Lightweight Real-Time Image Super-Resolution Network for 4K Images.
CVPR Workshops(2023)
摘要
Single-image super-resolution technology has become a topic of extensive research in various applications, aiming to enhance the quality and resolution of degraded images obtained from low-resolution sensors. However, most existing studies on single-image super-resolution have primarily focused on developing deep learning networks operating on high-performance graphics processing units. Therefore, this study proposes a lightweight real-time image super-resolution network for 4K images. Furthermore, we applied a reparameterization method to improve the network performance without incurring additional computational costs. The experimental results demonstrate that the proposed network achieves a PSNR of 30.15 dB and an inference time of 4.75 ms on an RTX 3090Ti device, as evaluated on the NTIRE 2023 Real-Time Super-Resolution validation scale X3 dataset. The code is available at https://github.com/Ganzooo/LRSRN.git.
更多查看译文
关键词
4K images,deep learning networks,degraded images,inference time,lightweight real-time image super-resolution network,low-resolution sensors,NTIRE 2023 real-time super-resolution validation scale X3 dataset,PSNR,RTX 3090Ti device,single-image super-resolution technology
AI 理解论文
溯源树
样例
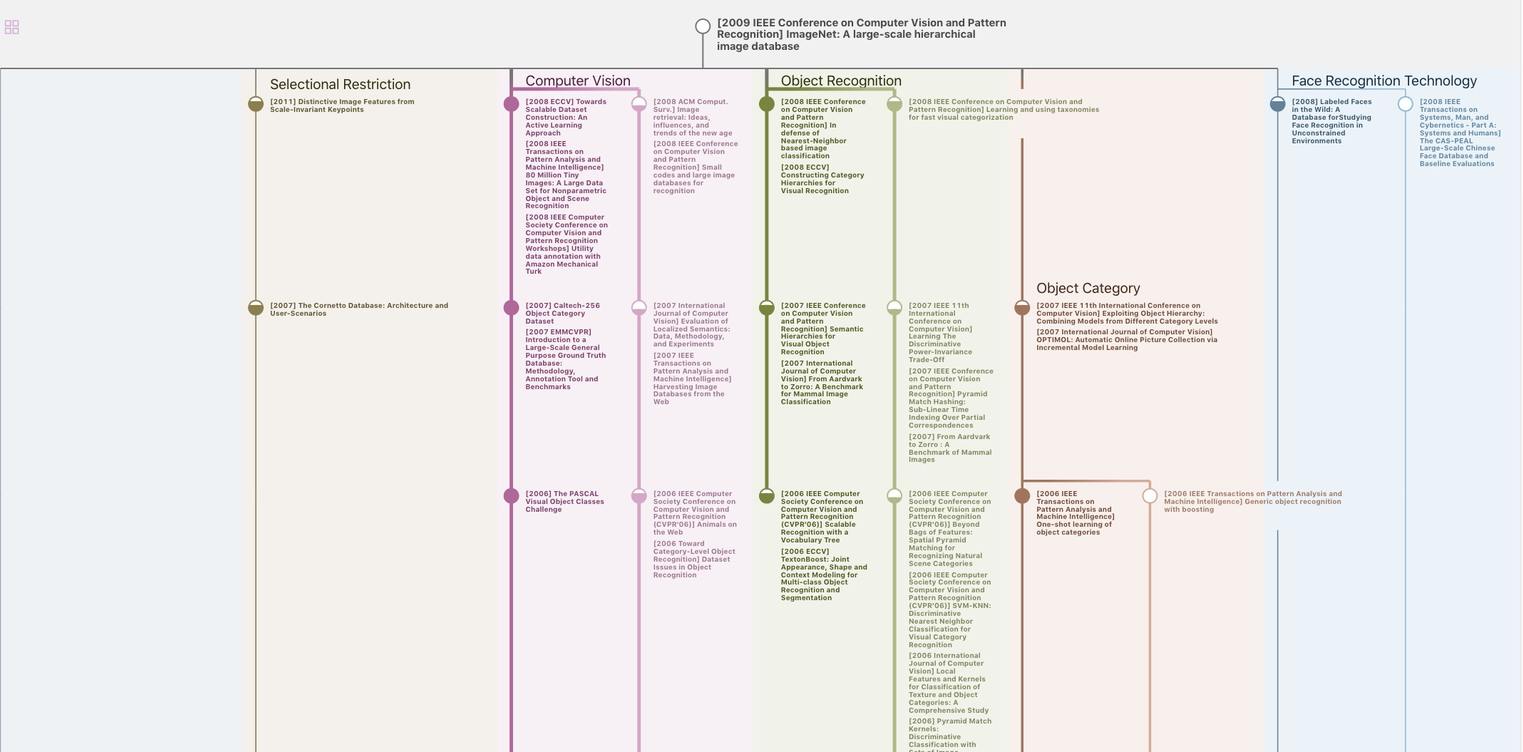
生成溯源树,研究论文发展脉络
数据免责声明
页面数据均来自互联网公开来源、合作出版商和通过AI技术自动分析结果,我们不对页面数据的有效性、准确性、正确性、可靠性、完整性和及时性做出任何承诺和保证。若有疑问,可以通过电子邮件方式联系我们:report@aminer.cn