VTQAGen: BART-based Generative Model for Visual Text Question Answering
PROCEEDINGS OF THE 31ST ACM INTERNATIONAL CONFERENCE ON MULTIMEDIA, MM 2023(2023)
摘要
Visual Text Question Answering (VTQA) is a challenging task that requires answering questions pertaining to visual content by combining image understanding and language comprehension. The main objective is to develop models that can accurately provide relevant answers based on complementary information from both images and text, as well as the semantic meaning of the question. Despite ongoing efforts, the VTQA task presents several challenges, including multimedia alignment, multi-step cross-media reasoning, and handling open-ended questions. This paper introduces a novel generative framework called VTQAGen, which leverages a Multi- modal Attention Layer to combine image-text pairs and question inputs, as well as a BART-based model for reasoning and entity extraction from both images and text. The framework incorporates a step-based ensemble method to enhance model performance and generalization ability. VTQAGen utilizes an encoder-decoder generative model based on BART. Faster R-CNN is employed to extract visual regions of interest, while BART's encoder is modified to handle multi-modal interaction. The decoder stage utilizes the shift-predict approach and introduces step-based logits fusion to improve stability and accuracy. In the experiments, the proposed VTQAGen demonstrates superior performance on the testing set, securing second place in the ACM Multimedia Visual Text Question Answer Challenge.
更多查看译文
关键词
Visual Text Question Answering,Multi-modal Attention,Crossmedia Reasoning
AI 理解论文
溯源树
样例
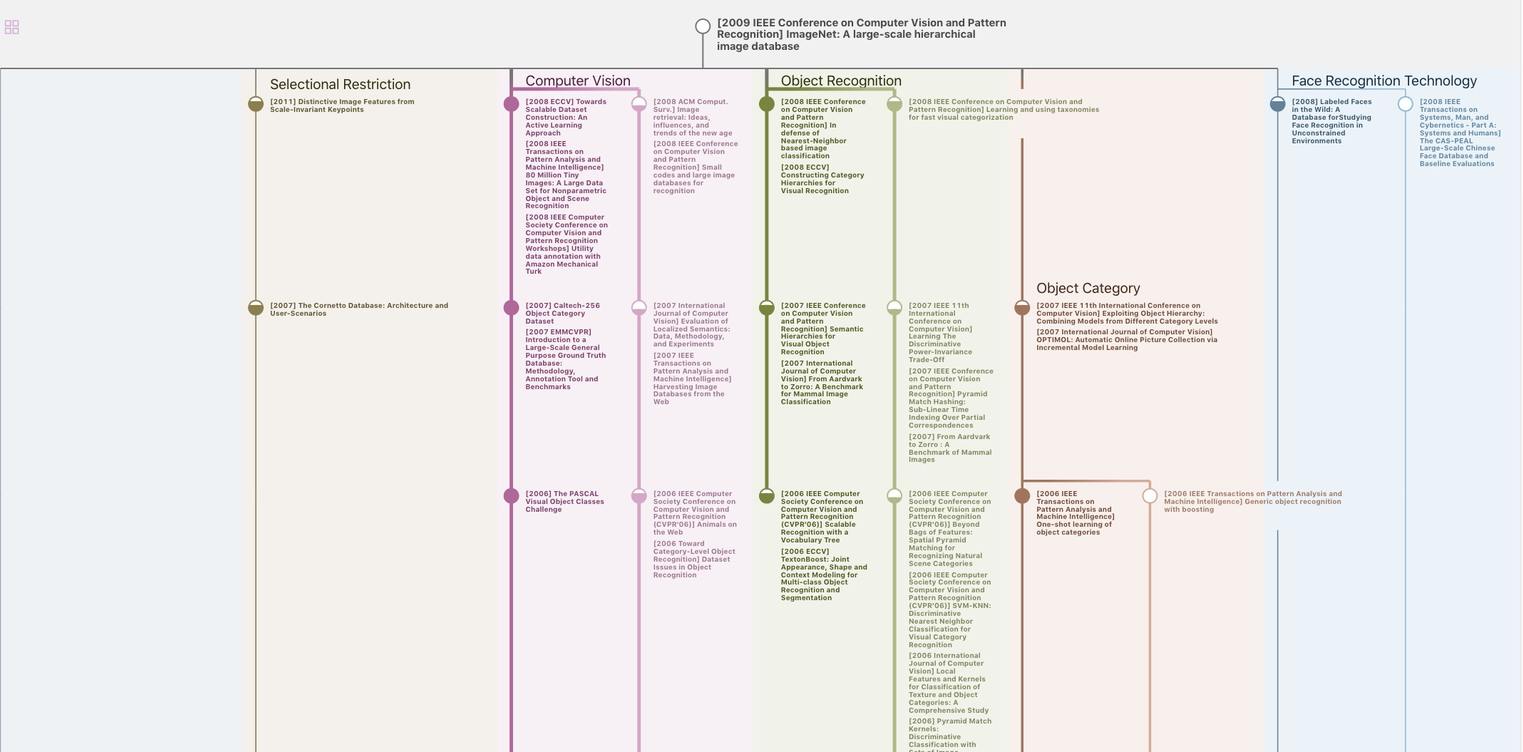
生成溯源树,研究论文发展脉络
数据免责声明
页面数据均来自互联网公开来源、合作出版商和通过AI技术自动分析结果,我们不对页面数据的有效性、准确性、正确性、可靠性、完整性和及时性做出任何承诺和保证。若有疑问,可以通过电子邮件方式联系我们:report@aminer.cn