AdaGL: Adaptive Learning for Agile Distributed Training of Gigantic GNNs
2023 60TH ACM/IEEE DESIGN AUTOMATION CONFERENCE, DAC(2023)
摘要
Distributed GNN training on contemporary massive and densely connected graphs requires information aggregation from all neighboring nodes, which leads to an explosion of inter-server communications. This paper proposes AdaGL, a highly scalable end-to-end framework for rapid distributed GNN training. AdaGL novelty lies upon our adaptive-learning based graph-allocation engine as well as utilizing multi-resolution coarse representation of dense graphs. As a result, AdaGL achieves an unprecedented level of balanced server computation while minimizing the communication overhead. Extensive proof-of-concept evaluations on billion-scale graphs show AdaGL attains ∼30−40% faster convergence compared with prior arts.
更多查看译文
关键词
Distributed Computing,Large-scale Graphs,Signal Processing on Graphs,Graph Matching,Representation Learning
AI 理解论文
溯源树
样例
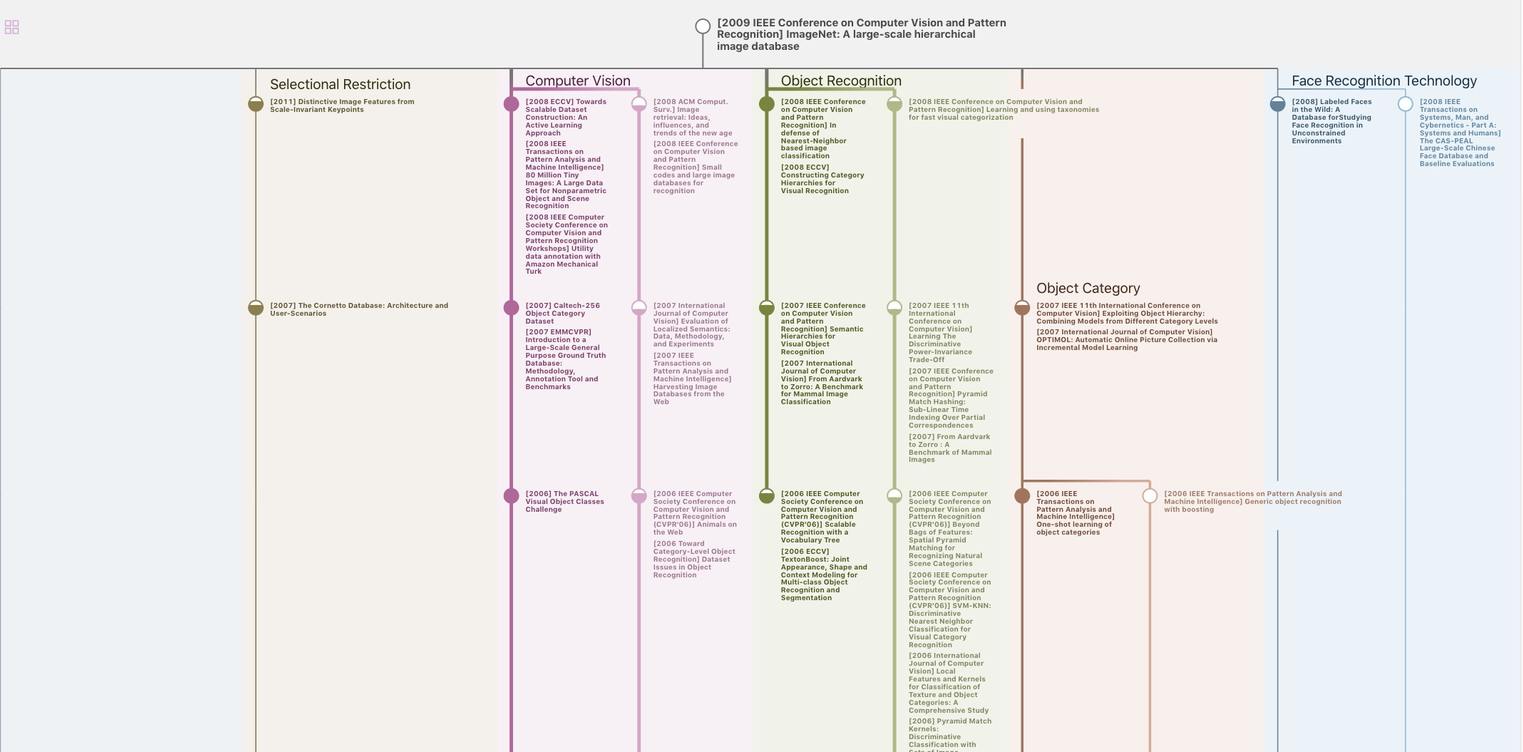
生成溯源树,研究论文发展脉络
数据免责声明
页面数据均来自互联网公开来源、合作出版商和通过AI技术自动分析结果,我们不对页面数据的有效性、准确性、正确性、可靠性、完整性和及时性做出任何承诺和保证。若有疑问,可以通过电子邮件方式联系我们:report@aminer.cn