Synthesize, Diagnose, and Optimize: Towards Fine-Grained Vision-Language Understanding
Computer Vision and Pattern Recognition(2024)
摘要
Vision language models (VLM) have demonstrated remarkable performance acrossvarious downstream tasks. However, understanding fine-grained visual-linguisticconcepts, such as attributes and inter-object relationships, remains asignificant challenge. While several benchmarks aim to evaluate VLMs in finergranularity, their primary focus remains on the linguistic aspect, neglectingthe visual dimension. Here, we highlight the importance of evaluating VLMs fromboth a textual and visual perspective. We introduce a progressive pipeline tosynthesize images that vary in a specific attribute while ensuring consistencyin all other aspects. Utilizing this data engine, we carefully design abenchmark, SPEC, to diagnose the comprehension of object size, position,existence, and count. Subsequently, we conduct a thorough evaluation of fourleading VLMs on SPEC. Surprisingly, their performance is close to random guess,revealing significant limitations. With this in mind, we propose a simple yeteffective approach to optimize VLMs in fine-grained understanding, achievingsignificant improvements on SPEC without compromising the zero-shotperformance. Results on two additional fine-grained benchmarks also showconsistent improvements, further validating the transferability of ourapproach. Code and data are available at https://github.com/wjpoom/SPEC.
更多查看译文
关键词
Vision language model,Fine-grained understdanding
AI 理解论文
溯源树
样例
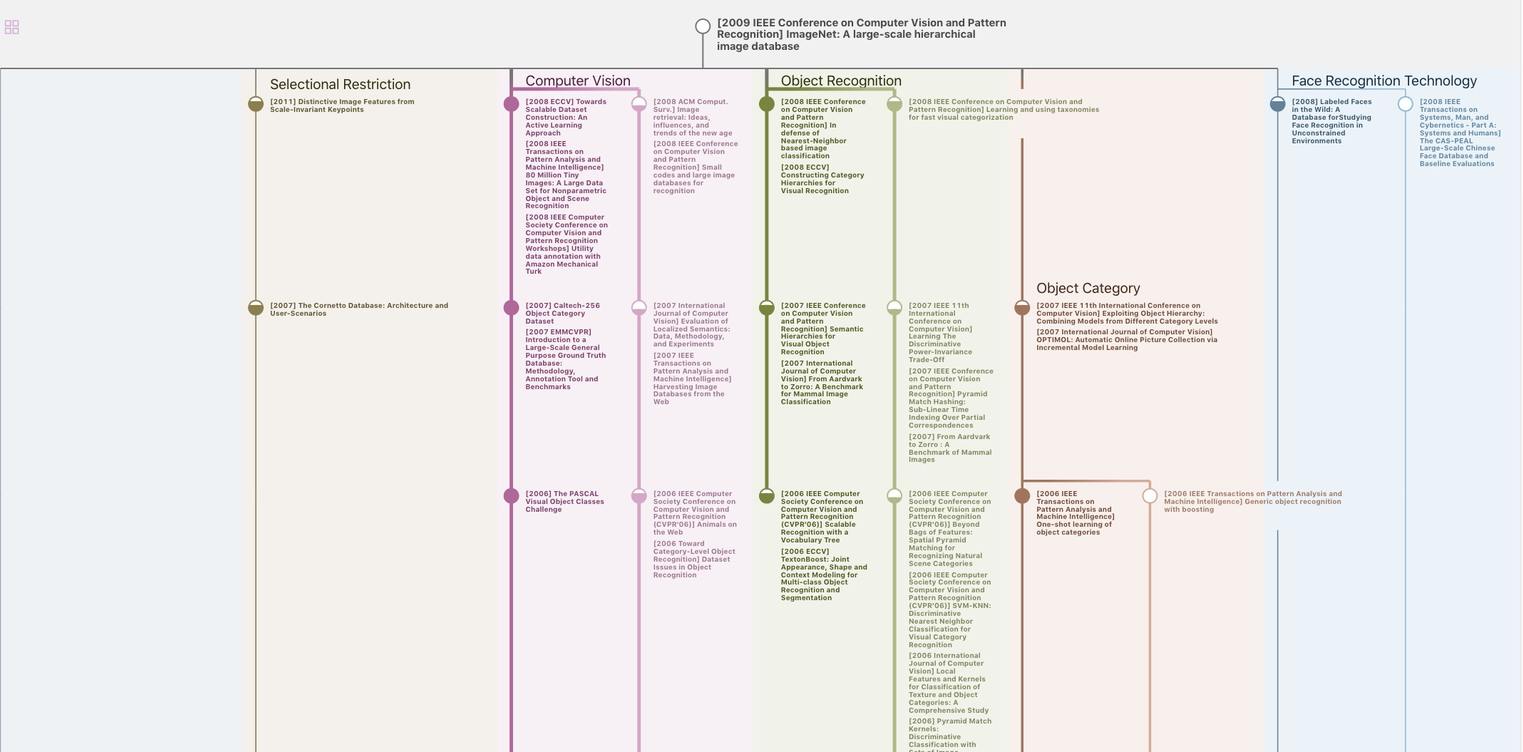
生成溯源树,研究论文发展脉络
数据免责声明
页面数据均来自互联网公开来源、合作出版商和通过AI技术自动分析结果,我们不对页面数据的有效性、准确性、正确性、可靠性、完整性和及时性做出任何承诺和保证。若有疑问,可以通过电子邮件方式联系我们:report@aminer.cn