C3F: Constant Collaboration and Communication Framework for Graph-Representation Dynamic Multi-Robotic Systems
IEEE ROBOTICS AND AUTOMATION LETTERS(2024)
摘要
Deep reinforcement learning (DRL) methods have been widely applied in distributed multi-robotic systems and successfully realized autonomous learning in many fields. In these fields, robots need to communicate and collaborate with other robots in real time, and reach agreed cognition for task assignment, which puts high requirements on efficiency and stability. However, robots may often get damaged even crash in complex environments, and have to be dynamically substituted. It seems not robust enough for most existing DRL works to make new robots fast adapt to current team policies, causing performance degradation. In this work, we get inspired by the genetic mechanism of social animals' instincts, and propose a robust multi-robotic collaboration and communication framework, C3F . It introduces graph-based representation to discover more features on the relevance among robots, and takes advantage of meta learning mechanism to conclude the general meta policy. When some robots crash and get replaced by new ones, this meta policy will be reused to guide new robots on how to quickly follow the existing collaboration and communication rules, and fast adapt to their roles in the team. The experiments on both the Webots simulator and the Starcraft II platform indicate that our methods have better performance compared with some SOTA methods, showing strong robustness and remarkable adaptability to the dynamic substitution in multi-robotic systems.
更多查看译文
关键词
Multi-robot systems,reinforcement learning,distributed robot systems
AI 理解论文
溯源树
样例
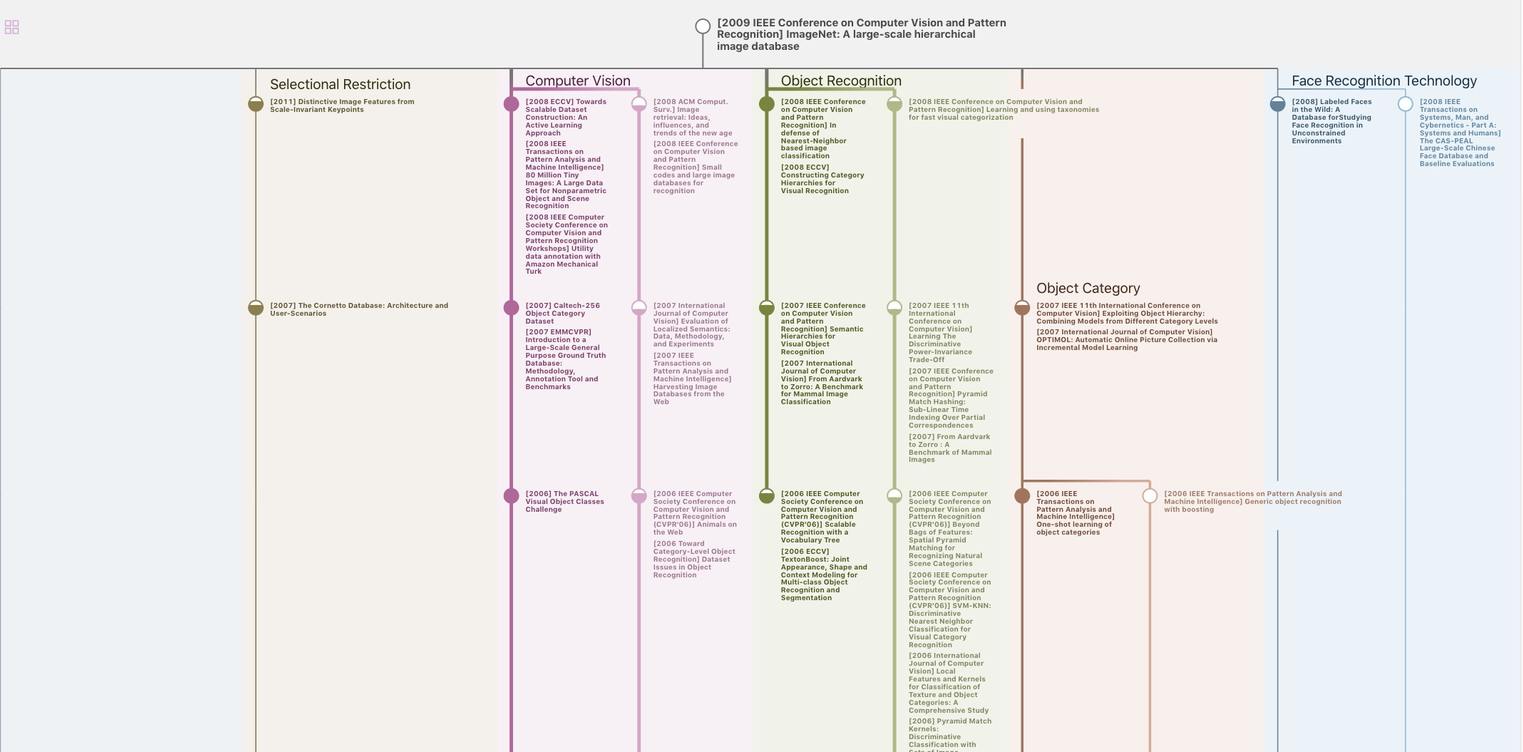
生成溯源树,研究论文发展脉络
数据免责声明
页面数据均来自互联网公开来源、合作出版商和通过AI技术自动分析结果,我们不对页面数据的有效性、准确性、正确性、可靠性、完整性和及时性做出任何承诺和保证。若有疑问,可以通过电子邮件方式联系我们:report@aminer.cn