CKBP V2: Better Annotation and Reasoning for Commonsense Knowledge Base Population
arxiv(2023)
摘要
Commonsense Knowledge Bases (CSKB) Population, which aims at automatically expanding knowledge in CSKBs with external resources, is an important yet hard task in NLP. Fang et al. (2021a) proposed a CSKB Population (CKBP) framework with an evaluation set CKBP v1. However, CKBP v1 relies on crowdsourced annotations that suffer from a considerable number of mislabeled answers, and the evaluationset lacks alignment with the external knowledge source due to random sampling. In this paper, we introduce CKBP v2, a new high-quality CSKB Population evaluation set that addresses the two aforementioned issues by employing domain experts as annotators and incorporating diversified adversarial samples to make the evaluation data more representative. We show that CKBP v2 serves as a challenging and representative evaluation dataset for the CSKB Population task, while its development set aids in selecting a population model that leads to improved knowledge acquisition for downstream commonsense reasoning. A better population model can also help acquire more informative commonsense knowledge as additional supervision signals for both generative commonsense inference and zero-shot commonsense question answering. Specifically, the question-answering model based on DeBERTa-v3-large (He et al., 2023b) even outperforms powerful large language models in a zero-shot setting, including ChatGPT and GPT-3.5.
更多查看译文
AI 理解论文
溯源树
样例
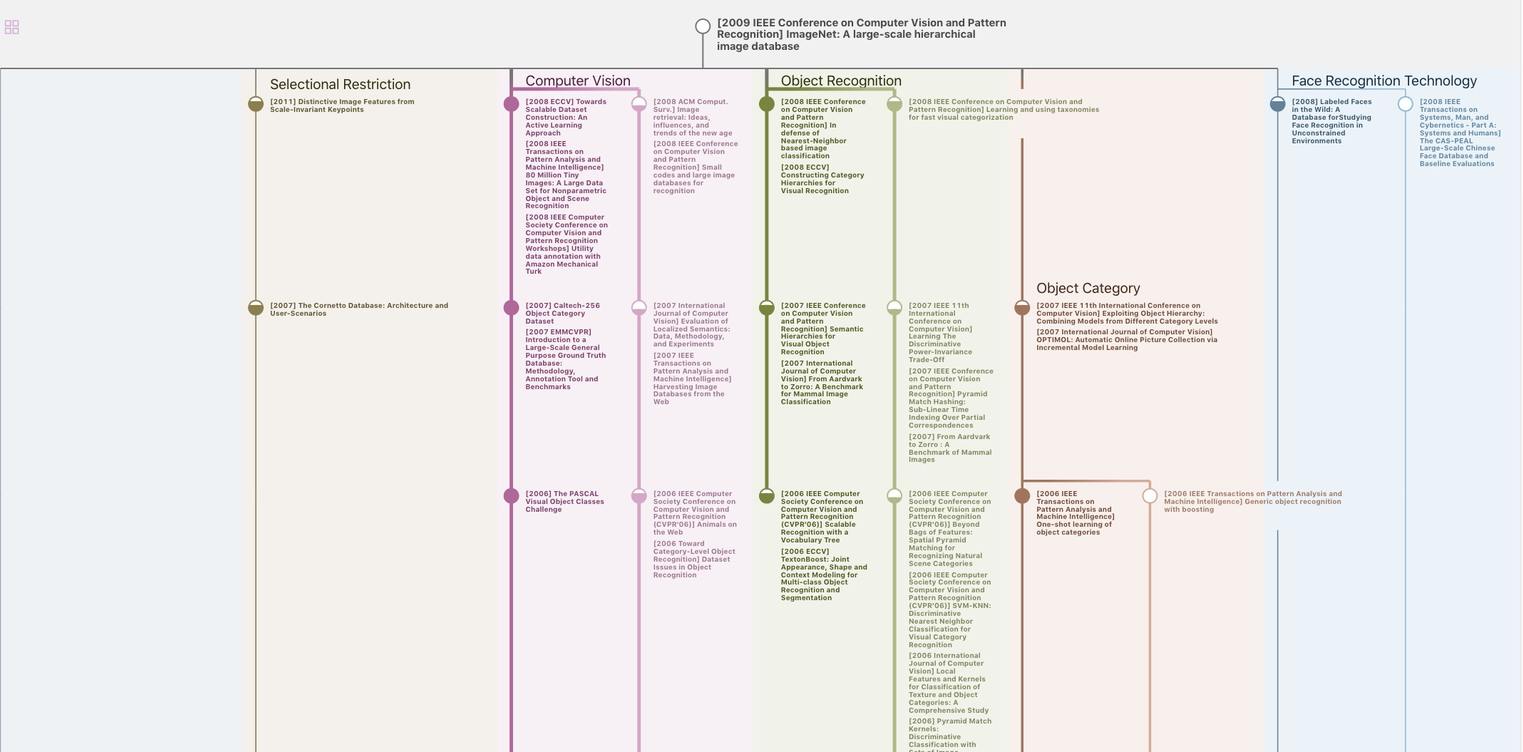
生成溯源树,研究论文发展脉络
数据免责声明
页面数据均来自互联网公开来源、合作出版商和通过AI技术自动分析结果,我们不对页面数据的有效性、准确性、正确性、可靠性、完整性和及时性做出任何承诺和保证。若有疑问,可以通过电子邮件方式联系我们:report@aminer.cn