Can Persistent Homology Provide an Efficient Alternative for Evaluation of Knowledge Graph Completion Methods?
WWW 2023(2023)
摘要
In this paper we present a novel method, Knowledge Persistence (), for faster evaluation of Knowledge Graph (KG) completion approaches. Current ranking-based evaluation is quadratic in the size of the KG, leading to long evaluation times and consequently a high carbon footprint. addresses this by representing the topology of the KG completion methods through the lens of topological data analysis, concretely using persistent homology. The characteristics of persistent homology allow to evaluate the quality of the KG completion looking only at a fraction of the data. Experimental results on standard datasets show that the proposed metric is highly correlated with ranking metrics (Hits@N, MR, MRR). Performance evaluation shows that is computationally efficient: In some cases, the evaluation time (validation+test) of a KG completion method has been reduced from 18 hours (using Hits@10) to 27 seconds (using ), and on average (across methods & data) reduces the evaluation time (validation+test) by ≈ 99.96%.
更多查看译文
关键词
Persistent Homology,Knowledge Graph Embedding,Shape Analysis,Signal Processing on Graphs,Topological Methods
AI 理解论文
溯源树
样例
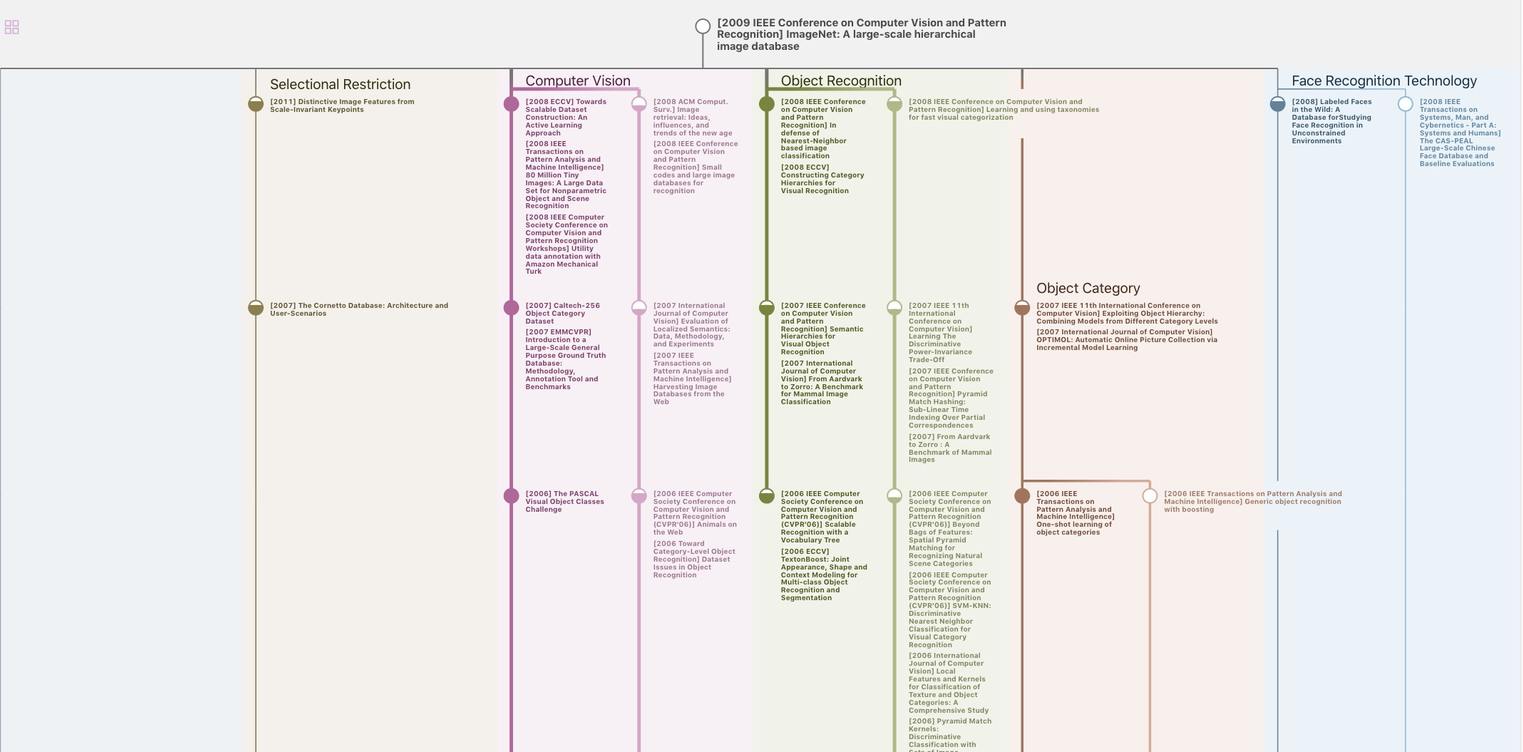
生成溯源树,研究论文发展脉络
数据免责声明
页面数据均来自互联网公开来源、合作出版商和通过AI技术自动分析结果,我们不对页面数据的有效性、准确性、正确性、可靠性、完整性和及时性做出任何承诺和保证。若有疑问,可以通过电子邮件方式联系我们:report@aminer.cn