Folding Attention: Memory and Power Optimization for On-Device Transformer-Based Streaming Speech Recognition.
IEEE International Conference on Acoustics, Speech, and Signal Processing(2024)
摘要
Transformer-based models excel in speech recognition. Existing efforts tooptimize Transformer inference, typically for long-context applications, centeron simplifying attention score calculations. However, streaming speechrecognition models usually process a limited number of tokens each time, makingattention score calculation less of a bottleneck. Instead, the bottleneck liesin the linear projection layers of multi-head attention and feedforwardnetworks, constituting a substantial portion of the model size and contributingsignificantly to computation, memory, and power usage. To address this bottleneck, we propose folding attention, a techniquetargeting these linear layers, significantly reducing model size and improvingmemory and power efficiency. Experiments on on-device Transformer-basedstreaming speech recognition models show that folding attention reduces modelsize (and corresponding memory consumption) by up to 24by up to 23
更多查看译文
AI 理解论文
溯源树
样例
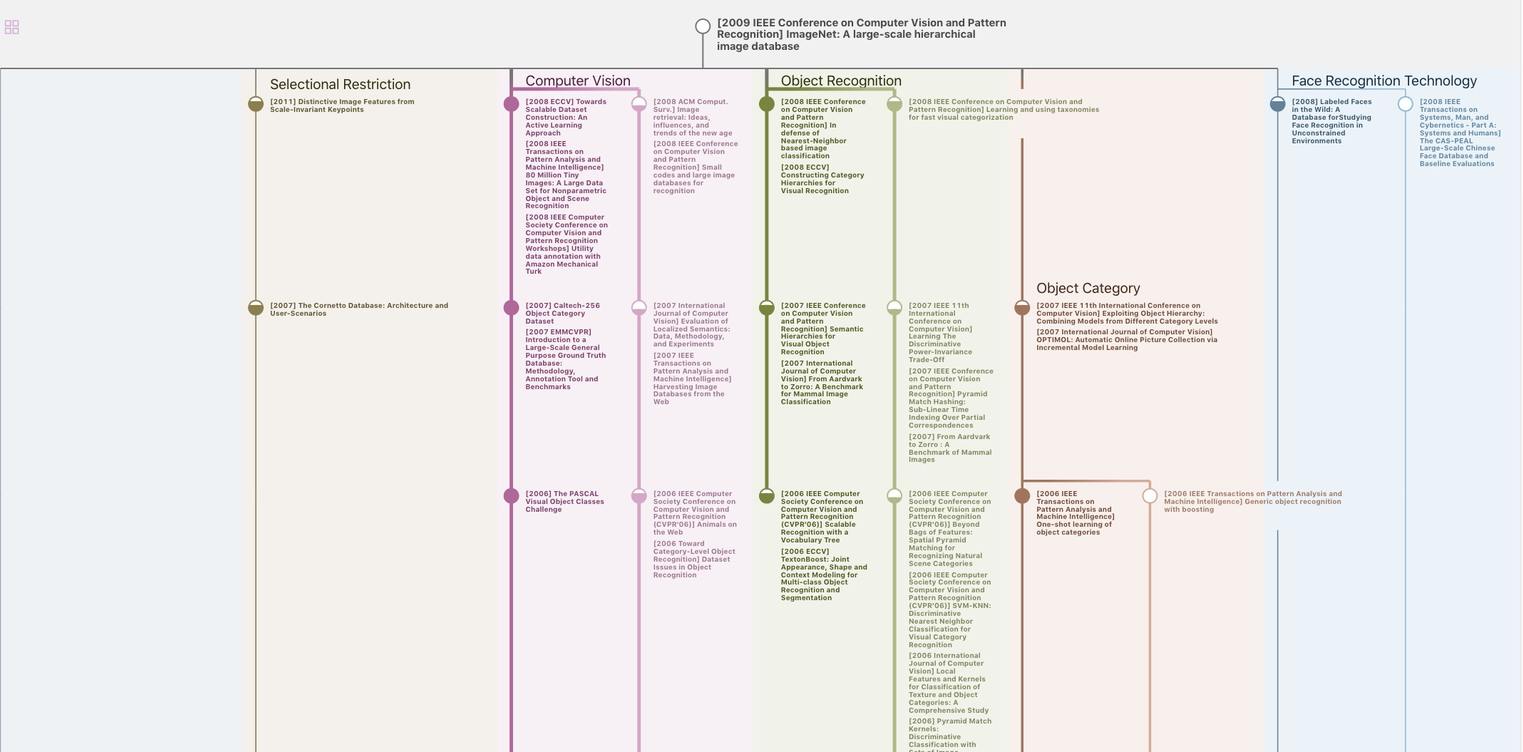
生成溯源树,研究论文发展脉络
数据免责声明
页面数据均来自互联网公开来源、合作出版商和通过AI技术自动分析结果,我们不对页面数据的有效性、准确性、正确性、可靠性、完整性和及时性做出任何承诺和保证。若有疑问,可以通过电子邮件方式联系我们:report@aminer.cn