Deep Learning Driven Multifeature Extraction for Quality Evaluation of Ultrafast Laser Drilled Microhole Arrays
JOURNAL OF LASER APPLICATIONS(2023)
摘要
An efficient quality evaluation method is crucial for the applications of high-quality microhole arrays drilled with ultrafast lasers. The vision-based feature extraction was used as a data acquisition method to evaluate the drilling quality in terms of the geometric quality of the hole shape. However, the morphological features such as the recast layer, microcracks, and debris on the surface are difficult to consider in the quality evaluation since simultaneous recognition of multiple features remains challenging. Herein, we successfully recognized and extracted multiple features by deep learning, thus achieving the quality evaluation of microhole arrays in terms of both geometrical and surface qualities. Microhole arrays of various sizes and surface quality are fabricated on copper, stainless steel, titanium, and glass using different processing parameters. Then, the images of the microhole arrays are prepared as the dataset to train the deep learning network by labeling the typical features of microholes. The well-trained deep learning network has efficient and powerful recognition ability. Typical features such as the hole profile, recast layer, microcracks, and debris can be recognized and extracted simultaneously; thereby the geometric and surface quality of the microhole are obtained. We also demonstrate the implementation of the method with a fast quality evaluation of an array of 2300 microholes based on a statistical approach. The methods presented here extend the quality evaluation of microhole arrays by considering both geometric and surface qualities and can also be applied to quality monitoring in other ultrafast laser micromachining.
更多查看译文
关键词
ultrafast laser,laser drilling,microhole arrays,feature extraction,deep learning,quality evaluation
AI 理解论文
溯源树
样例
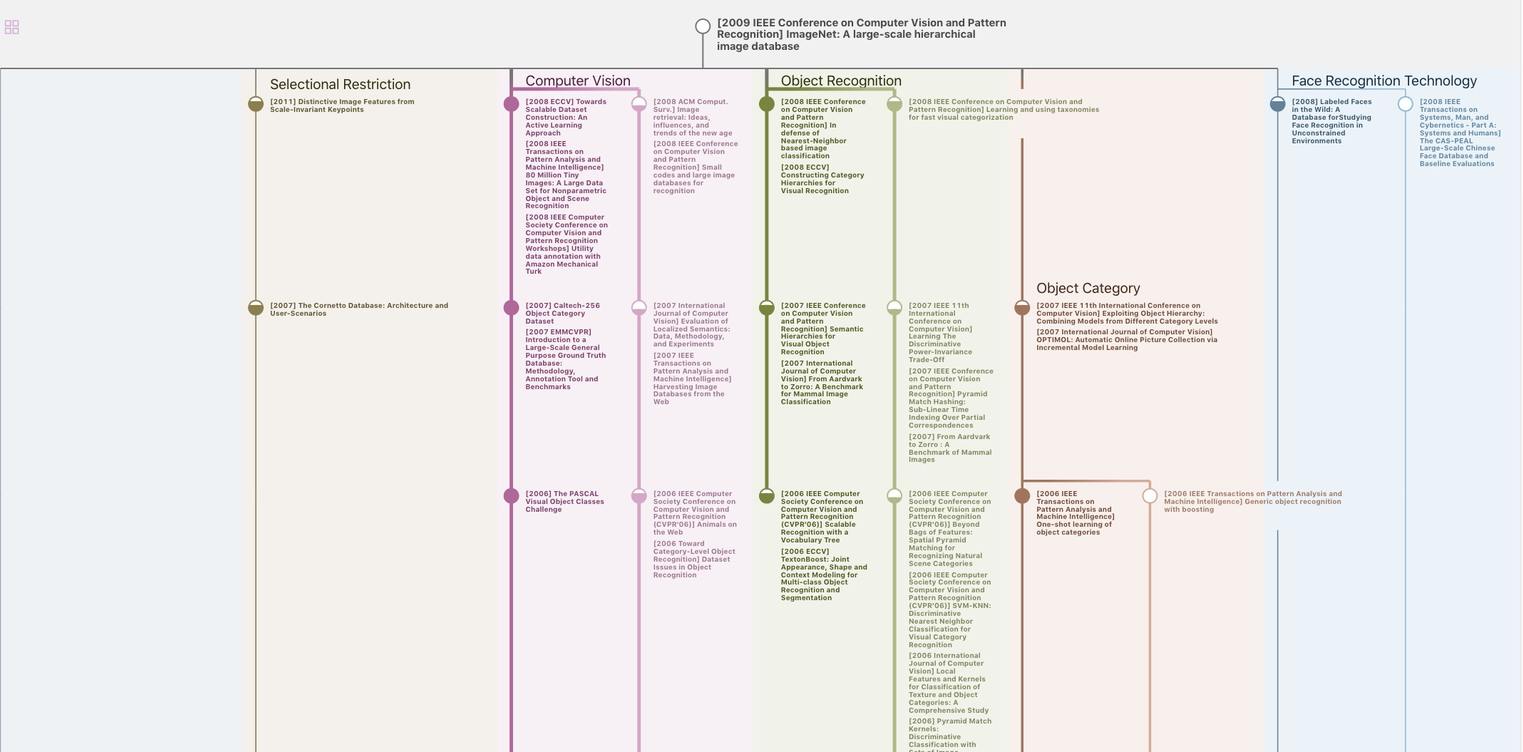
生成溯源树,研究论文发展脉络
数据免责声明
页面数据均来自互联网公开来源、合作出版商和通过AI技术自动分析结果,我们不对页面数据的有效性、准确性、正确性、可靠性、完整性和及时性做出任何承诺和保证。若有疑问,可以通过电子邮件方式联系我们:report@aminer.cn