Pathologist Validation of a Machine Learning–Derived Feature for Colon Cancer Risk Stratification
JAMA NETWORK OPEN(2023)
摘要
ImportanceIdentifying new prognostic features in colon cancer has the potential to refine histopathologic review and inform patient care. Although prognostic artificial intelligence systems have recently demonstrated significant risk stratification for several cancer types, studies have not yet shown that the machine learning-derived features associated with these prognostic artificial intelligence systems are both interpretable and usable by pathologists. ObjectiveTo evaluate whether pathologist scoring of a histopathologic feature previously identified by machine learning is associated with survival among patients with colon cancer. Design, Setting, and ParticipantsThis prognostic study used deidentified, archived colorectal cancer cases from January 2013 to December 2015 from the University of Milano-Bicocca. All available histologic slides from 258 consecutive colon adenocarcinoma cases were reviewed from December 2021 to February 2022 by 2 pathologists, who conducted semiquantitative scoring for tumor adipose feature (TAF), which was previously identified via a prognostic deep learning model developed with an independent colorectal cancer cohort. Main Outcomes and MeasuresPrognostic value of TAF for overall survival and disease-specific survival as measured by univariable and multivariable regression analyses. Interpathologist agreement in TAF scoring was also evaluated. ResultsA total of 258 colon adenocarcinoma histopathologic cases from 258 patients (138 men [53%]; median age, 67 years [IQR, 65-81 years]) with stage II (n=119) or stage III (n=139) cancer were included. Tumor adipose feature was identified in 120 cases (widespread in63 cases, multifocal in 31, and unifocal in26). For overall survival analysis after adjustment for tumor stage, TAF was independently prognostic in 2 ways: TAF as a binary feature (presence vs absence: hazard ratio [HR] for presence of TAF, 1.55 [95% CI, 1.07-2.25]; P=.02) and TAF as a semiquantitative categorical feature (HR for widespread TAF, 1.87 [95% CI, 1.23-2.85]; P=.004). Interpathologist agreement for widespread TAF vs lower categories (absent, unifocal, or multifocal) was 90%, corresponding to a kappa metric at this threshold of 0.69 (95% CI, 0.58-0.80). Conclusions and RelevanceIn this prognostic study, pathologists were able to learn and reproducibly score for TAF, providing significant risk stratification on this independent data set. Although additional work is warranted to understand the biological significance of this feature and to establish broadly reproducible TAF scoring, this work represents the first validation to date of human expert learning from machine learning in pathology. Specifically, this validation demonstrates that a computationally identified histologic feature can represent a human-identifiable, prognostic feature with the potential for integration into pathology practice.
更多查看译文
关键词
Cancer Imaging,Feature Extraction,Predictive Modeling,Machine Learning,Tumor Heterogeneity
AI 理解论文
溯源树
样例
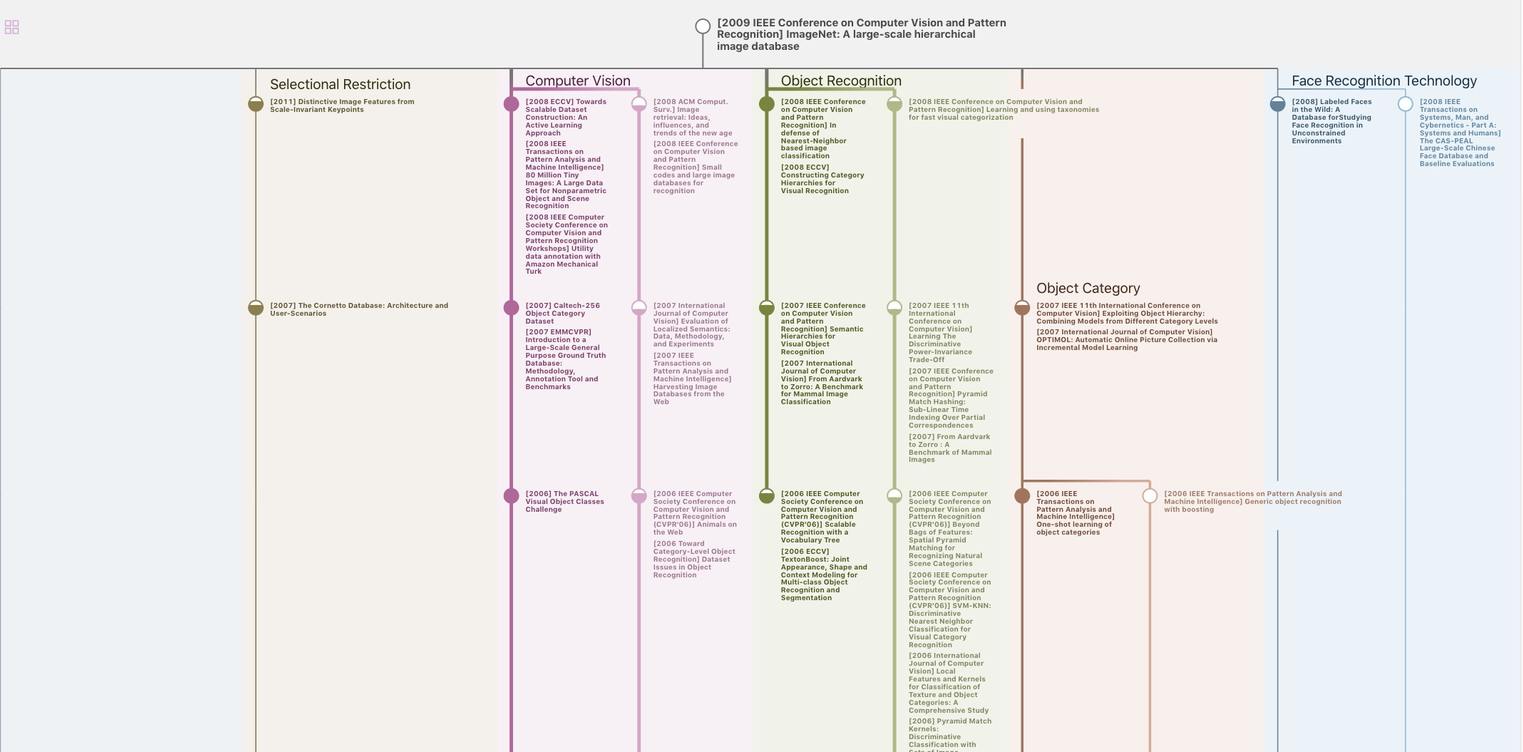
生成溯源树,研究论文发展脉络
数据免责声明
页面数据均来自互联网公开来源、合作出版商和通过AI技术自动分析结果,我们不对页面数据的有效性、准确性、正确性、可靠性、完整性和及时性做出任何承诺和保证。若有疑问,可以通过电子邮件方式联系我们:report@aminer.cn