User-Context Collaboration and Tensor Factorization for GNN-Based Social Recommendation
IEEE TRANSACTIONS ON NETWORK SCIENCE AND ENGINEERING(2023)
摘要
One goal of social recommendation is to utilize social information to alleviate data sparsity and improve recommendation accuracy. User social relationships are inherently graph-structured, graph neural network (GNN) has recently attracted extensive attention in social recommendation because of its capability to integrate structural information and topology. However, current graph neural network (GNN)-based social recommendation models fail to consider context information during user interactions, which hinders more accurate modeling of user interest features. To address this problem, we propose a new social recommendation model based on context-aware graph neural network named CENTRIC (User- C ont E xt Collaboratio N and T enso R Factorization for GNN-based Soc I al Re C ommendation). Specifically, first a multi-channel GNN model with user-context collaboration module is designed, so that context can directly affect user features and participate in the calculation of user interaction probability with items. Then tensor factorization is adopted in output layer to effectively fuse the features extracted from different channels. Experiments on three public datasets show that CENTRIC significantly outperforms other state-of-the-art social recommendation models, further experiments also demonstrate that context information and tensor factorization help improve the accuracy of GNN-based social recommendation.
更多查看译文
关键词
Context modeling,Tensors,Collaboration,Graph neural networks,Data models,Social networking (online),Feature extraction,Recommender systems,Social recommendation,context-aware,graph neural network,tensor factorization
AI 理解论文
溯源树
样例
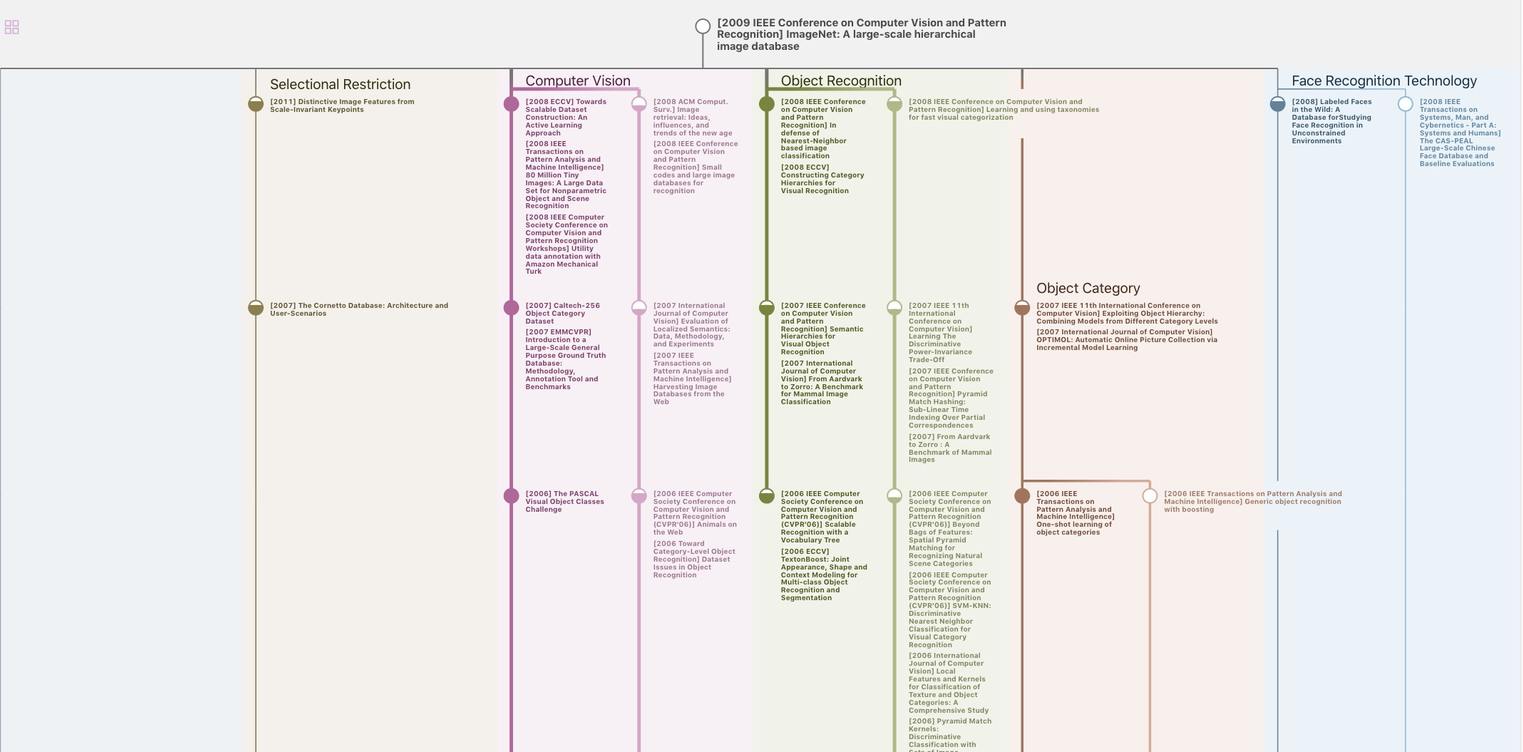
生成溯源树,研究论文发展脉络
数据免责声明
页面数据均来自互联网公开来源、合作出版商和通过AI技术自动分析结果,我们不对页面数据的有效性、准确性、正确性、可靠性、完整性和及时性做出任何承诺和保证。若有疑问,可以通过电子邮件方式联系我们:report@aminer.cn