On the Use of Deep Learning for Phase Recovery
Light, science & applications(2024)
摘要
Phase recovery (PR) refers to calculating the phase of the light field from its intensity measurements. As exemplified from quantitative phase imaging and coherent diffraction imaging to adaptive optics, PR is essential for reconstructing the refractive index distribution or topography of an object and correcting the aberration of an imaging system. In recent years, deep learning (DL), often implemented through deep neural networks, has provided unprecedented support for computational imaging, leading to more efficient solutions for various PR problems. In this review, we first briefly introduce conventional methods for PR. Then, we review how DL provides support for PR from the following three stages, namely, pre-processing, in-processing, and post-processing. We also review how DL is used in phase image processing. Finally, we summarize the work in DL for PR and provide an outlook on how to better use DL to improve the reliability and efficiency of PR. Furthermore, we present a live-updating resource ( https://github.com/kqwang/phase-recovery ) for readers to learn more about PR.
更多查看译文
关键词
Phase Recovery,Neural Network,Phase Contrast,Intensity Measurements,Learning Module,Light Field,Mapping Relationship,Paired Datasets,Diffraction Images,Hologram,Adaptive Optics,Refractive Index Distribution,Quantitative Phase Imaging,Coherent Diffraction,Coherent Diffractive Imaging,Network Training,Image Intensity,Wavefront,Neural Network Training,Light Phase,Inference Time,Peak Signal-to-noise Ratio,Structural Similarity Index Measure,Well-posedness
AI 理解论文
溯源树
样例
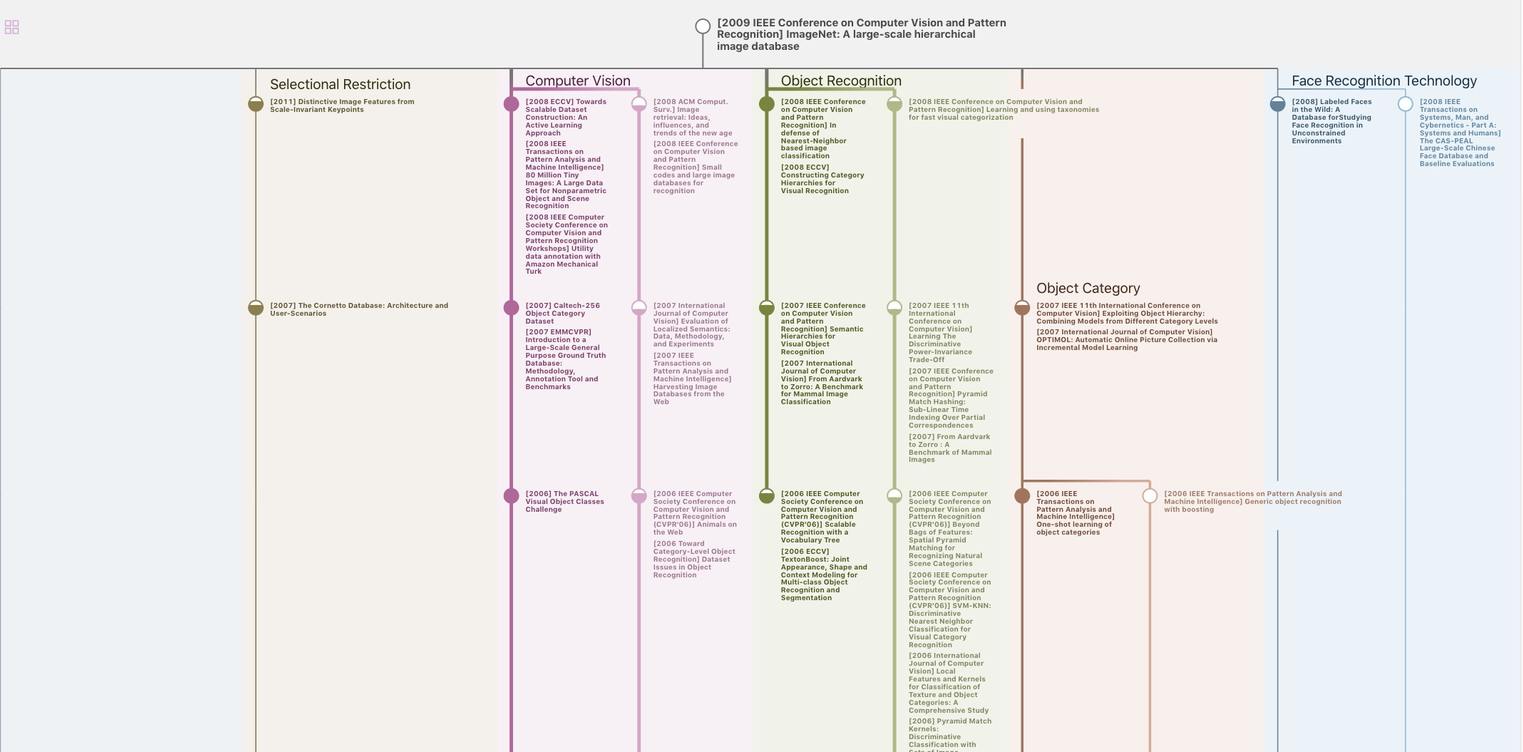
生成溯源树,研究论文发展脉络
数据免责声明
页面数据均来自互联网公开来源、合作出版商和通过AI技术自动分析结果,我们不对页面数据的有效性、准确性、正确性、可靠性、完整性和及时性做出任何承诺和保证。若有疑问,可以通过电子邮件方式联系我们:report@aminer.cn