Sim-to-Real Transfer for Estimation over Wireless Networks
openalex(2023)
摘要
We investigate a simulation to real-world transfer of data-driven models for state estimation using measurements received over a wireless network. Real-world networks, such as vehicular communication systems, face issues like channel impairments and network congestion, and hence estimates are computed using a history of measurements that are received intermittently and are aged on reception. Training an estimator model directly with real-world data is expensive and impractical due to diverse network conditions. To address this, we present an approach that doesn’t rely on real-world network data. We train a model using only data generated by a low-cost low-fidelity simulation of networks. To bridge the gap between simulation and reality, we employ domain randomization, appropriately randomizing simulation parameters during training. An in depth investigation of network simulation is provided that has a FCFS queue with two parameters, the rate of arrival of packets into the queue and packet service rates. The model is validated by two real network deployments. In one, we process vehicular trajectory from up to 70 nodes over WiFi to estimate vehicle positions and speeds. In the other, GPS coordinates are communicated by public transit buses over city-wide cellular networks to a transport authority database. This information is obtained by a server running in the AWS cloud, by polling at a certain fixed rate. The model uses the polled data to generate estimates of bus positions. Our proposed estimator model is a novel deep neural network (DNN) architecture, which outperforms existing approaches to data-driven estimation.
更多查看译文
关键词
Wireless Networks,Social Sensing,Real-time Status Updates,Short-Term Forecasting
AI 理解论文
溯源树
样例
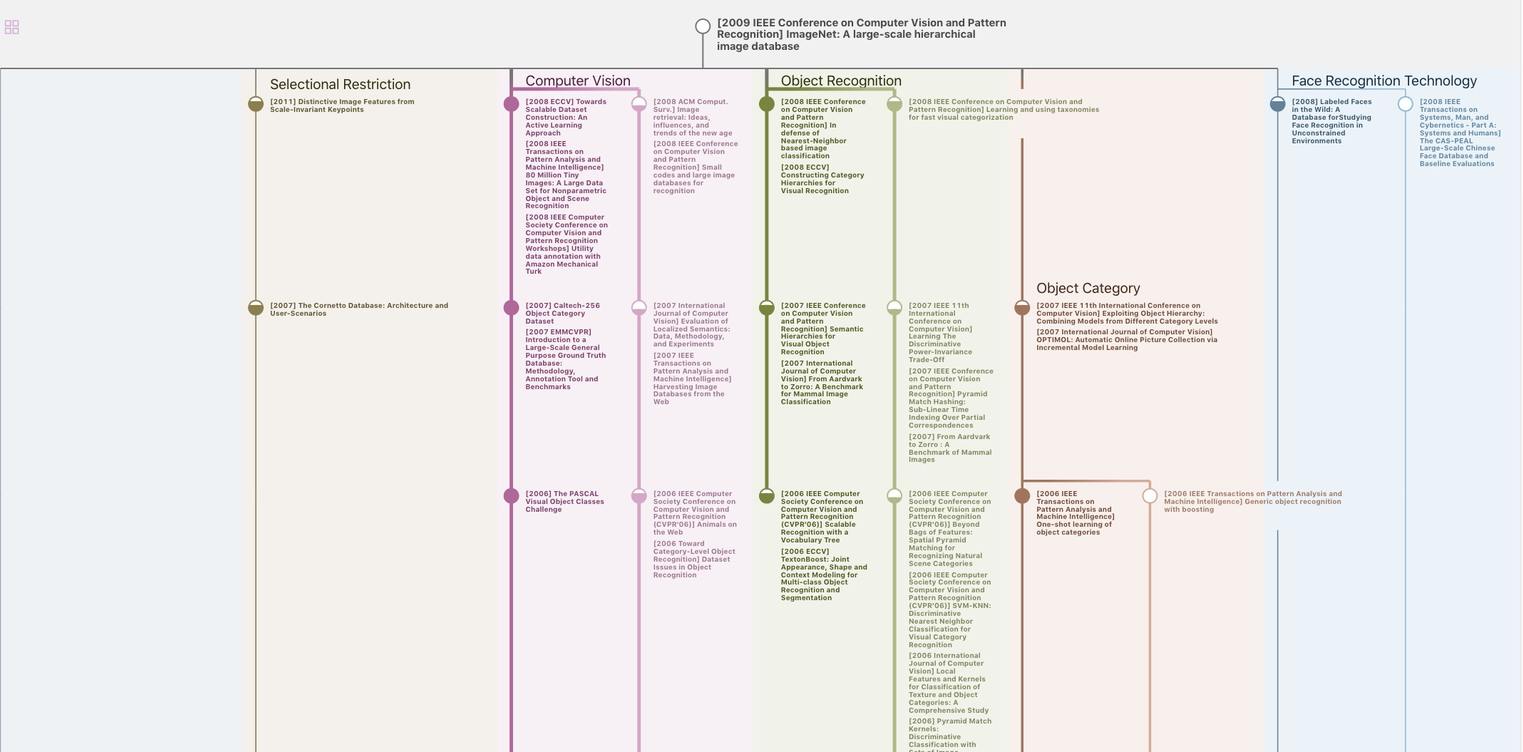
生成溯源树,研究论文发展脉络
数据免责声明
页面数据均来自互联网公开来源、合作出版商和通过AI技术自动分析结果,我们不对页面数据的有效性、准确性、正确性、可靠性、完整性和及时性做出任何承诺和保证。若有疑问,可以通过电子邮件方式联系我们:report@aminer.cn