Process Voltage Temperature Variability Estimation of Tunneling Current for Band-to-Band-Tunneling Based Neuron
IEEE TRANSACTIONS ON ELECTRON DEVICES(2024)
摘要
Compact and energy-efficient synapse and neurons are essential to realize the full potential of neuromorphic computing. In addition, a low variability is indeed needed for neurons in deep neural networks for higher accuracy. Further, process (P), voltage (V), and temperature (T) (PVT) variation are essential considerations for low-power circuits as performance impact and compensation complexities are added costs. Recently, band-to-band tunneling (BTBT) neuron has been demonstrated to operate successfully in a network to enable a liquid state machine (LSM). A comparison of the PVT with competing modes of operation (e.g., BTBT versus subthreshold and above threshold) of the same transistor is a critical factor in assessing performance. In this work, we demonstrate the PVT variation impact on the BTBT regime and benchmark the operation against the subthreshold regime (SS) and ON-regime ( $\text{I}_{ \mathrm{\scriptscriptstyle ON}}$ ) of partially depleted silicon-on-insulator MOSFET. It is shown that the ON-state regime offers the lowest variability but dissipates higher power, hence not usable for low-power sources. Among the BTBT and SS regimes, which can enable the low-power neuron, the BTBT regime has shown $\sim 3\,\,{\times }$ variability reduction ( ${ \boldsymbol {\sigma }_{I{_{D}}}{/} \boldsymbol {\mu }_{I{_{D}}}}$ ) compared to the SS regime, considering the cumulative PVT variability. The improvement is due to the well-known weaker P, V, and T dependence of BTBT versus SS. We show that the BTBT variation is uncorrelated with mutually correlated SS and $\text{I}_{{ \mathrm{\scriptscriptstyle ON}}}$ operation—indicating its different origin from the mechanism and location perspectives. Hence, the BTBT regime is promising for low-current, low-power, and low device-to-device (D2D) variability neuron operation.
更多查看译文
关键词
Band-to-band-tunneling (BTBT),neuron,on regime (ION),process variability,silicon-on-insulator (SOI),subthreshold regime (SS),temperature variability,voltage variability
AI 理解论文
溯源树
样例
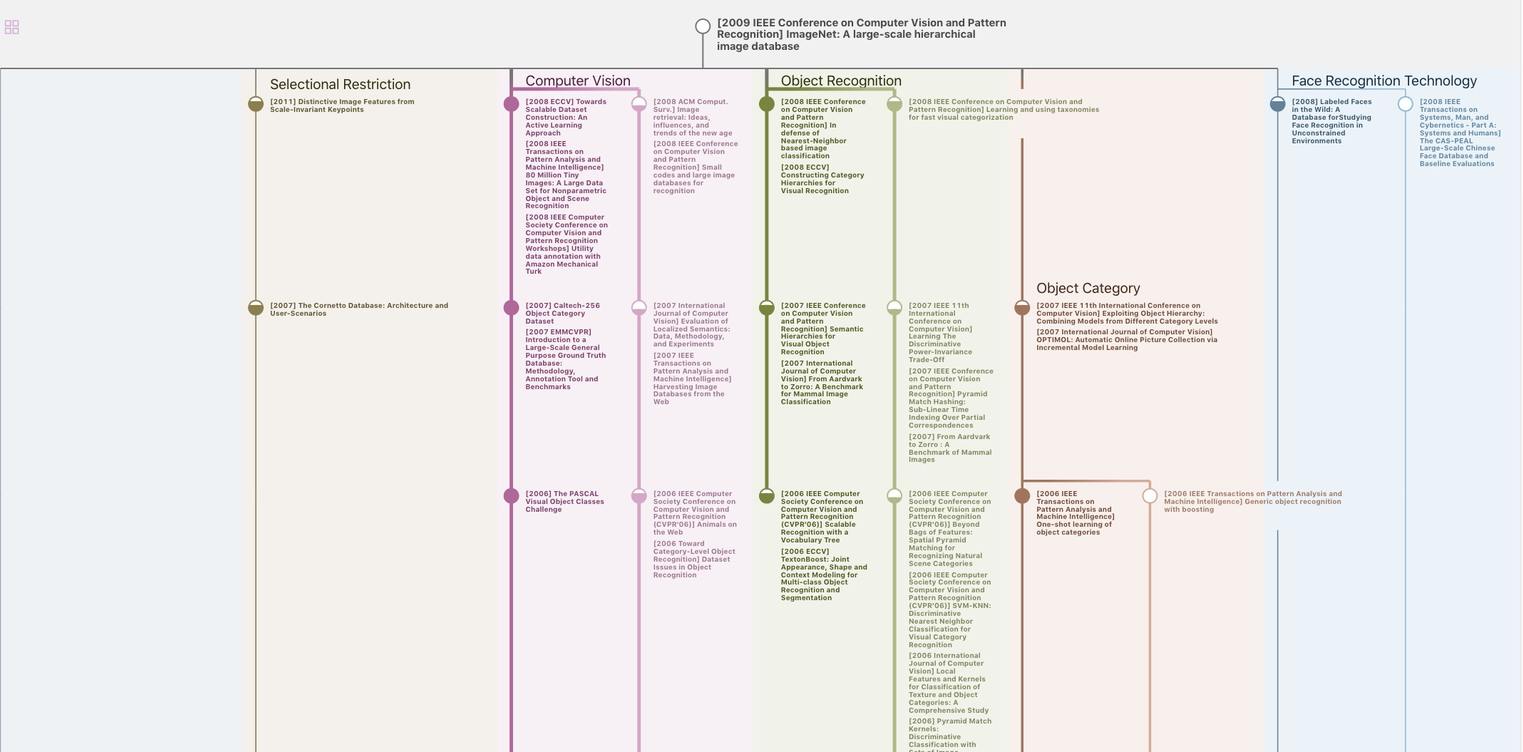
生成溯源树,研究论文发展脉络
数据免责声明
页面数据均来自互联网公开来源、合作出版商和通过AI技术自动分析结果,我们不对页面数据的有效性、准确性、正确性、可靠性、完整性和及时性做出任何承诺和保证。若有疑问,可以通过电子邮件方式联系我们:report@aminer.cn