Automatic Pair Construction for Contrastive Post-training
NAACL-HLT (Findings)(2024)
摘要
Alignment serves as an important step to steer large language models (LLMs)towards human preferences. In this paper, we propose an automatic way toconstruct contrastive data for LLM, using preference pairs from multiple modelsof varying strengths (e.g., InstructGPT, ChatGPT and GPT-4). We compare thecontrastive techniques of SLiC and DPO to SFT baselines and find that DPOprovides a step-function improvement even after continuing SFT saturates. Wealso explore a data curriculum learning scheme for contrastive post-training,which starts by learning from "easier" pairs and transitioning to "harder"ones, which further improves alignment. Finally, we scale up our experiments totrain with more data and larger models like Orca. Remarkably, our automaticcontrastive post-training further improves the performance of Orca, already astate-of-the-art instruction learning model tuned with GPT-4 outputs, tooutperform ChatGPT.
更多查看译文
AI 理解论文
溯源树
样例
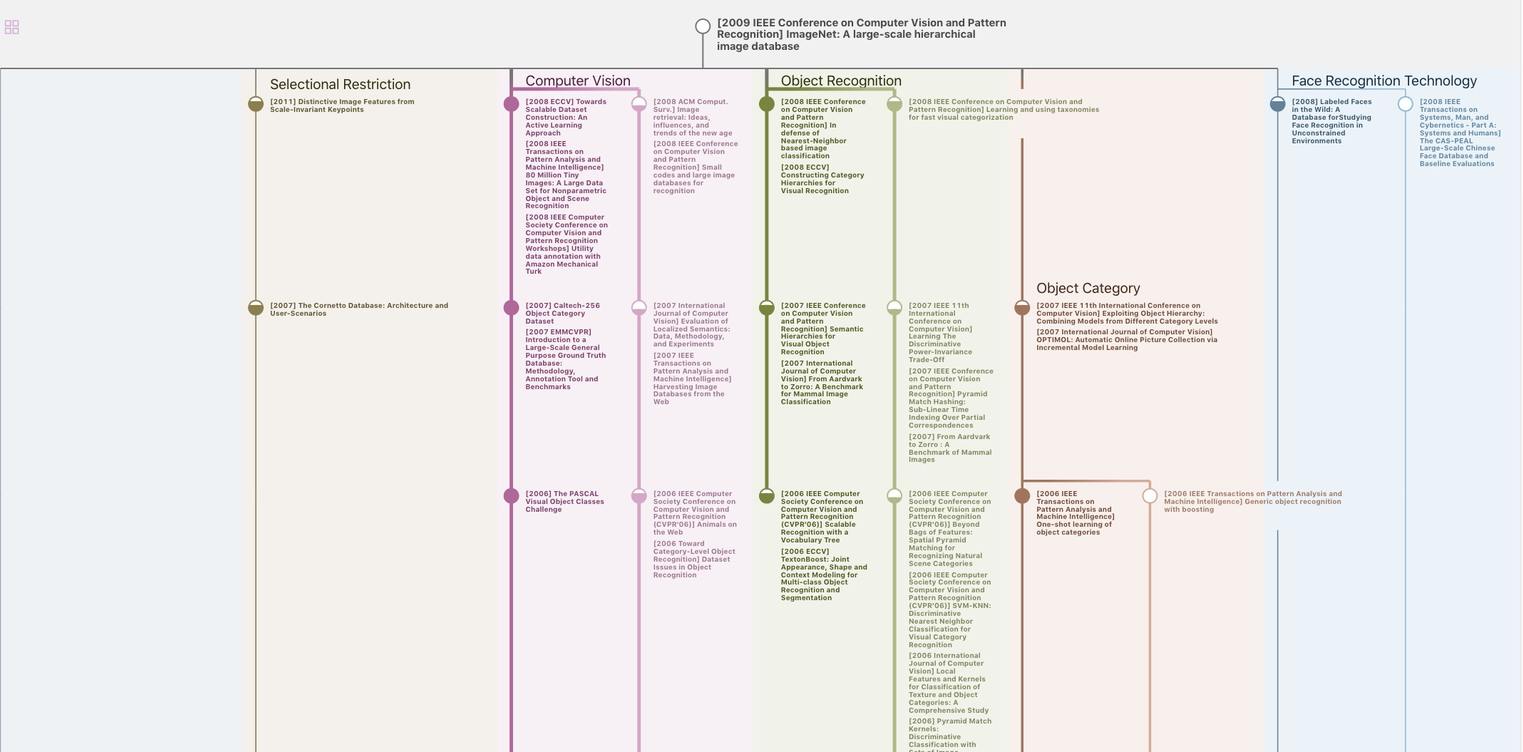
生成溯源树,研究论文发展脉络
数据免责声明
页面数据均来自互联网公开来源、合作出版商和通过AI技术自动分析结果,我们不对页面数据的有效性、准确性、正确性、可靠性、完整性和及时性做出任何承诺和保证。若有疑问,可以通过电子邮件方式联系我们:report@aminer.cn