Nonlinear System Identification of Tremors Dynamics: A Data-driven Approximation Using Koopman Operator Theory.
2023 11TH INTERNATIONAL IEEE/EMBS CONFERENCE ON NEURAL ENGINEERING, NER(2023)
摘要
People who suffer from tremors have difficulty performing activities of daily living. Efforts in developing a model of a limb with tremors can pave the way for non-surgical tremor suppression techniques. However, due to the nonlinearity, developing an accurate model of tremors is challenging. This paper implements a data-driven method for approximating the Koopman operator, which is capable of presenting nonlinear dynamics in a linear framework and is promising for predicting the nonlinear system. A dynamic model of tremors is developed with ultrasound (US) image data collected from a patient with essential tremor as they grasp objects. The method is applied to predict the patient's tremor dynamics and is compared with the nonlinear Hammerstein-Wiener system identification technique.
更多查看译文
关键词
data-driven approximation,data-driven method,dynamic model,essential tremor,image data,Koopman operator theory,nonlinear dynamics,nonlinear Hammerstein-Wiener system identification technique,nonlinear system identification,nonlinearity,nonsurgical tremor suppression techniques,patient,tremors dynamics,ultrasound image data,US
AI 理解论文
溯源树
样例
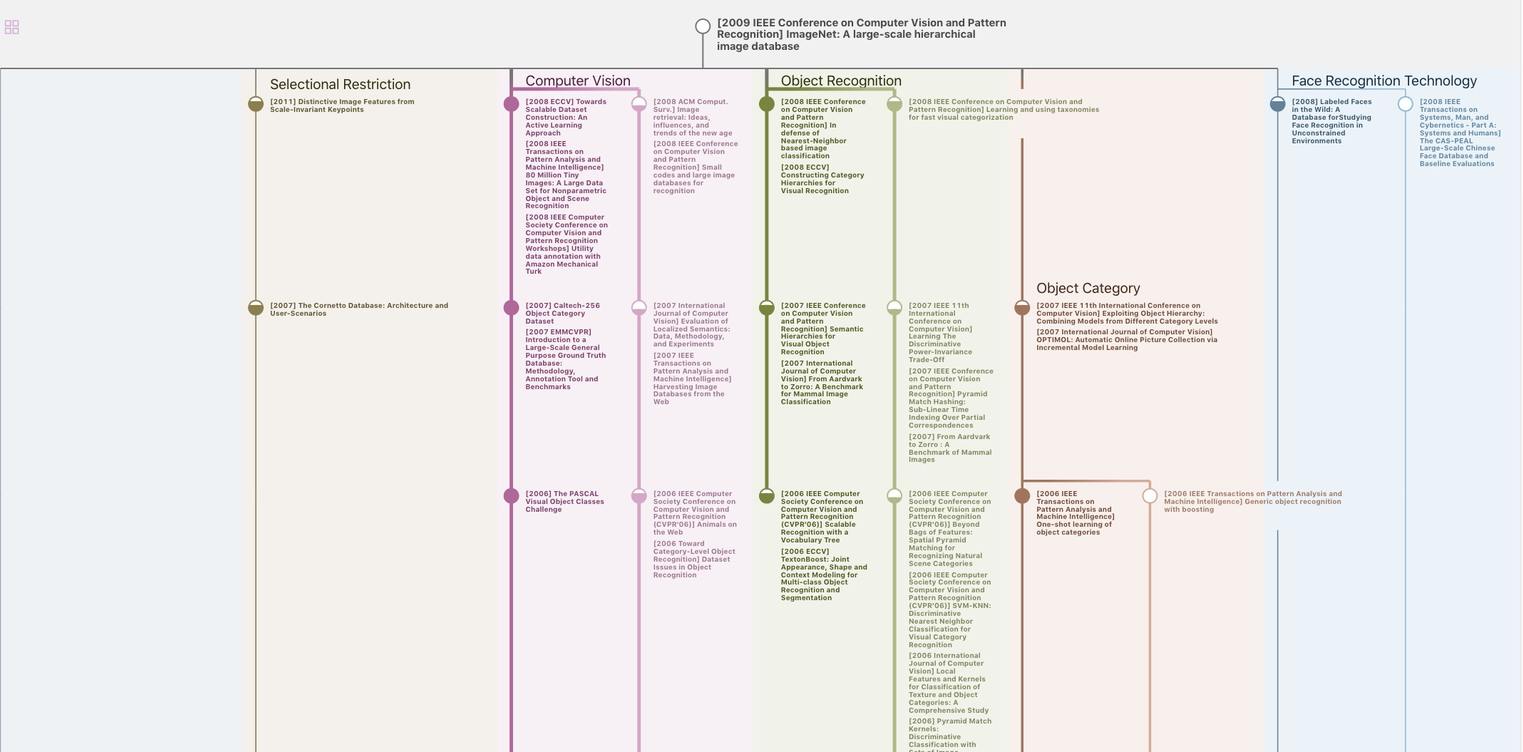
生成溯源树,研究论文发展脉络
数据免责声明
页面数据均来自互联网公开来源、合作出版商和通过AI技术自动分析结果,我们不对页面数据的有效性、准确性、正确性、可靠性、完整性和及时性做出任何承诺和保证。若有疑问,可以通过电子邮件方式联系我们:report@aminer.cn