Testing Learning-Enabled Cyber-Physical Systems with Large-Language Models: A Formal Approach
COMPANION PROCEEDINGS OF THE 32ND ACM INTERNATIONAL CONFERENCE ON THE FOUNDATIONS OF SOFTWARE ENGINEERING, FSE COMPANION 2024(2024)
摘要
The integration of machine learning (ML) into cyber-physical systems (CPS)offers significant benefits, including enhanced efficiency, predictivecapabilities, real-time responsiveness, and the enabling of autonomousoperations. This convergence has accelerated the development and deployment ofa range of real-world applications, such as autonomous vehicles, deliverydrones, service robots, and telemedicine procedures. However, the softwaredevelopment life cycle (SDLC) for AI-infused CPS diverges significantly fromtraditional approaches, featuring data and learning as two critical components.Existing verification and validation techniques are often inadequate for thesenew paradigms. In this study, we pinpoint the main challenges in ensuringformal safety for learningenabled CPS.We begin by examining testing as the mostpragmatic method for verification and validation, summarizing the currentstate-of-the-art methodologies. Recognizing the limitations in current testingapproaches to provide formal safety guarantees, we propose a roadmap totransition from foundational probabilistic testing to a more rigorous approachcapable of delivering formal assurance.
更多查看译文
关键词
AI-based Systems,LLM-based Testing,automata-learning,model-based testing
AI 理解论文
溯源树
样例
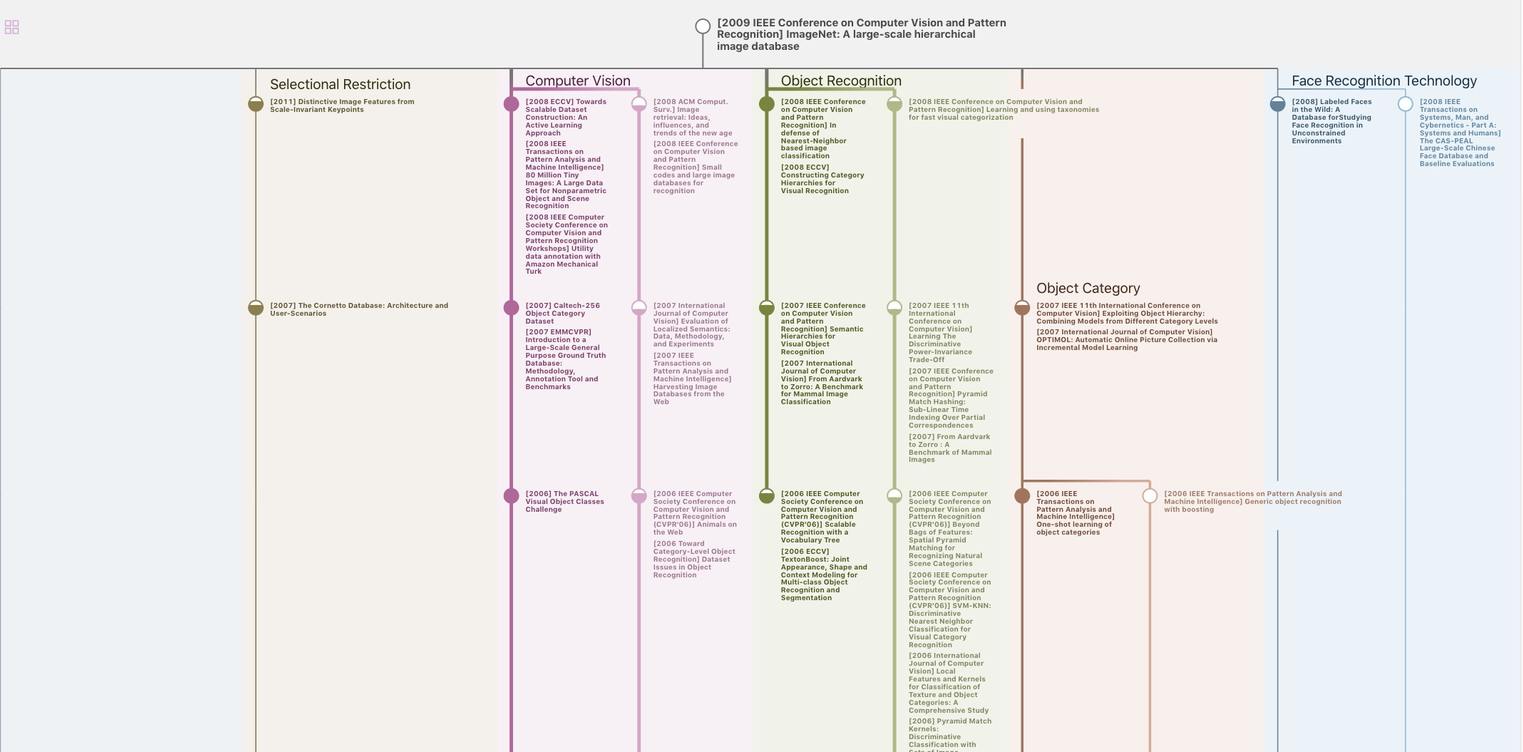
生成溯源树,研究论文发展脉络
数据免责声明
页面数据均来自互联网公开来源、合作出版商和通过AI技术自动分析结果,我们不对页面数据的有效性、准确性、正确性、可靠性、完整性和及时性做出任何承诺和保证。若有疑问,可以通过电子邮件方式联系我们:report@aminer.cn