Strong identifiability and parameter learning in regression with heterogeneous response
arXiv (Cornell University)(2022)
摘要
Mixtures of regression are a powerful class of models for regression learning
with respect to a highly uncertain and heterogeneous response variable of
interest. In addition to being a rich predictive model for the response given
some covariates, the parameters in this model class provide useful information
about the heterogeneity in the data population, which is represented by the
conditional distributions for the response given the covariates associated with
a number of distinct but latent subpopulations. In this paper, we investigate
conditions of strong identifiability, rates of convergence for conditional
density and parameter estimation, and the Bayesian posterior contraction
behavior arising in finite mixture of regression models, under exact-fitted and
over-fitted settings and when the number of components is unknown. This theory
is applicable to common choices of link functions and families of conditional
distributions employed by practitioners. We provide simulation studies and data
illustrations, which shed some light on the parameter learning behavior found
in several popular regression mixture models reported in the literature.
更多查看译文
关键词
strong identifiability,regression,learning
AI 理解论文
溯源树
样例
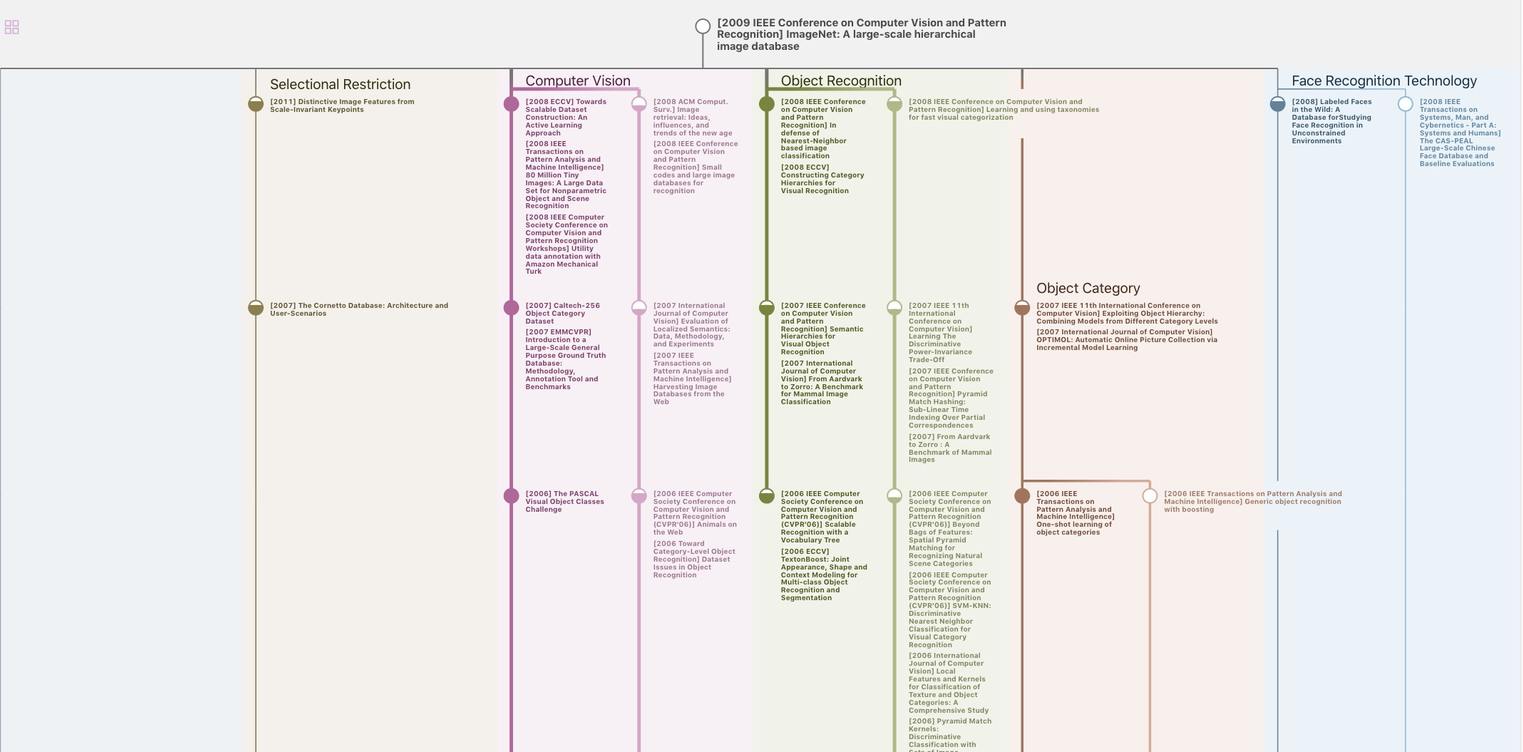
生成溯源树,研究论文发展脉络
数据免责声明
页面数据均来自互联网公开来源、合作出版商和通过AI技术自动分析结果,我们不对页面数据的有效性、准确性、正确性、可靠性、完整性和及时性做出任何承诺和保证。若有疑问,可以通过电子邮件方式联系我们:report@aminer.cn