Re-weighting and Hierarchical Pre-training Boost 3D Medical Self-Supervised Representation.
CCIS(2022)
摘要
The shortage of annotations and class imbalance in three-dimensional (3d) medical volume processing tasks downgrade the performance of supervised deep learning algorithms. We solve this problem via self-supervised learning and the hierarchical pre-training paradigm. This paper aims to improve the discriminability of self-supervised representation extracted by a 3d neural network from class-imbalanced medical volumes and to explore the potential of using deep learning features as complementary knowledge to radiomics features. We propose a self-supervised representation learning framework to extract deep-features from volume patches. Specifically, 1) we design a hyperparameter-free sample re-weighting module, which can embed into existing self-supervised contrastive learning architecture, and 2) we introduce the hierarchical pre-training paradigm into volume modality to improve the data utilization efficiency on limited samples. We conduct detailed experiments on public datasets of two modalities, computerized tomography (CT) and magnetic resonance imaging (MRI), to demonstrate the advantages of our architecture over the state-of-the-art counterpart in terms of feature discriminability.
更多查看译文
关键词
Contrastive learning,Class imbalance,Distribution estimation,Hierarchical pre-training
AI 理解论文
溯源树
样例
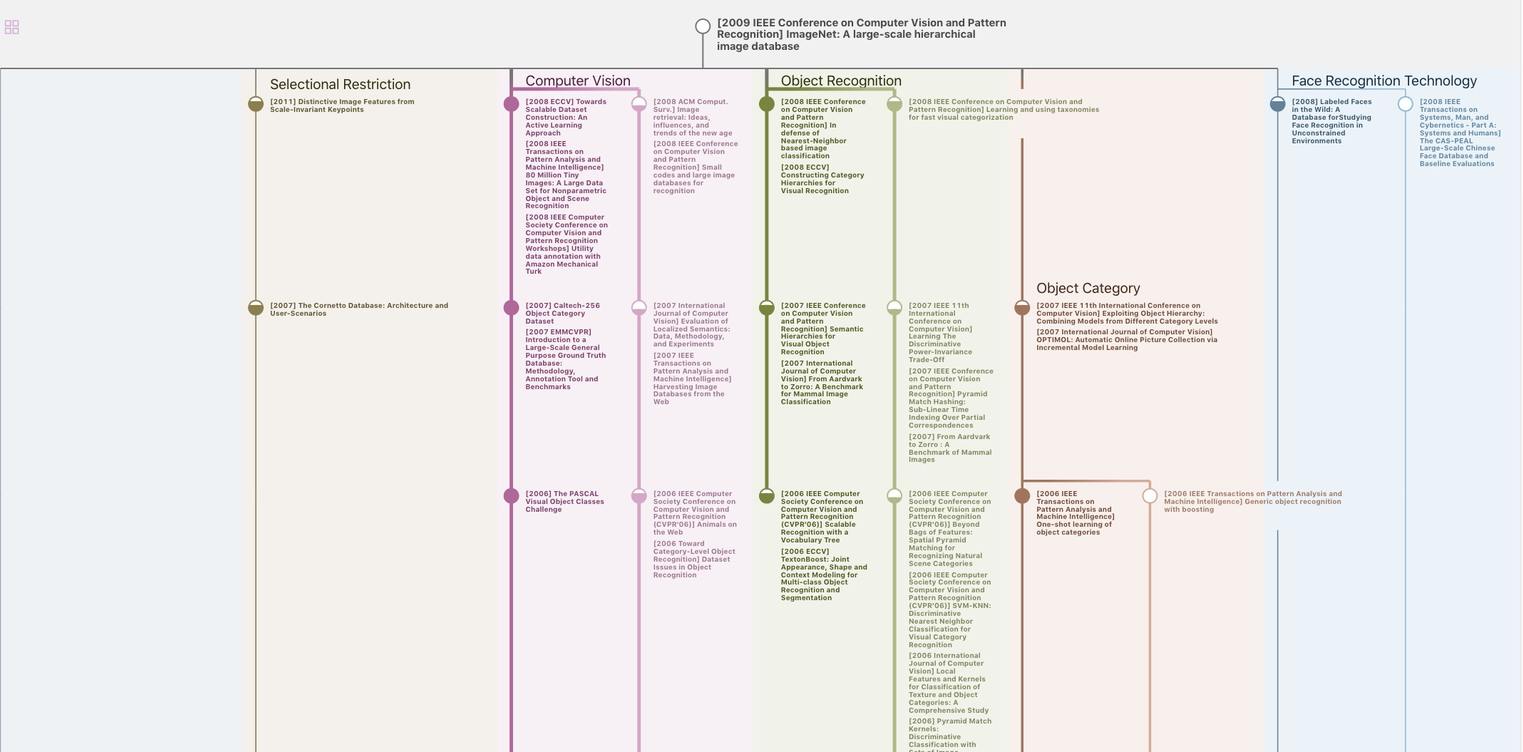
生成溯源树,研究论文发展脉络
数据免责声明
页面数据均来自互联网公开来源、合作出版商和通过AI技术自动分析结果,我们不对页面数据的有效性、准确性、正确性、可靠性、完整性和及时性做出任何承诺和保证。若有疑问,可以通过电子邮件方式联系我们:report@aminer.cn