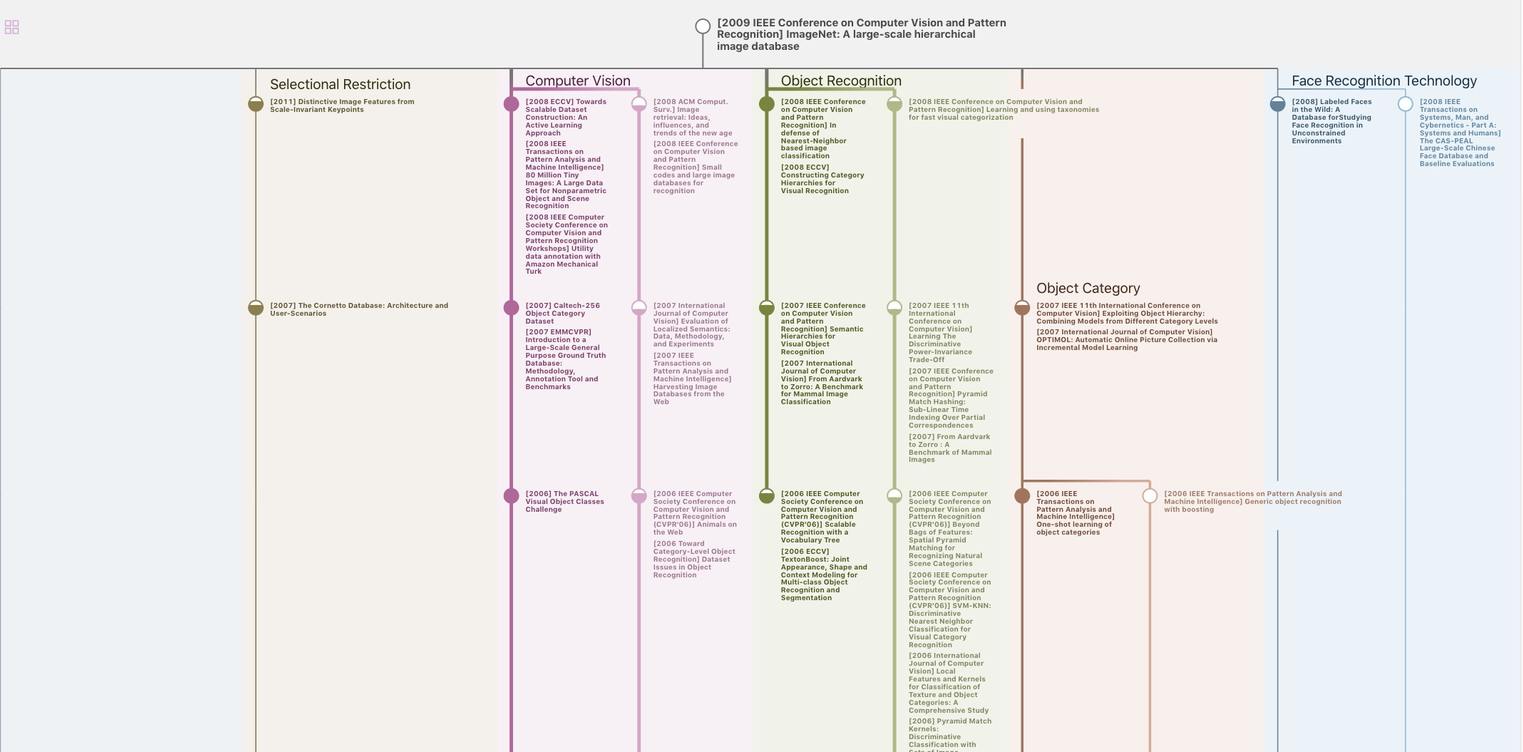
生成溯源树,研究论文发展脉络
数据免责声明
页面数据均来自互联网公开来源、合作出版商和通过AI技术自动分析结果,我们不对页面数据的有效性、准确性、正确性、可靠性、完整性和及时性做出任何承诺和保证。若有疑问,可以通过电子邮件方式联系我们:report@aminer.cn