Object-level Hyperspectral Target Detection Based on Spectral-Spatial Features Integrated YOLOv4-Tiny Network
International Conference on Image, Video and Signal Processing(2022)
摘要
The spectral resolution and spatial resolution of hyperspectral remote sensing images are mutually limited. To keep the same signal-to-noise ratio, the spatial resolution will decrease when the spectral resolution improves. The targets in low-resolution hyperspectral image, such as airplanes, cars and ships, appear as several pixels or sub-pixels. Current hyperspectral target detection methods mainly focus on pixel-level targets, which process spectral information and simple neighbourhood-pixel-related information in a pixel-by-pixel detection strategy. The contribution of spatial features is limited, and it takes a long time to train and detect pixel-by-pixel. Inspired by the deep learning-based object detection technologies for RGB images, we designed a hyperspectral image target detection method based on spectral-spatial features integrated YOLOv4-tiny network (SS-YOLONet). The 3D hyperspectral images were directly sent to the detection network, their spectral information and complex spatial features were extracted by channel attention module, spatial attention module and 3D convolution. Considering the small size of targets such as airplanes, we extracted two shallow features for small-scale objects. In the experiment, we used the pansharpened EO-1 hyperspectral images to verify the effectiveness of the proposed algorithm.
更多查看译文
AI 理解论文
溯源树
样例
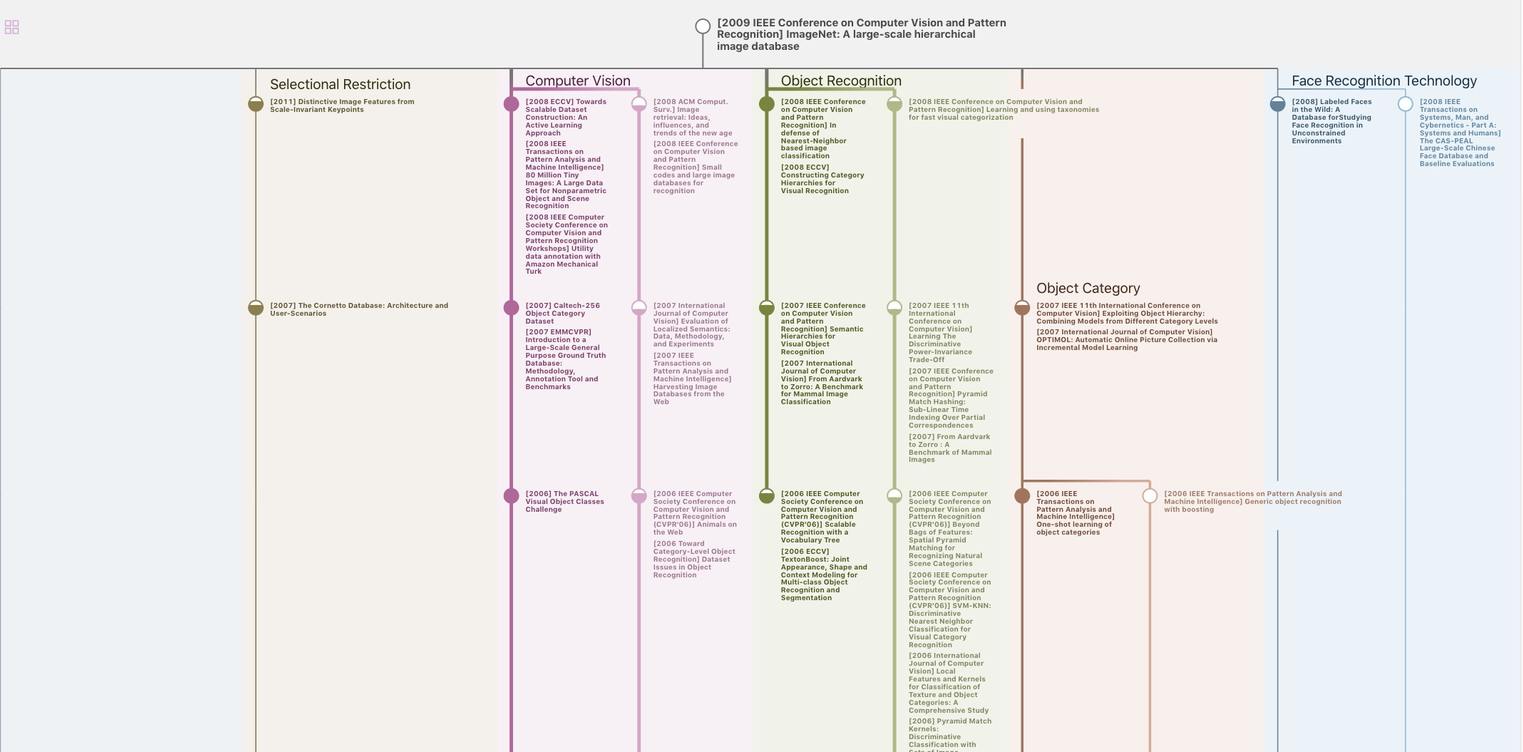
生成溯源树,研究论文发展脉络
数据免责声明
页面数据均来自互联网公开来源、合作出版商和通过AI技术自动分析结果,我们不对页面数据的有效性、准确性、正确性、可靠性、完整性和及时性做出任何承诺和保证。若有疑问,可以通过电子邮件方式联系我们:report@aminer.cn