End-to-End Spatio-Temporal Action Localisation with Video Transformers
Computer Vision and Pattern Recognition(2024)
摘要
The most performant spatio-temporal action localisation models use external person proposals and complex external memory banks. We propose a fully end-to-end, purely-transformer based model that directly ingests an input video, and outputs tubelets – a sequence of bounding boxes and the action classes at each frame. Our flexible model can be trained with either sparse bounding-box supervision on individual frames, or full tubelet annotations. And in both cases, it predicts coherent tubelets as the output. Moreover, our end-to-end model requires no additional pre-processing in the form of proposals, or post-processing in terms of non-maximal suppression. We perform extensive ablation experiments, and significantly advance the state-of-the-art results on four different spatio-temporal action localisation benchmarks with both sparse keyframes and full tubelet annotations.
更多查看译文
关键词
spatio-temporal action localisation,video
AI 理解论文
溯源树
样例
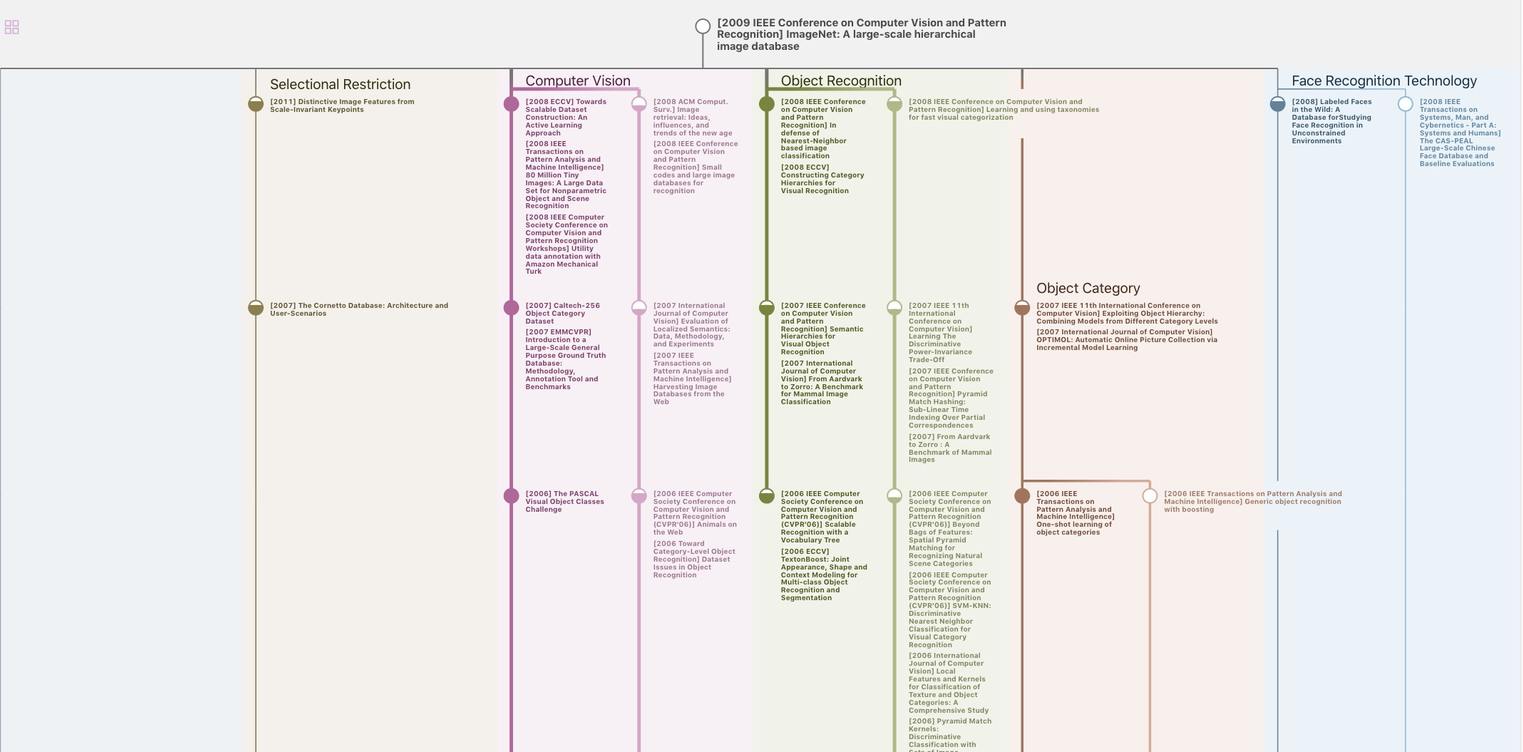
生成溯源树,研究论文发展脉络
数据免责声明
页面数据均来自互联网公开来源、合作出版商和通过AI技术自动分析结果,我们不对页面数据的有效性、准确性、正确性、可靠性、完整性和及时性做出任何承诺和保证。若有疑问,可以通过电子邮件方式联系我们:report@aminer.cn