HNeRV: A Hybrid Neural Representation for Videos.
2023 IEEE/CVF CONFERENCE ON COMPUTER VISION AND PATTERN RECOGNITION (CVPR)(2023)
摘要
Implicit neural representations store videos as neural networks and have performed well for various vision tasks such as video compression and denoising. With frame index or positional index as input, implicit representations (NeRV, E-NeRV, etc.) reconstruct video frames from fixed and content-agnostic embeddings. Such embedding largely limits the regression capacity and internal generalization for video interpolation. In this paper, we propose a Hybrid Neural Representation for Videos (HNeRV), where a learnable encoder generates content-adaptive embeddings, which act as the decoder input. Besides the input embedding, we introduce HNeRV blocks, which ensure model parameters are evenly distributed across the entire network, such that higher layers (layers near the output) can have more capacity to store high-resolution content and video details. With content-adaptive embeddings and redesigned architecture, HNeRV outperforms implicit methods in video regression tasks for both reconstruction quality (+4.7 PSNR) and convergence speed (16 × faster), and shows better internal generalization. As a simple and efficient video representation, HNeRV also shows decoding advantages for speed, flexibility, and deployment, compared to traditional codecs (H.264, H.265) and learning-based compression methods. Finally, we explore the effectiveness of HNeRV on downstream tasks such as video compression and video inpainting.
更多查看译文
关键词
Deep learning architectures and techniques
AI 理解论文
溯源树
样例
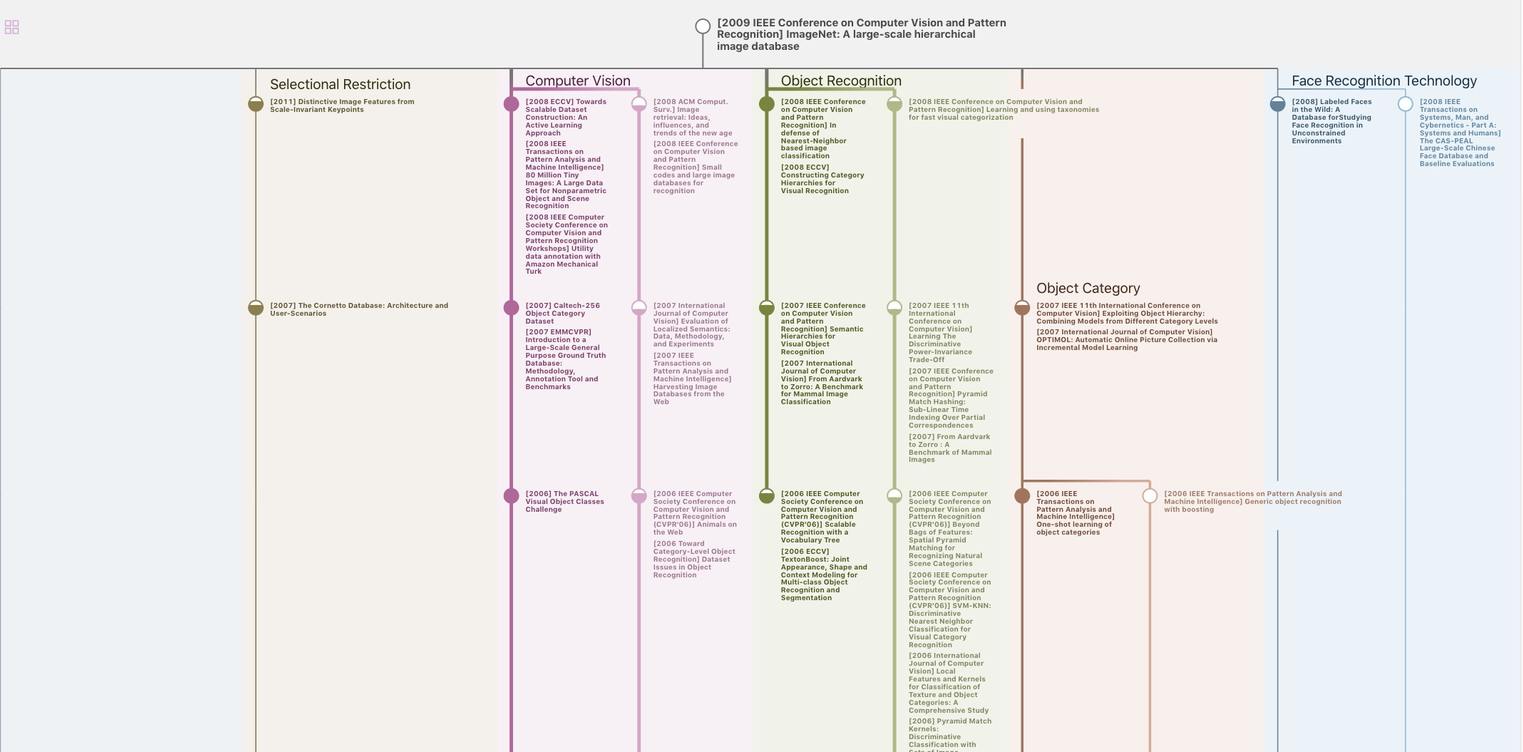
生成溯源树,研究论文发展脉络
数据免责声明
页面数据均来自互联网公开来源、合作出版商和通过AI技术自动分析结果,我们不对页面数据的有效性、准确性、正确性、可靠性、完整性和及时性做出任何承诺和保证。若有疑问,可以通过电子邮件方式联系我们:report@aminer.cn