A Survey of Deep Active Learning for Foundation Models
Intelligent Computing(2023)
摘要
Active learning (AL) is an effective sample selection approach that annotates only a subset of the training data to address the challenge of data annotation, and deep learning (DL) is data-intensive and reliant on abundant training data. Deep active learning (DeepAL) benefits from the integration of AL and DL, offering an efficient solution that balances model performance and annotation costs. The importance of DeepAL has been increasingly recognized with the emergence of large foundation models that depend heavily on substantial computational resources and extensive training data. This survey endeavors to provide a comprehensive overview of DeepAL. Specifically, we first analyze and summarize various sample query strategies, data querying considerations, model training paradigms, and real-world applications of DeepAL. In addition, we discuss the challenges that arise in the era of foundation models and propose potential directions for future AL research. The survey aims to bridge a gap in the existing literature by organizing and summarizing current approaches, offering insights into DeepAL and highlighting the necessity of developing specialized DeepAL techniques tailored to foundation models. By critically examining the current state of DeepAL, this survey contributes to a more profound understanding of the field and serves as a guide for researchers and practitioners interested in DeepAL techniques.
更多查看译文
AI 理解论文
溯源树
样例
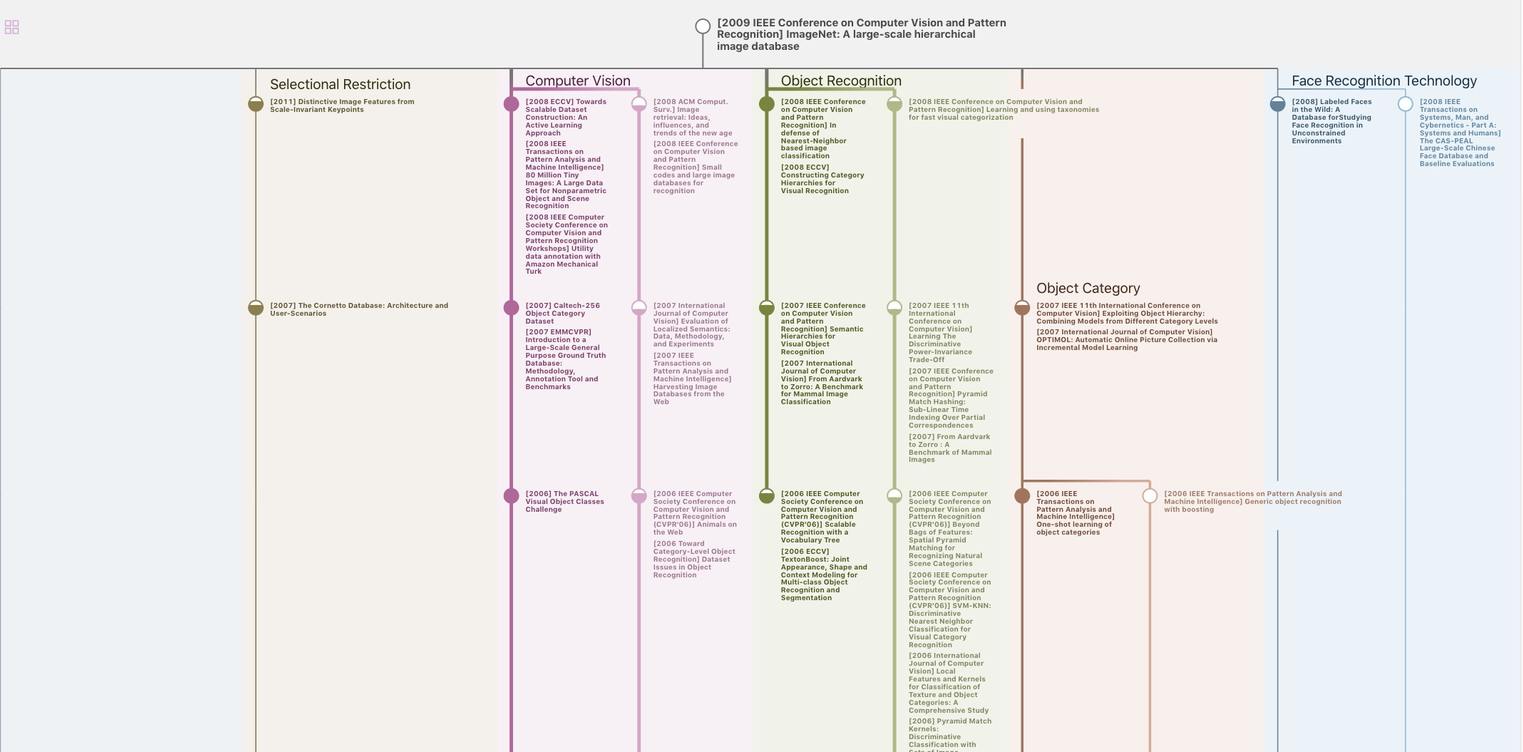
生成溯源树,研究论文发展脉络
数据免责声明
页面数据均来自互联网公开来源、合作出版商和通过AI技术自动分析结果,我们不对页面数据的有效性、准确性、正确性、可靠性、完整性和及时性做出任何承诺和保证。若有疑问,可以通过电子邮件方式联系我们:report@aminer.cn