CIFF-Net: Contextual Image Feature Fusion for Melanoma Diagnosis
BIOMEDICAL SIGNAL PROCESSING AND CONTROL(2024)
摘要
Melanoma is considered to be the deadliest variant of skin cancer causing around 75% of total skin cancer deaths. To diagnose Melanoma, clinicians assess and compare multiple skin lesions of the same patient concurrently to gather contextual information regarding the patterns, and abnormality of the skin. So far this concurrent multi-image comparative method has not been explored by existing deep learning-based schemes. In response to this gap, a contextual image feature fusion (CIFF) based deep neural network (CIFF-Net) is proposed, which integrates patient-level contextual information into the traditional approaches for improved Melanoma diagnosis by concurrent multi-image comparative method. The proposed multi-kernel self attention (MKSA) module offers better generalization of the extracted features by introducing multi-kernel operations in the self attention mechanisms. To utilize both self attention and contextual feature-wise attention, an attention guided module named contextual feature fusion (CFF) is proposed that integrates extracted features from different contextual images into a single feature vector. Finally, in comparative contextual feature fusion (CCFF) module, primary and all contextual features are compared concurrently to generate comparative features mirroring the holistic assessment performed by clinicians. Significant improvement in performance has been achieved on the ISIC-2020 dataset over the traditional approaches with scores of 95.7% AUC and 98.3% Accuracy that validate the effectiveness of the proposed contextual learning scheme.
更多查看译文
关键词
Melanoma diagnosis,Skin lesion recognition,Clinical-inspired,Dermoscopy images,Deep learning
AI 理解论文
溯源树
样例
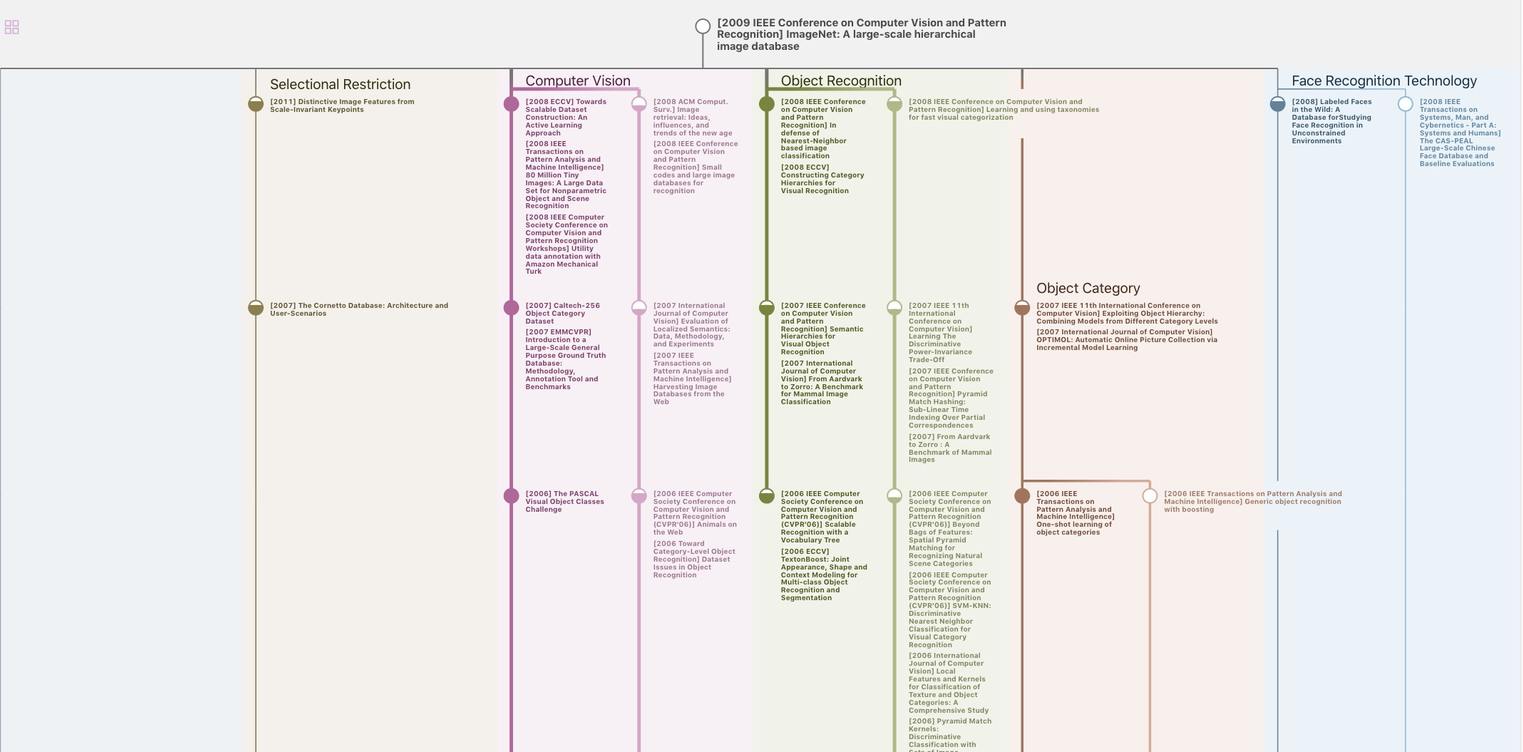
生成溯源树,研究论文发展脉络
数据免责声明
页面数据均来自互联网公开来源、合作出版商和通过AI技术自动分析结果,我们不对页面数据的有效性、准确性、正确性、可靠性、完整性和及时性做出任何承诺和保证。若有疑问,可以通过电子邮件方式联系我们:report@aminer.cn