On the Relevance of Explanation for RDF Resources Similarity.
MOBA(2023)
摘要
Artificial Intelligence (AI) has been shown to productively affect organizational decision making, in terms of returned economic value. In particular, agile business may significantly benefit from the ability of AI systems to constantly pursue contextual knowledge awareness. Undoubtedly, a key added value of such systems is the ability to explain results. In fact, users are more inclined to trust and feel the accountability of systems, when the output is returned together with a human-readable explanation. Nevertheless, some of the information in an explanation might be irrelevant to users—despite its truthfulness. This paper discusses the relevance of explanation for resources similarity, provided by AI systems. In particular, the analysis focuses on one system based on Large Language Models (LLMs)—namely ChatGPT— and on one logic-based tool relying on the computation of the Least Common Subsumer in the Resource Description Framework (RDF). This discussion reveals the need for a formal distinction between relevant and irrelevant information, that we try to answer with a definition of relevance amenable to implementation.
更多查看译文
关键词
similarity,resources,relevance
AI 理解论文
溯源树
样例
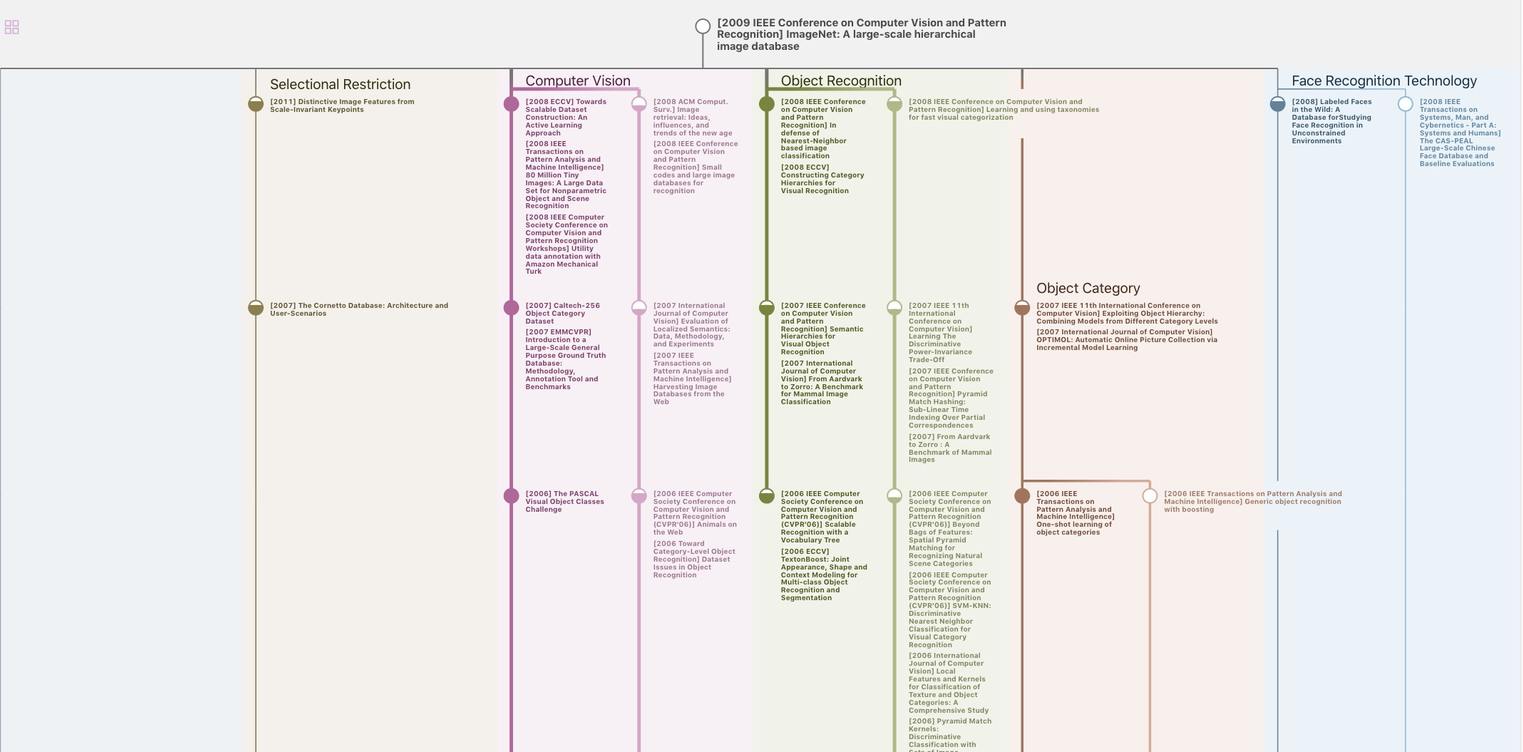
生成溯源树,研究论文发展脉络
数据免责声明
页面数据均来自互联网公开来源、合作出版商和通过AI技术自动分析结果,我们不对页面数据的有效性、准确性、正确性、可靠性、完整性和及时性做出任何承诺和保证。若有疑问,可以通过电子邮件方式联系我们:report@aminer.cn